Navigation And Mapping In Forest Environment Using Sparse Point Clouds
REMOTE SENSING(2020)
摘要
Odometry during forest operations is demanding, involving limited field of vision (FOV), back-and-forth work cycle movements, and occasional close obstacles, which create problems for state-of-the-art systems. We propose a two-phase on-board process, where tree stem registration produces a sparse point cloud (PC) which is then used for simultaneous location and mapping (SLAM). A field test was carried out using a harvester with a laser scanner and a global navigation satellite system (GNSS) performing forest thinning over a 520 m strip route. Two SLAM methods are used: The proposed sparse SLAM (sSLAM) and a standard method, LeGO-LOAM (LLOAM). A generic SLAM post-processing method is presented, which improves the odometric accuracy with a small additional processing cost. The sSLAM method uses only tree stem centers, reducing the allocated memory to approximately 1% of the total PC size. Odometry and mapping comparisons between sSLAM and LLOAM are presented. Both methods show 85% agreement in registration within 15 m of the strip road and odometric accuracy of 0.5 m per 100 m. Accuracy is evaluated by comparing the harvester location derived through odometry to locations collected by a GNSS receiver mounted on the harvester.
更多查看译文
关键词
tree map, SLAM, odometry, LiDAR, 3D object registration, cut-to-length (CTL) harvester, situational awareness
AI 理解论文
溯源树
样例
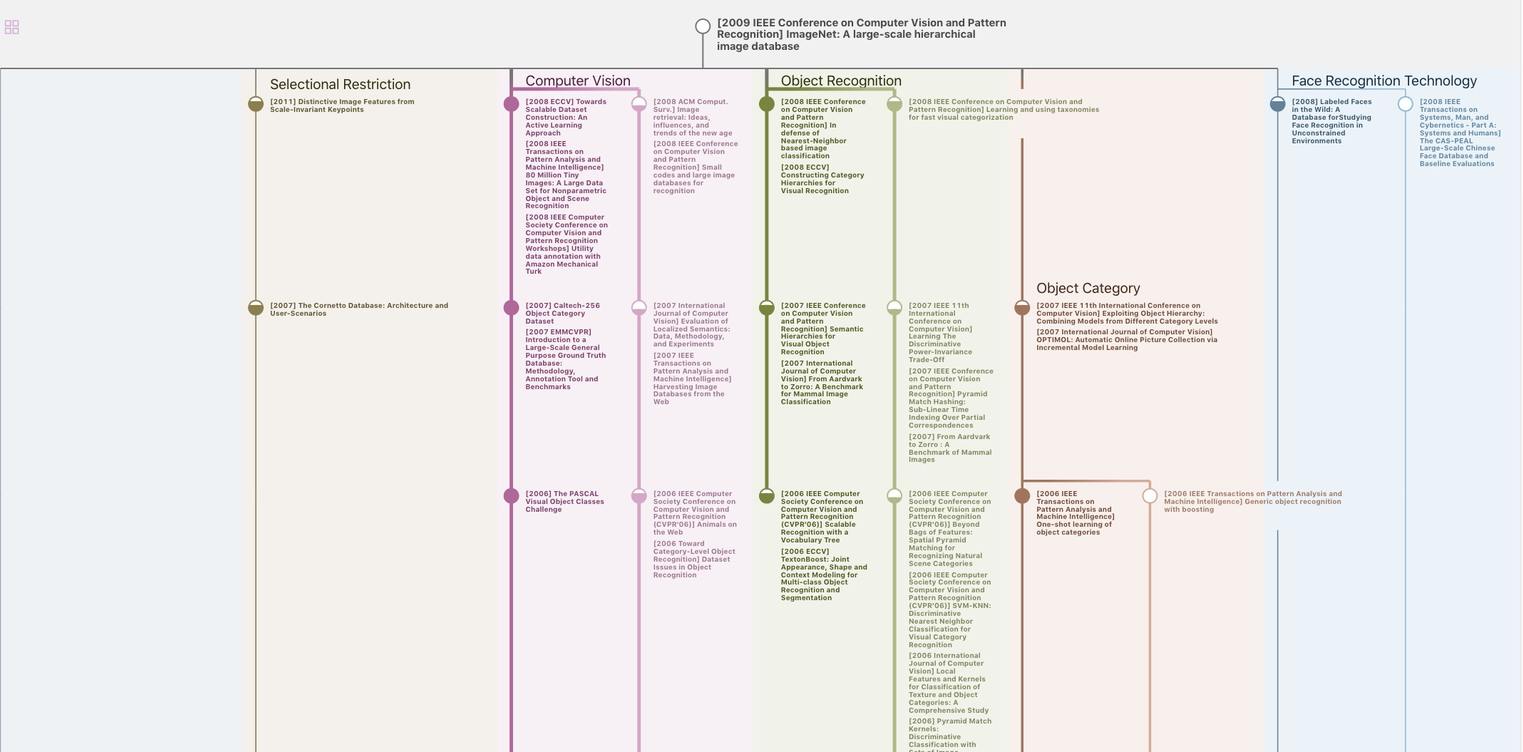
生成溯源树,研究论文发展脉络
Chat Paper
正在生成论文摘要