Predicting therapeutic outcomes in Rheumatoid Arthritis using real-world pharmacogenetic and clinical data
2020 IEEE International Conference on Bioinformatics and Biomedicine (BIBM)(2020)
摘要
OBJECTIVE: To develop a pharmacogenetic and clinical model to predict effectiveness outcomes in a cohort of patients diagnosed with rheumatoid arthritis (RA) treated with methotrexate. METHODS: Five machine learning methods were tested on the patient data set with previous preprocessing for dataset cleaning and feature selection: Logistic regression, decision trees, random forests, Support Vector Machines (SVM) and Artificial Neural Networks (ANN). The models were applied in a cohort of 155 patients treated with MTX which was derived in a training (124 patients) and a test cohort (31 patients). Both clinical variables and genetic variations were included. The chosen outcome was the therapy response measured as a DAS 28 <3.2. The performance evaluation criterion was the area (AUC) under the receiver operating characteristics (ROC) curve. RESULTS: The algorithms with the highest predictive power were SVM (AUC: 0.81, 95% CI: 0.74 - 0.90) and ANN (AUC: 0.82, 95% CI: 0.75 - 0.89). For the MTX cohort, the main selected variables were age, follow-up time, functional class, and genotypes of the rs9344, rs4148396, rs4673993, rs1801133 and rs7279445 variants. CONCLUSIONS: A prognostic model with high predictive power was developed in the cohort of patients treated with MTX, which is able to identify patients less likely not to respond to treatment.
更多查看译文
关键词
Rheumatoid Arthritis,Pharmacogenetics,Machine Learning,Data Mining,Real-World Data
AI 理解论文
溯源树
样例
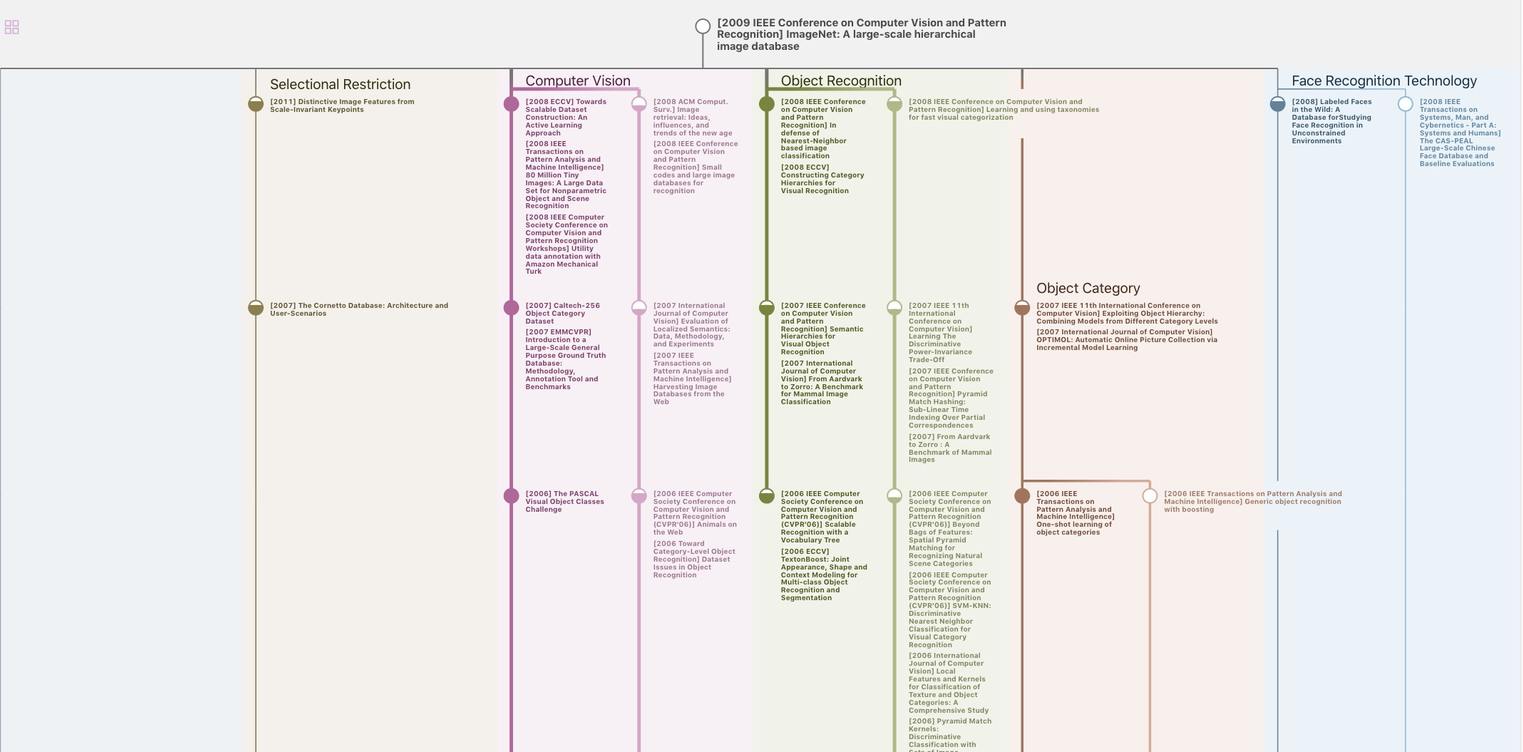
生成溯源树,研究论文发展脉络
Chat Paper
正在生成论文摘要