VAGAN: Vehicle-Aware Generative Adversarial Networks for Vehicle Detection in Rain
2020 16th International Conference on Control, Automation, Robotics and Vision (ICARCV)(2020)
摘要
Vision-based vehicle detection under bad weather conditions is still a challenging problem. Adhesive raindrops on windshield have been known to diffract light and distort parts of the scene behind them. In this paper, we propose a Vehicle-Aware Generative Adversarial Networks (VAGAN) to improve vehicle detection from rain images. We train a Generative Adversarial Network (GAN) on image pairs, each comprising of an original rain image and one that is manually labelled with colored bounding boxes of the vehicles therein. The latter represents a fake version of the original image emphasizing the regions of interest. To further enhance vehicle awareness, we exploit the fact that the vehicle rear lights are usually turned on during rainy conditions to compute a saliency map of image, and use it formulate a background preserving constrain on the learning vehicle loss function. We show that this novel adversarial framework is able to generate new images with colored regions overlay over vehicles, hence effectively learning to differentiate image background from vehicles. The final vehicle detection in the generated images is not affected by image translation noise because we can simply use color segmentation to localize the vehicles. Experimental results on a large dataset show that our approach is an effective way to solve vehicle detection in rain images, achieving state-of-the-art performance.
更多查看译文
关键词
vision-based vehicle detection,rain images,vehicle awareness,vehicle rear lights,image background,image translation noise,VAGAN,vehicle-aware generative adversarial networks,vehicle loss function learning,colored bounding boxes
AI 理解论文
溯源树
样例
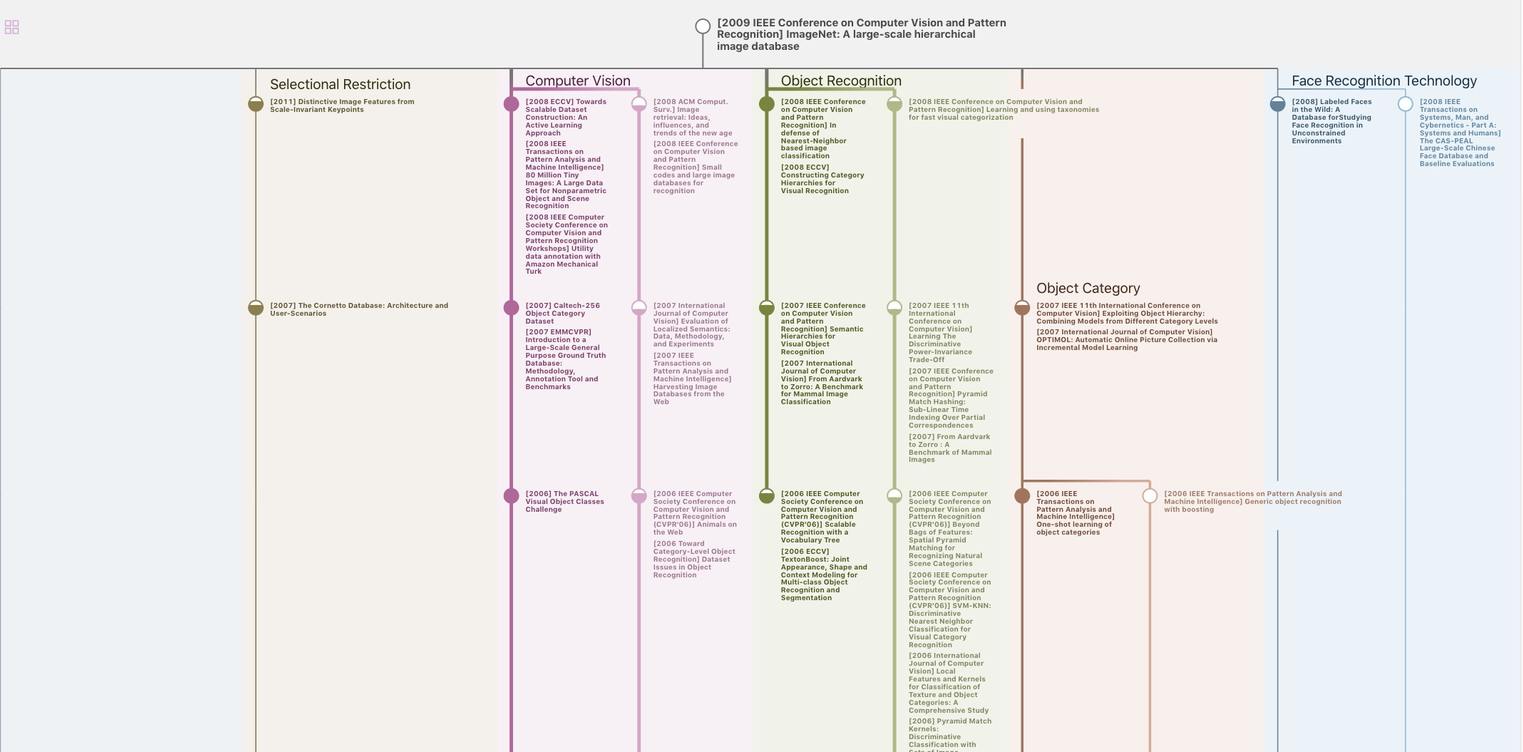
生成溯源树,研究论文发展脉络
Chat Paper
正在生成论文摘要