Link Prediction and Unlink Prediction on Dynamic Networks
IEEE Trans Comput Soc Syst(2023)
摘要
Link prediction on dynamic networks has been extensively studied and widely applied in various applications. However, existing methods only consider either the network structure or the temporal information, ignoring the potentialities of using both types of information together to comprehend the complex behaviors of dynamic networks. Moreover, temporal unlink prediction, which also plays an important role in the evolution of social networks, has not been paid much attention. Accurately predicting the links and unlinks on the future network greatly contributes to the network analysis that uncovers more latent relations between nodes. In this work, we assume that there are two kinds of relations between nodes, namely, long-term relations and short-term relations, and we propose an effective algorithm called LULS for temporal link prediction and unlink prediction based on such relations. Specifically, for each snapshot of a dynamic network, LULS first collects higher order structures as two topological matrices by applying short random walks. Then, LULS initializes and optimizes a global matrix and a sequence of temporary matrices for all the snapshots by using nonnegative matrix factorization (NMF) based on the topological matrices, where the global matrix denotes long-term relations and the temporary matrices represent short-term relations of snapshots. Finally, LULS calculates the similarity matrix of the future snapshot and predicts the links and unlinks for the future network. In addition, we further improve the prediction results by using graph regularization constraints to enhance the global matrix, resulting in that the global matrix contains a wealth of topological information and temporal information. The conducted experiments on real-world networks illustrate that LULS outperforms other baselines for both link prediction and unlink prediction tasks.
更多查看译文
关键词
Dynamic network,link prediction,nonnegative matrix factorization,random walk,unlink prediction
AI 理解论文
溯源树
样例
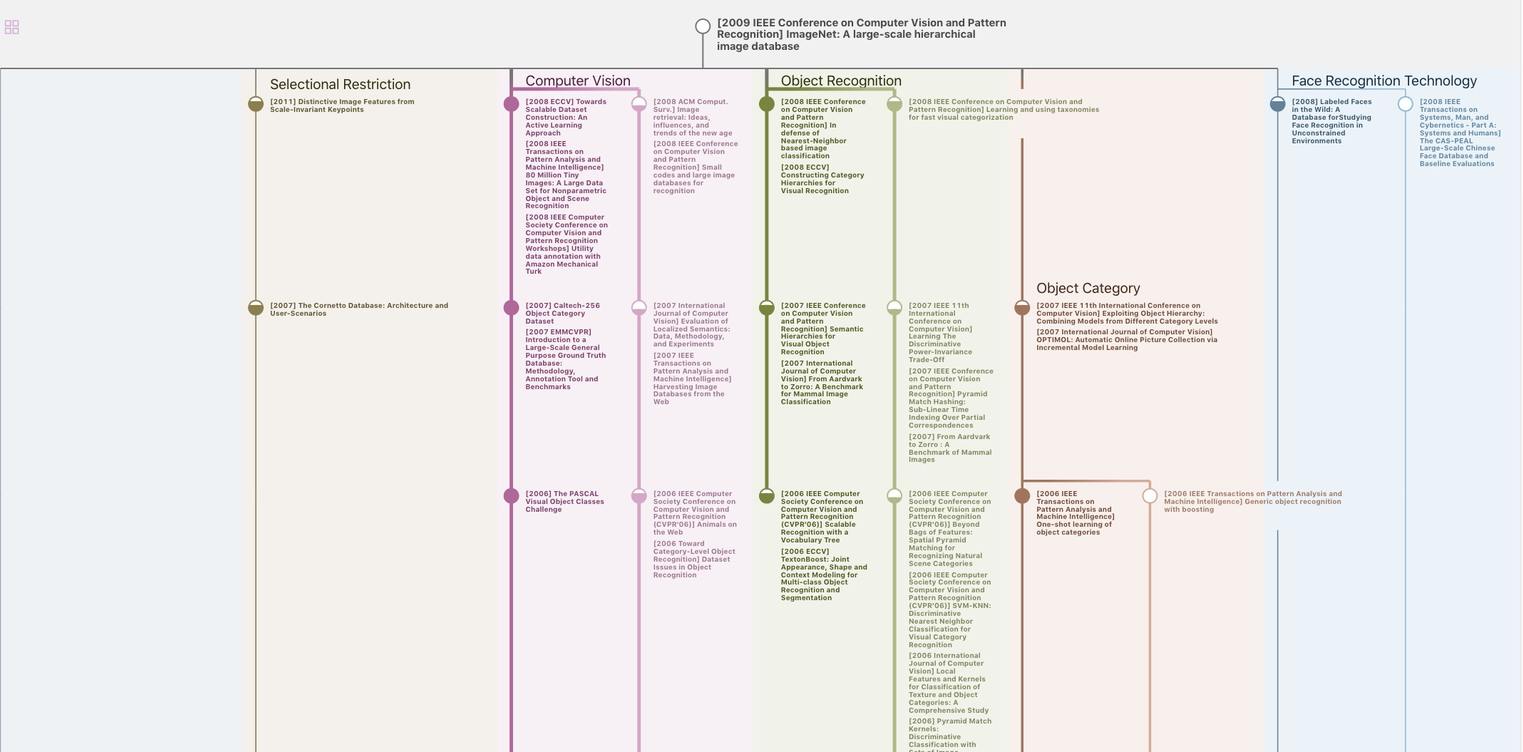
生成溯源树,研究论文发展脉络
Chat Paper
正在生成论文摘要