Evaluating Downscaling Factors of Microwave Satellite Soil Moisture Based on Machine Learning Method.
Remote sensing(2021)
摘要
Downscaling microwave remotely sensed soil moisture (SM) is an effective way to obtain spatial continuous SM with fine resolution for hydrological and agricultural applications on a regional scale. Downscaling factors and functions are two basic components of SM downscaling where the former is particularly important in the era of big data. Based on machine learning method, this study evaluated Land Surface Temperature (LST), Land surface Evaporative Efficiency (LEE), and geographical factors from Moderate Resolution Imaging Spectroradiometer (MODIS) products for downscaling SMAP (Soil Moisture Active and Passive) SM products. This study spans from 2015 to the end of 2018 and locates in the central United States. Original SMAP SM and in-situ SM at sparse networks and core validation sites were used as reference. Experiment results indicated that (1) LEE presented comparative performance with LST as downscaling factors; (2) adding geographical factors can significantly improve the performance of SM downscaling; (3) integrating LST, LEE, and geographical factors got the best performance; (4) using Z-score normalization or hyperbolic-tangent normalization methods did not change the above conclusions, neither did using support vector regression nor feed forward neural network methods. This study demonstrates the possibility of LEE as an alternative of LST for downscaling SM when there is no available LST due to cloud contamination. It also provides experimental evidence for adding geographical factors in the downscaling process.
更多查看译文
关键词
soil moisture,downscaling,SMAP,MODIS,machine learning
AI 理解论文
溯源树
样例
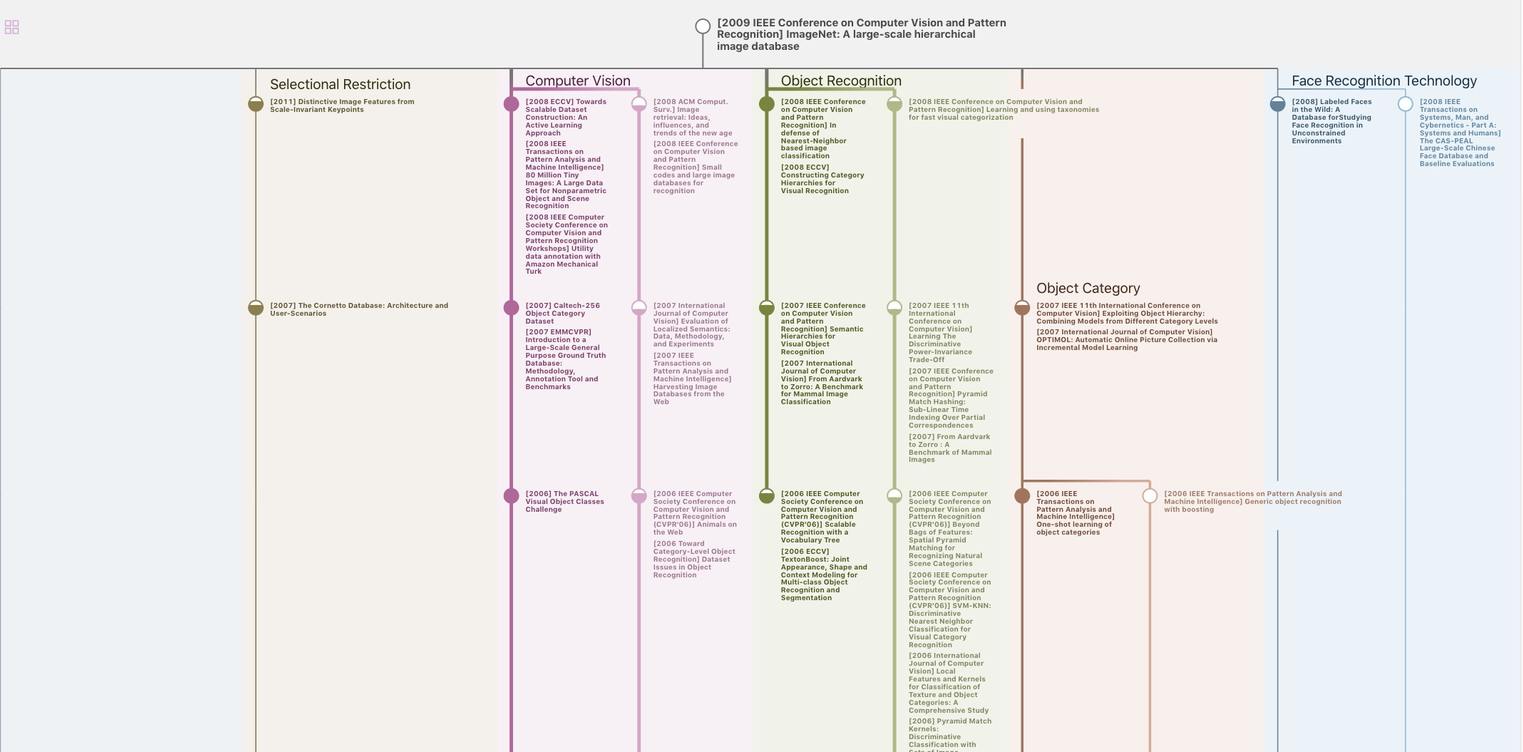
生成溯源树,研究论文发展脉络
Chat Paper
正在生成论文摘要