Variational Autoencoder Anomaly-Detection of Avalanche Deposits in Satellite SAR Imagery.
CI(2020)
摘要
This work demonstrates that deep unsupervised learning holds much promise for rare event detection, even when labeled data is limited. Rare event detection is challenging for traditional supervised learning approaches due to high class-imbalance. We demonstrate the efficacy of deep unsupervised learning, in particular a variational autoencoder (VAE), when used for anomaly detection, in an application to avalanche detection in the French Alps, from satellite SAR imagery and a limited on-the-ground survey. Remarkably, our results demonstrate that supervision (i.e., access to labeled data) is only needed in the validation phase of our training pipeline, to tune a hyper-parameter: the threshold on reconstruction error of the VAE (trained in an unsupervised fashion) that will be used to designate an observation as an anomaly, i.e., an avalanche in this context. Our method outperforms previous benchmarks in avalanche detection, including supervised learning a convolutional neural network on an artificially balanced version of the same data. To the best of our knowledge, this is the first step in exploring the potential of applying deep unsupervised learning methods to detect avalanche deposits. Our proposed semi-supervised learning pipeline is also promising for real-world settings in which the data is very unbalanced and labels are limited.
更多查看译文
关键词
unsupervised deep learning, anomaly detection, variational autoencoder, avalanche detection, satellite imaging
AI 理解论文
溯源树
样例
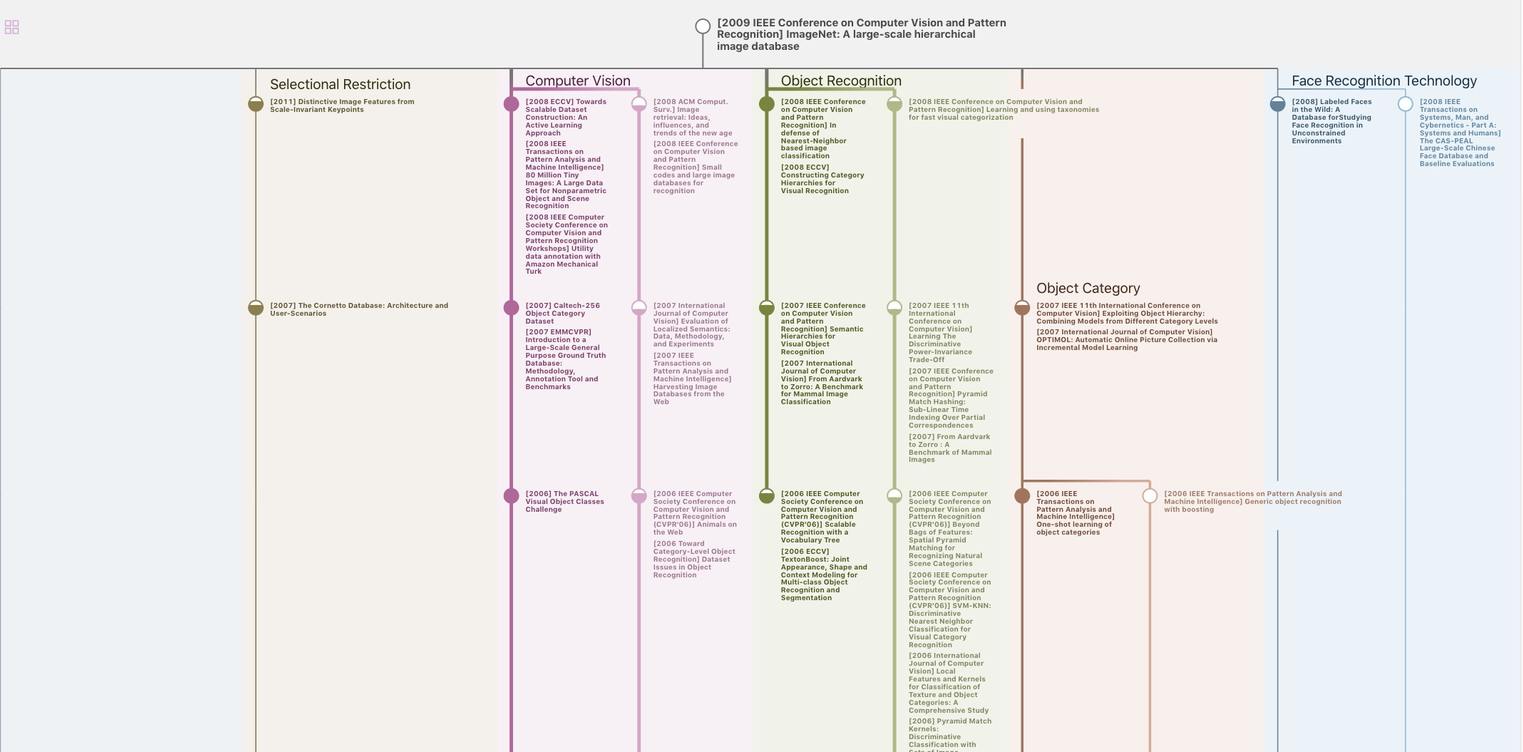
生成溯源树,研究论文发展脉络
Chat Paper
正在生成论文摘要