Autonomous Curriculum Generation For Self-Learning Agents
16TH IEEE INTERNATIONAL CONFERENCE ON CONTROL, AUTOMATION, ROBOTICS AND VISION (ICARCV 2020)(2020)
摘要
The applicability of deep reinforcement learning algorithms to the domain of robotics is limited by the issue of sample inefficiency. As in most machine learning methods, more samples generally mean better learning effectiveness. Sample collection for robotics application is a time-consuming process in addition to safety issues for both the robot itself and the environment surrounding it that come into play for real-world scenarios. Because of these limitations, sample efficiency plays a very vital role in the field of robotic learning. To deal with this, curriculum learning offers a methodology that allows robots to suffer less from the sample collection burden required, trying to keep it at a minimum. This study aims to tackle the sample inefficiency that deep reinforcement learning algorithms face in the domain of robotics by designing a curriculum. We propose an algorithm which decides on the sequence of tasks that the agent must learn to enable the transfer of knowledge in a sample-efficient manner towards the target task. Our algorithm performs a parameter-space task representation for the purpose of deciding on the difficultiness of the tasks. Once the difficulty level of each is determined, easy tasks are learned first before the final target task. We perform a study on a double inverted pendulum setup. Simulation results showed that transfer of knowledge via curriculum is more sample efficient than a direct transfer.
更多查看译文
关键词
robotic learning,curriculum learning,deep reinforcement learning algorithms,sample-efficient manner,parameter-space task representation,autonomous curriculum generation,self-learning agents,machine learning,robotics application,inverted pendulum
AI 理解论文
溯源树
样例
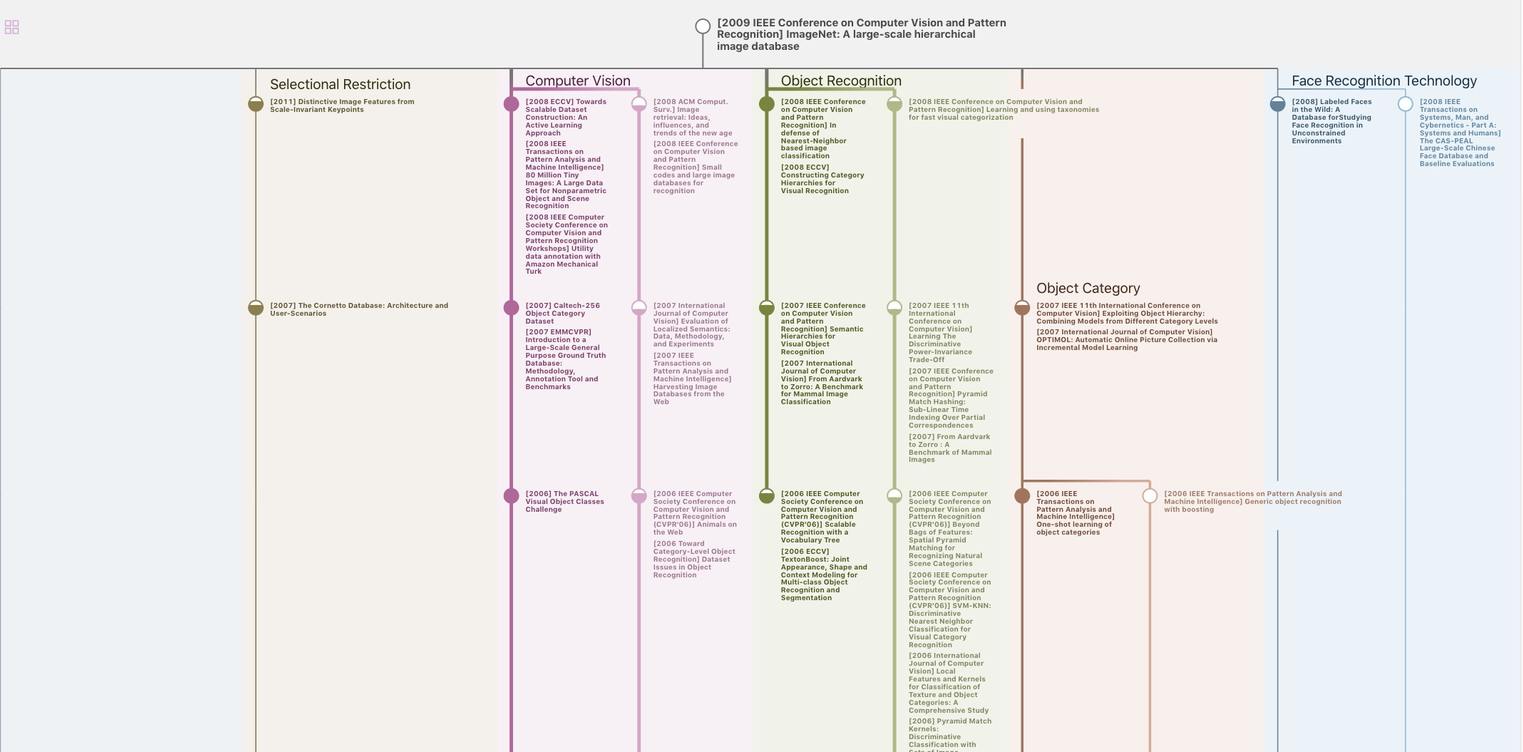
生成溯源树,研究论文发展脉络
Chat Paper
正在生成论文摘要