Predicting CRISPR-Cas9 Off-target with Self-supervised Neural Networks
2020 IEEE International Conference on Bioinformatics and Biomedicine (BIBM)(2020)
摘要
CRISPR-Cas9 is causing a new revolution in many fields such a s basic biological research, medicine, and biotechnology as the third-generation gene-editing tool. However, the phenomenon of off-target is a stumbling block to the vigorous development of gene-editing technology. In this paper, we proposed DNA-BERT by adding more meaningful tasks that learn regulatory sequence code from genomic sequence and remove useless tasks based on original Bidirectional Encoder Representations from Transformers (BERT) model to make it more suitable for DNA sequence tasks. Due to the lack of training samples, we use it to pre-training from massive genome data and use LightGBM(Light Gradient Boosting Model) to build a classification and regression model using DNA-BERT embeddings combine with hand-crafted features including mismatches, the secondary structure and so on. The empirical results from the public benchmark demonstrate that our method achieves better performance compared with state-of-art off-target methods (i.e. Elevation, DeepCRISPR, CNN-based method, CFD, MIT, CROP-IT, CCTop) on benchmark studies.
更多查看译文
关键词
Deep Learning,CRISPR-Cas9,Off-target Prediction
AI 理解论文
溯源树
样例
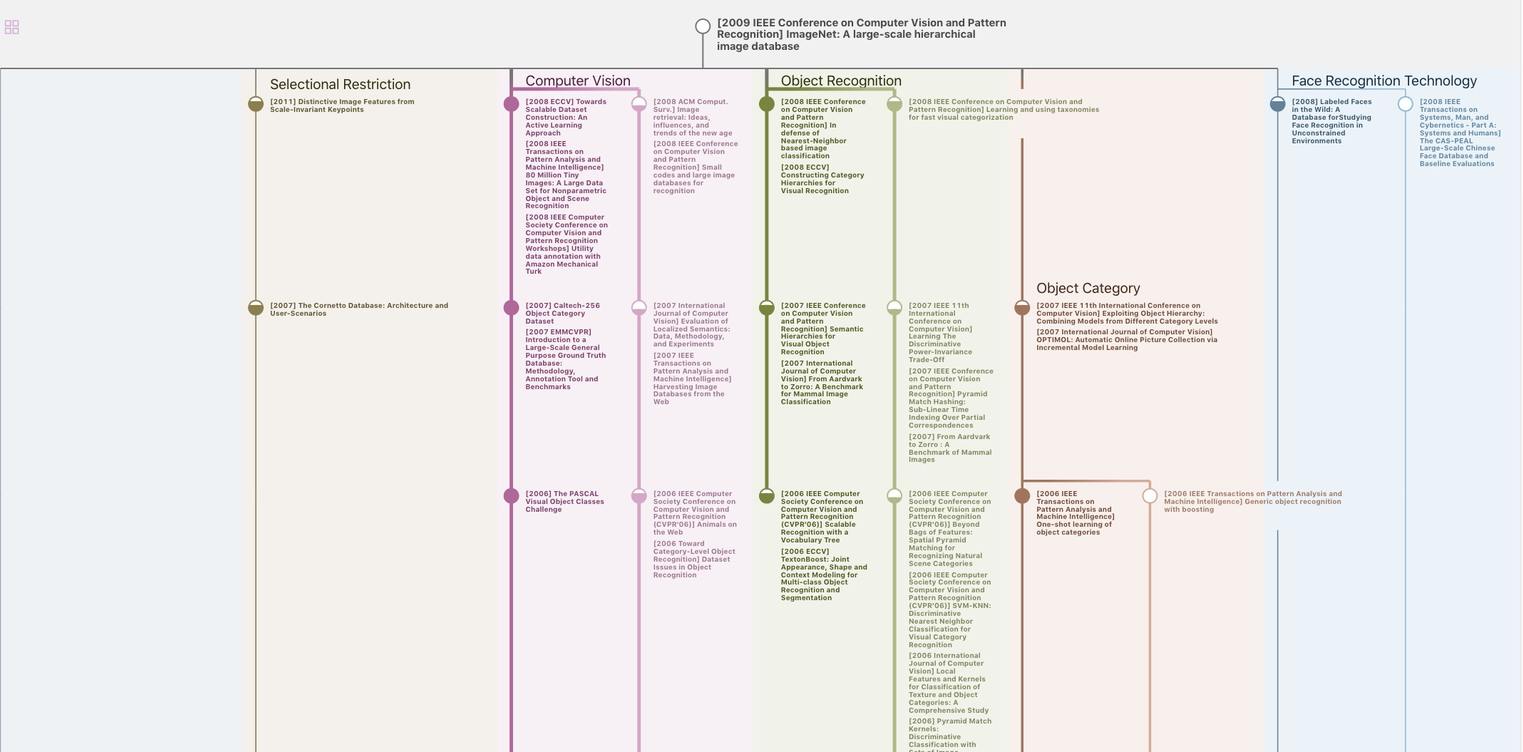
生成溯源树,研究论文发展脉络
Chat Paper
正在生成论文摘要