Leveraging Dynamic Occupancy Grids For 3d Object Detection In Point Clouds
16TH IEEE INTERNATIONAL CONFERENCE ON CONTROL, AUTOMATION, ROBOTICS AND VISION (ICARCV 2020)(2020)
摘要
Traditionally, point cloud-based 3D object detectors are trained on annotated, non-sequential samples taken from driving sequences (e.g. the KITTI dataset). However, by doing this, the developed algorithms renounce to exploit any dynamic information from the driving sequences. It is reasonable to think that this information, which is available at test time when deploying the models in the experimental vehicles, could have significant predictive potential for the object detection task. To study the advantages that this kind of information could provide, we construct a dataset of dynamic occupancy grid maps from the raw KITTI dataset and find the correspondence to each of the KITTI 3D object detection dataset samples. By training a Lidar-based state-of-the-art 3D object detector with and without the dynamic information we get insights into the predictive value of the dynamics. Our results show that having access to the environment dynamics improves by 27% the ability of the detection algorithm to predict the orientation of smaller obstacles such as pedestrians. Furthermore, the 3D and bird's eye view bounding box predictions for pedestrians in challenging cases also see a 7% improvement. Qualitatively speaking, the dynamics help with the detection of partially occluded and far-away obstacles. We illustrate this fact with numerous qualitative prediction results.
更多查看译文
关键词
point cloud-based 3D object detectors,driving sequences,dynamic occupancy grid maps,raw KITTI dataset,KITTI 3D object detection dataset samples,object detection algorithm,lidar-based 3D object detector,smaller obstacles orientation,bird eye view bounding box predictions
AI 理解论文
溯源树
样例
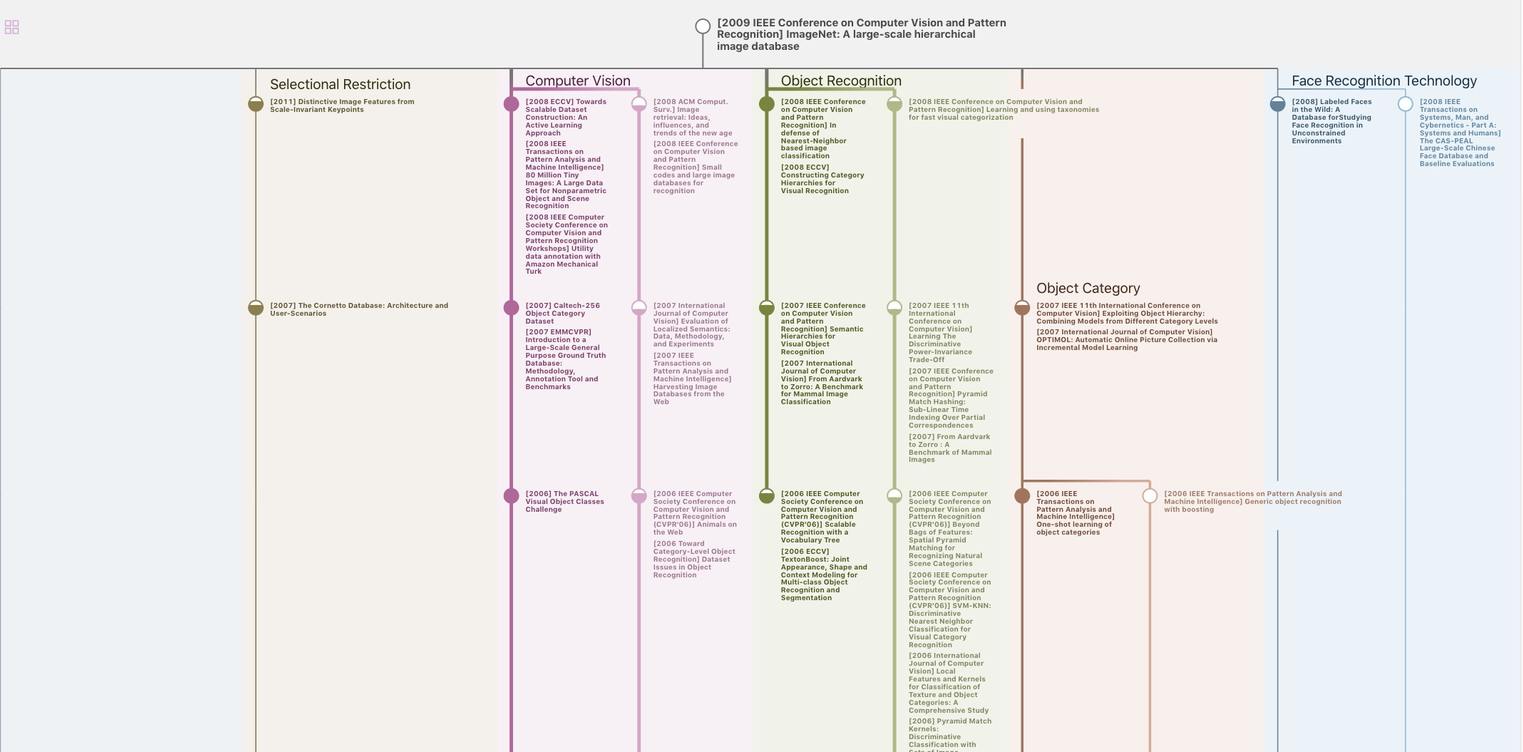
生成溯源树,研究论文发展脉络
Chat Paper
正在生成论文摘要