Macroscopic Traffic Stream Variables Prediction with Weather Impact Using Hybrid CNN-LSTM model.
ICDCN(2021)
摘要
Accurate prediction of the macroscopic traffic stream variables such as speed and flow is important for traffic operation and management in an intelligent transportation system. Adverse weather conditions like fog, snow, and rainfall affect the driver’s visibility, road capacity, and mobility. The accurate prediction of the traffic stream variables in adverse weather conditions is challenging because of the non-linear and complex characteristics of the traffic stream and spatiotemporal correlation between traffic and weather variables. Prolonged heavy rain causes massive waterlogging in developing countries due to weak drainage systems, narrow streets, and encroachment, further affecting these traffic stream variables. Snow reduces the road capacity as much as waterlogging does. Prolonged snowfall creates a thick layer on the road, which affects the traffic stream variables. Traffic data has a high spatial and temporal resolution compared to weather data, which makes the problem more challenging. In this paper, we define a soft temporal threshold to capture the prolonged impact of weather variables. To capture the traffic and weather data’s spatiotemporal and temporal features, we propose a hybrid CNN-LSTM model. To validate model performance, data from San Diego and Minneapolis Minnesota Twin city are used. The test experiments show that the hybrid CNN-LSTM model learns spatiotemporal and temporal features accurately compared to other deep learning models.
更多查看译文
AI 理解论文
溯源树
样例
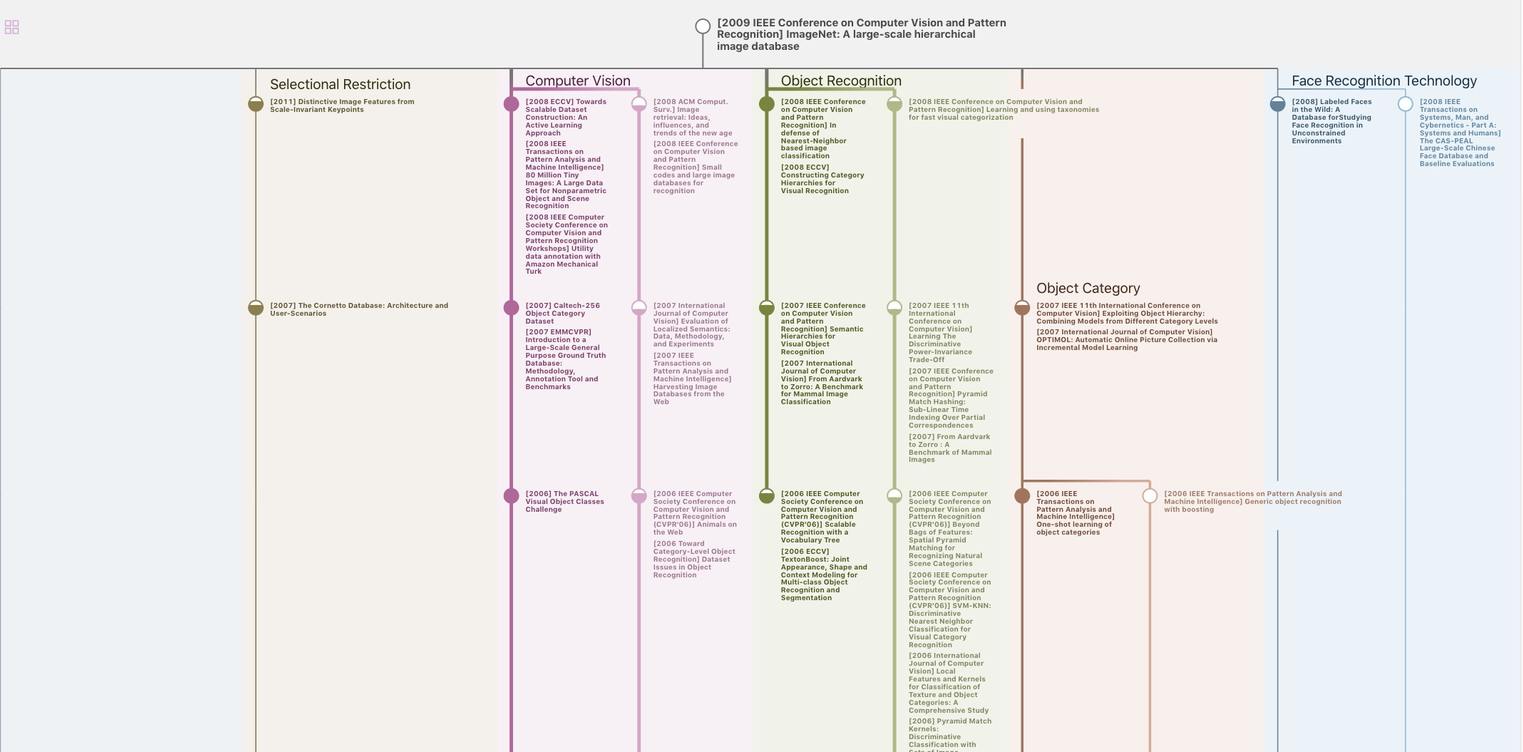
生成溯源树,研究论文发展脉络
Chat Paper
正在生成论文摘要