Go with the Flow: Adaptive Control for Neural ODEs.
International Conference on Learning Representations(2020)
摘要
Despite their elegant formulation and lightweight memory cost, neural ordinary differential equations (NODEs) suffer from known representational limitations. In particular, the single flow learned by NODEs cannot express all homeomorphisms from a given data space to itself, and their static weight parameterization restricts the type of functions they can learn compared to discrete architectures with layer-dependent weights. Here, we describe a new module called neurallycontrolled ODE (N-CODE) designed to improve the expressivity of NODEs. The parameters of N-CODE modules are dynamic variables governed by a trainable map from initial or current activation state, resulting in forms of open-loop and closed-loop control, respectively. A single module is sufficient for learning a distribution on non-autonomous flows that adaptively drive neural representations. We provide theoretical and empirical evidence that N-CODE circumvents limitations of previous NODEs models and show how increased model expressivity manifests in several supervised and unsupervised learning problems. These favorable empirical results indicate the potential of using dataand activity-dependent plasticity in neural networks across numerous domains.
更多查看译文
关键词
adaptive control,flow
AI 理解论文
溯源树
样例
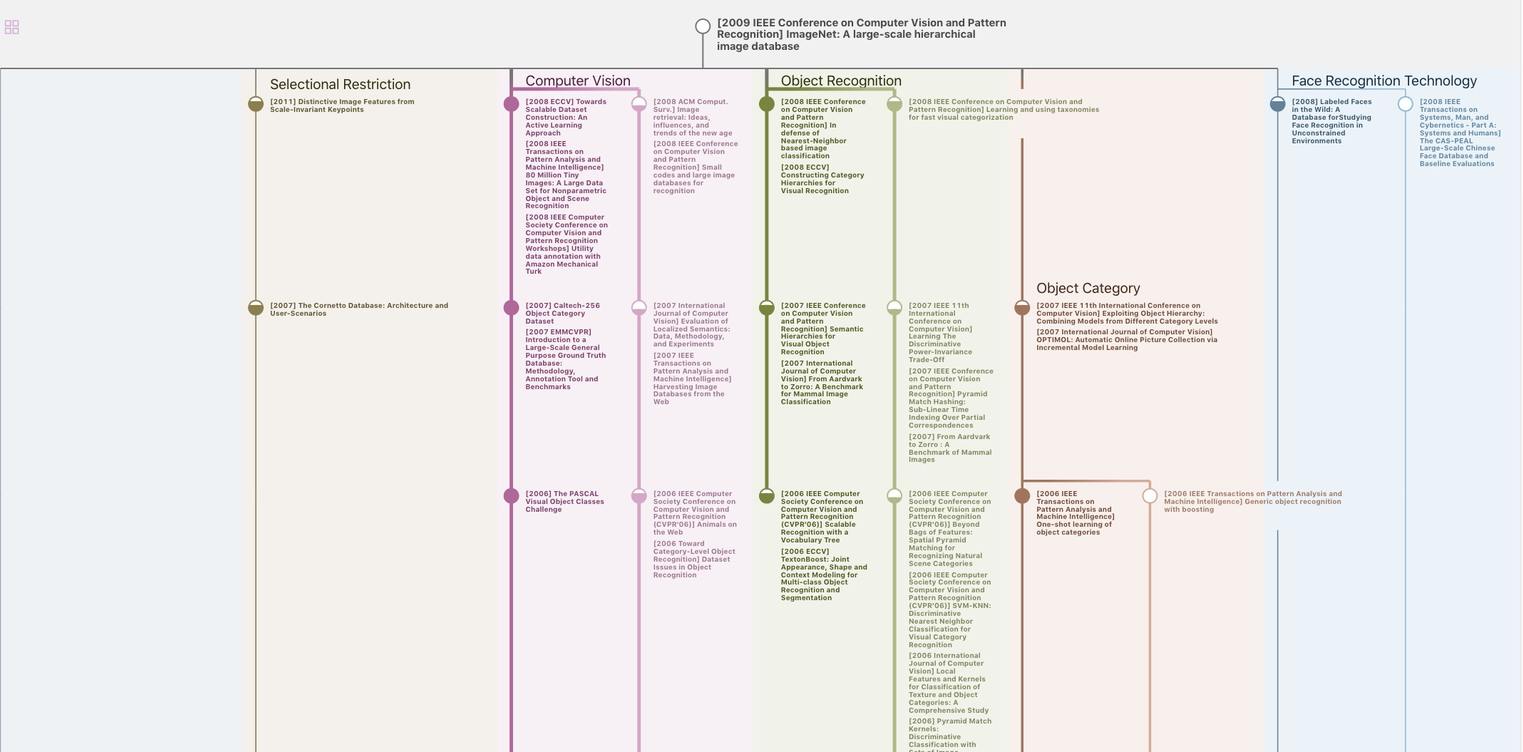
生成溯源树,研究论文发展脉络
Chat Paper
正在生成论文摘要