Data-driven magneto-elastic predictions with scalable classical spin-lattice dynamics
NPJ COMPUTATIONAL MATERIALS(2021)
摘要
A data-driven framework is presented for building magneto-elastic machine-learning interatomic potentials (ML-IAPs) for large-scale spin-lattice dynamics simulations. The magneto-elastic ML-IAPs are constructed by coupling a collective atomic spin model with an ML-IAP. Together they represent a potential energy surface from which the mechanical forces on the atoms and the precession dynamics of the atomic spins are computed. Both the atomic spin model and the ML-IAP are parametrized on data from first-principles calculations. We demonstrate the efficacy of our data-driven framework across magneto-structural phase transitions by generating a magneto-elastic ML-IAP for α -iron. The combined potential energy surface yields excellent agreement with first-principles magneto-elastic calculations and quantitative predictions of diverse materials properties including bulk modulus, magnetization, and specific heat across the ferromagnetic–paramagnetic phase transition.
更多查看译文
关键词
dynamics,data-driven,magneto-elastic,spin-lattice
AI 理解论文
溯源树
样例
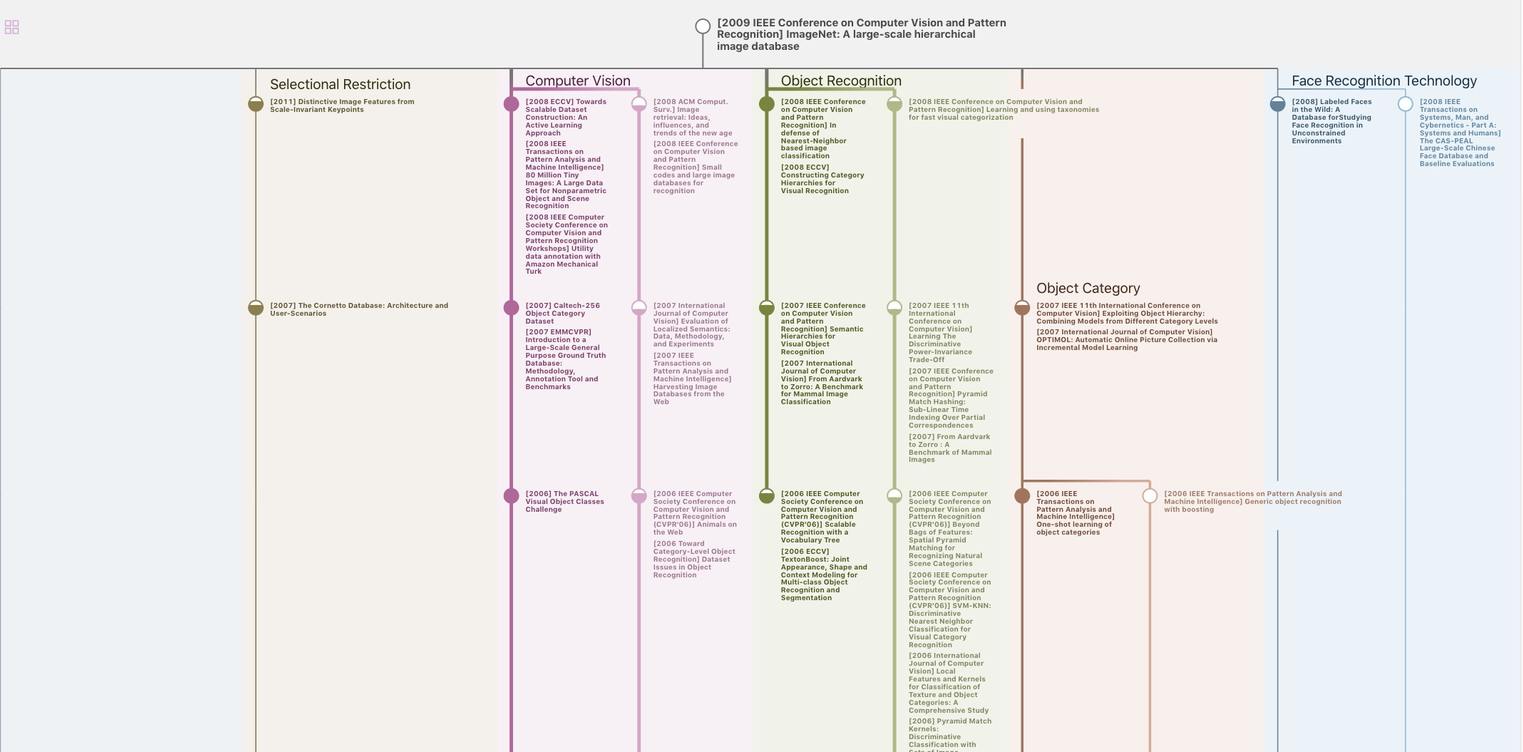
生成溯源树,研究论文发展脉络
Chat Paper
正在生成论文摘要