Knowledge Distillation as Semiparametric Inference
ICLR(2021)
摘要
A popular approach to model compression is to train an inexpensive student model to mimic the class probabilities of a highly accurate but cumbersome teacher model. Surprisingly, this two-step knowledge distillation process often leads to higher accuracy than training the student directly on labeled data. To explain and enhance this phenomenon, we cast knowledge distillation as a semiparametric inference problem with the optimal student model as the target, the unknown Bayes class probabilities as nuisance, and the teacher probabilities as a plug-in nuisance estimate. By adapting modern semiparametric tools, we derive several new guarantees for the prediction error of standard distillation and develop several enhancements with improved guarantees. We validate our findings empirically on both tabular data and image data and observe consistent improvements from our knowledge distillation enhancements.
更多查看译文
关键词
knowledge distillation
AI 理解论文
溯源树
样例
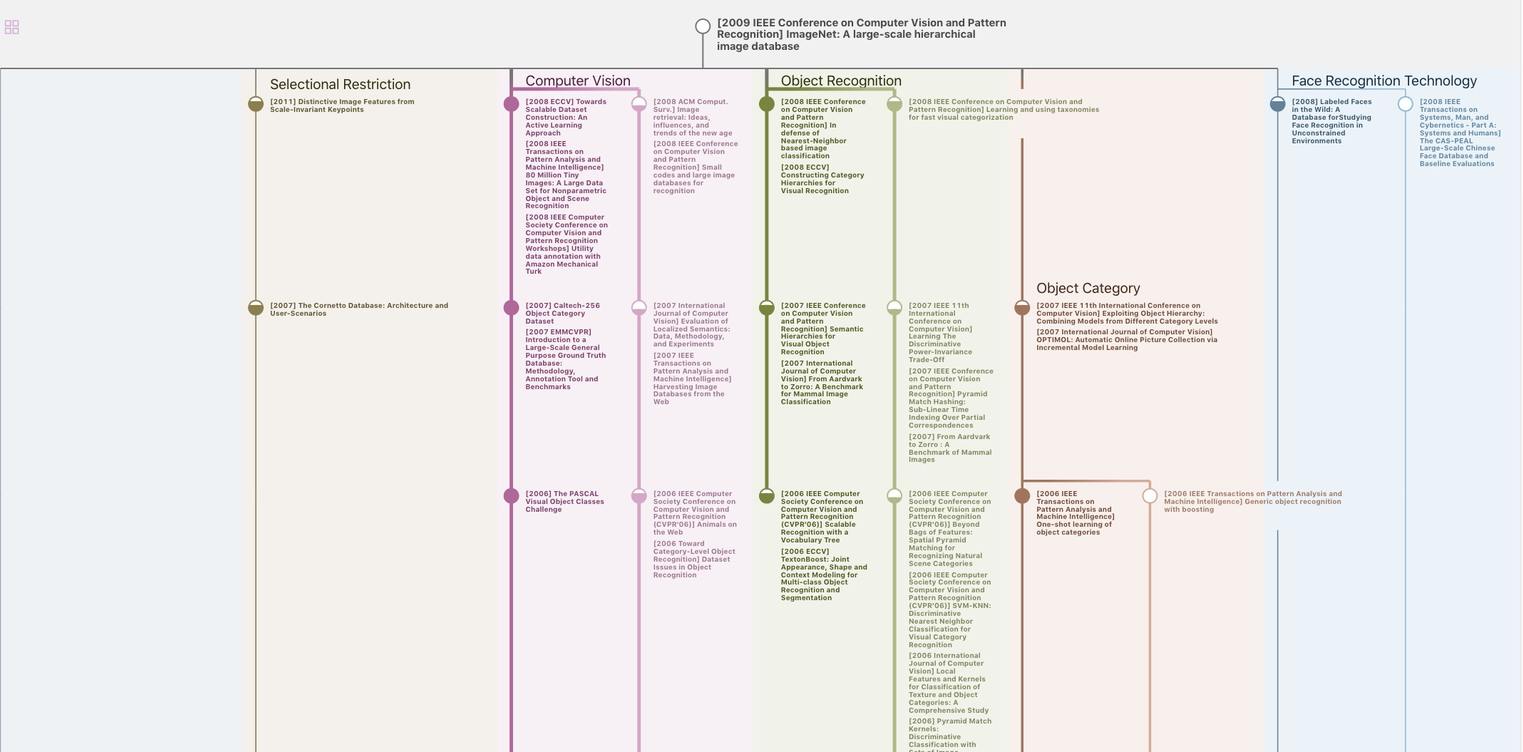
生成溯源树,研究论文发展脉络
Chat Paper
正在生成论文摘要