Model-Based Reinforcement Learning for Optimal Feedback Control of Switched Systems
2020 59th IEEE Conference on Decision and Control (CDC)(2020)
摘要
This paper examines the use of reinforcement learning-based controllers to approximate multiple value functions of specific classes of subsystems while following a switching sequence. Each subsystem may have varying characteristics, such as different cost or different system dynamics. Stability of the overall switching sequence is proven using Lyapunov-based analysis techniques. Specifically, Lyapunov-based methods are developed to prove boundedness of individual subsystems and to determine a minimum dwell-time condition to ensure stability of the overall switching sequence. Uniformly ultimately bounded regulation of the states, approximation of the value function, and approximation of the optimal control policy is achieved for arbitrary switching sequences provided the minimum dwell-time condition is satisfied.
更多查看译文
关键词
arbitrary switching sequences,minimum dwell-time condition,model-based reinforcement learning,reinforcement learning-based controllers,approximate multiple value functions,switching sequence,system dynamics,Lyapunov-based analysis techniques,Lyapunov-based methods,individual subsystems,value function,optimal control policy
AI 理解论文
溯源树
样例
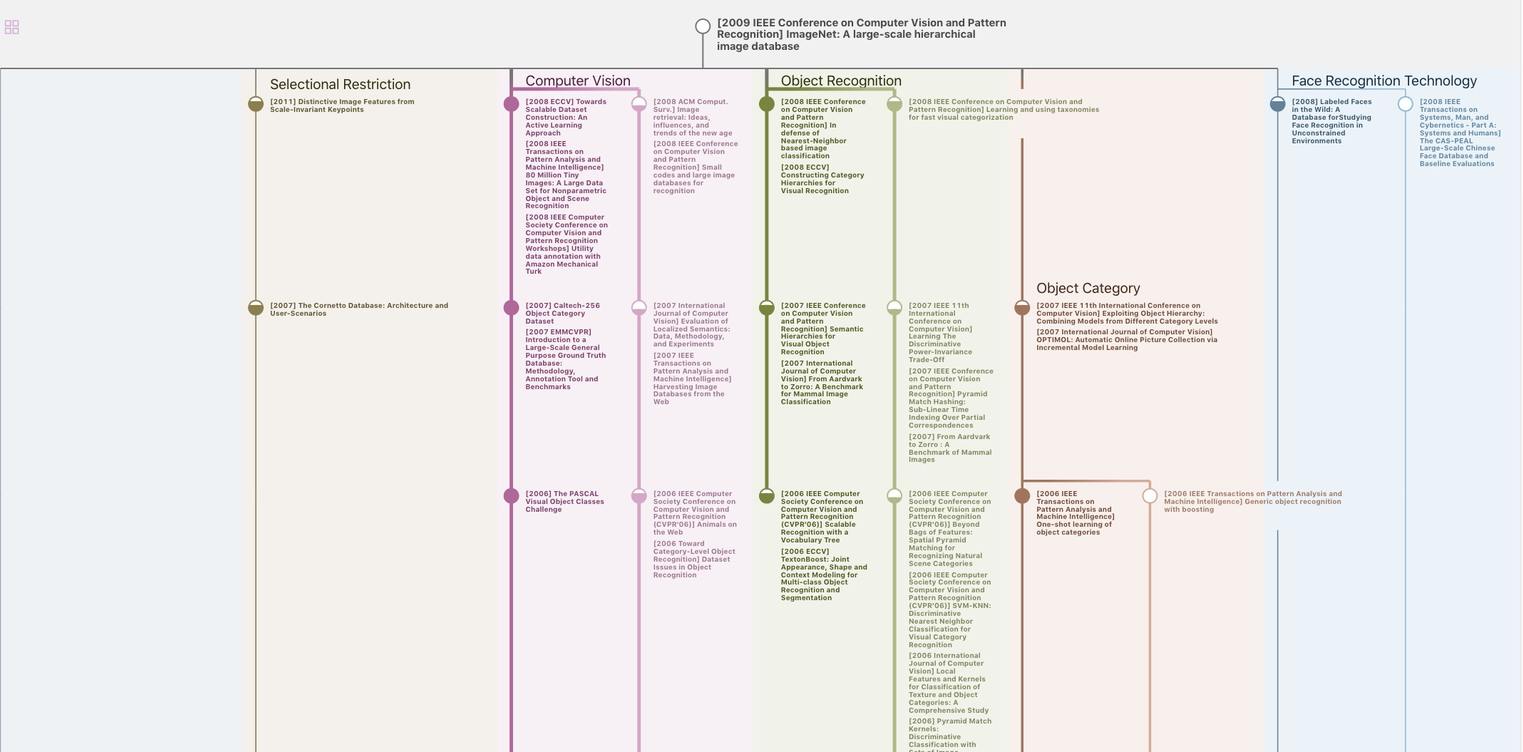
生成溯源树,研究论文发展脉络
Chat Paper
正在生成论文摘要