Prediction and generalisation over directed actions by grid cells
ICLR(2021)
摘要
Knowing how the effects of directed actions generalise to new situations (e.g. moving North, South, East and West, or turning left, right, etc.) is key to rapid generalisation of action to new situations. Markovian tasks can be characterised by a state space and a transition matrix and recent work has proposed that neural grid codes provide an efficient representation of the state space, as eigenvectors of a transition matrix reflecting diffusion across states, that allows efficient prediction of future state distributions. Here we address the ability to predict the effects of directed actions across new tasks, irrespective of differences in local transition structure, to allow fast generalisation and form a \"sense of direction\". We extend the eigenbasis prediction model, utilising tools from Fourier analysis, to prediction over arbitrary translation-invariant directed transition structures (i.e. displacement and diffusion), showing that a single set of eigenvectors can support predictions over arbitrary directed actions via action-specific eigenvalues. We show the equivalence between the generalised prediction framework and traditional models of grid cells firing driven by self-motion to perform path integration using oscillatory interference models (via Fourier components as velocity-controlled oscillators) or continuous attractor networks (via analysis of the update dynamics). We thus provide a unifying framework for the role of the grid system in predictive planning, sense of direction and path integration. The resulting model allows a single grid-like representation to support efficient prediction over directed transitions in spatial and non-spatial tasks, supporting generalisable inference over directed actions across different tasks.
更多查看译文
关键词
grid cells,directed actions,prediction,generalisation
AI 理解论文
溯源树
样例
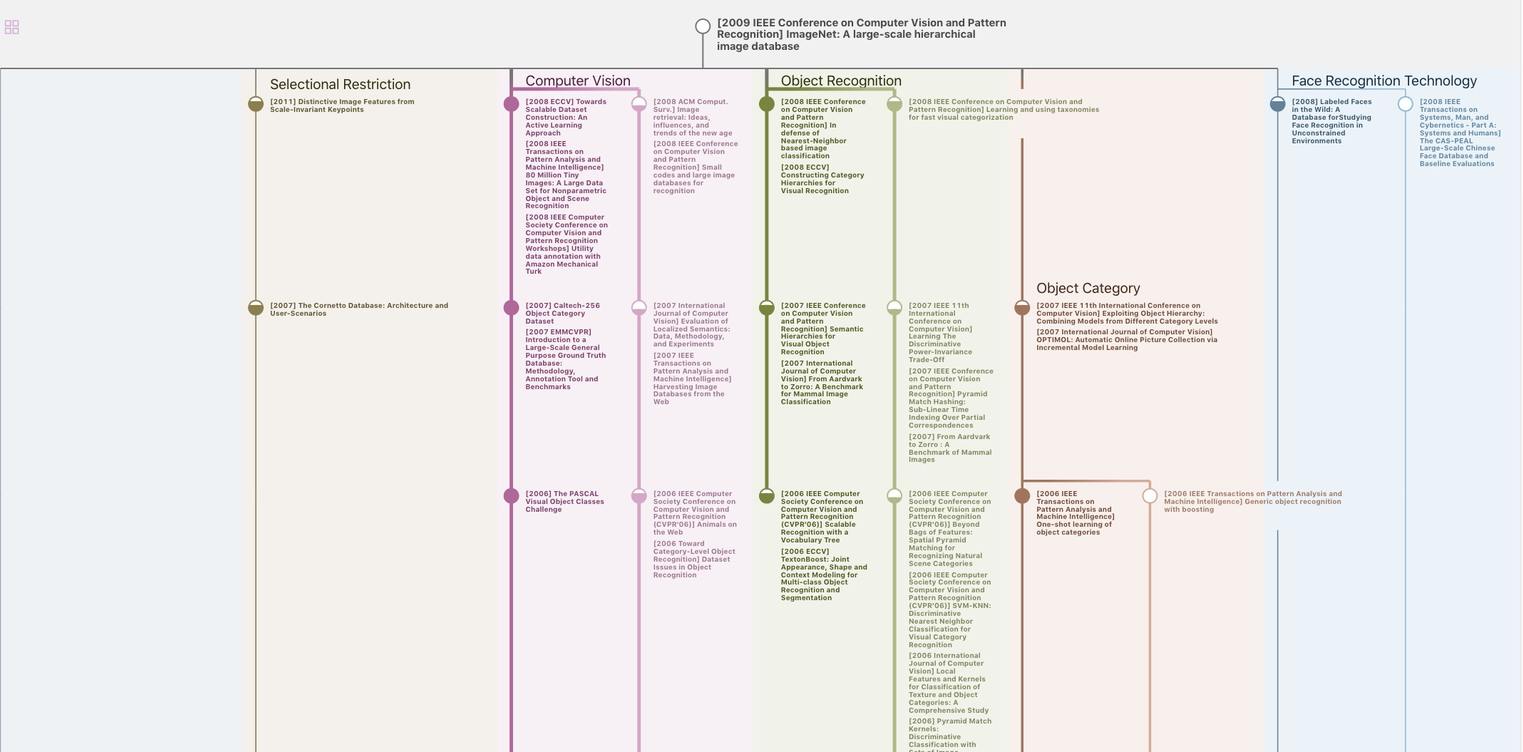
生成溯源树,研究论文发展脉络
Chat Paper
正在生成论文摘要