A Search and Filter Strategy for Identifying Differentially Co-Expressed Analyte Modules.
BIBM(2020)
摘要
Biological pathways underlying complex traits of interest are comprised of numerous analytes working in concert to produce the phenotype. Correlation network analysis (CNA) is widely used to identify these sets of co-expressed analytes (referred to as ‘modules’). A fundamental task in CNA is cluster identification. However, the objective of a clustering algorithm is to find an optimal partition of the data, while the goal of CNA is typically to find individual clusters of interest. Simply put, network clustering does not optimize for module identification. To address these issues we reframe CNA for module identification as a search problem and propose a novel, yet simple, method to identify group associated modules. Our method has two main innovations: Generalized Iterative Cluster Search for finding cancidate clusters, and similarity hyper-network sampling for reducing the search results to a set of best clusters for module significance testing. We then demonstrate the power of this method by applying it to lung tissue transcriptomics from a Chronic Obstructive Pulmonary Disease (COPD) case-control study, finding hundreds of modules with statistically significant group association.
更多查看译文
关键词
lung tissue transcriptomics,chronic obstructive pulmonary disease,hyper-network sampling,differentially co-expressed analyte modules,generalized iterative cluster search,module significance testing,group associated modules,search problem,module identification,network clustering,clustering algorithm,cluster identification,CNA,correlation network analysis,numerous analytes,biological pathways
AI 理解论文
溯源树
样例
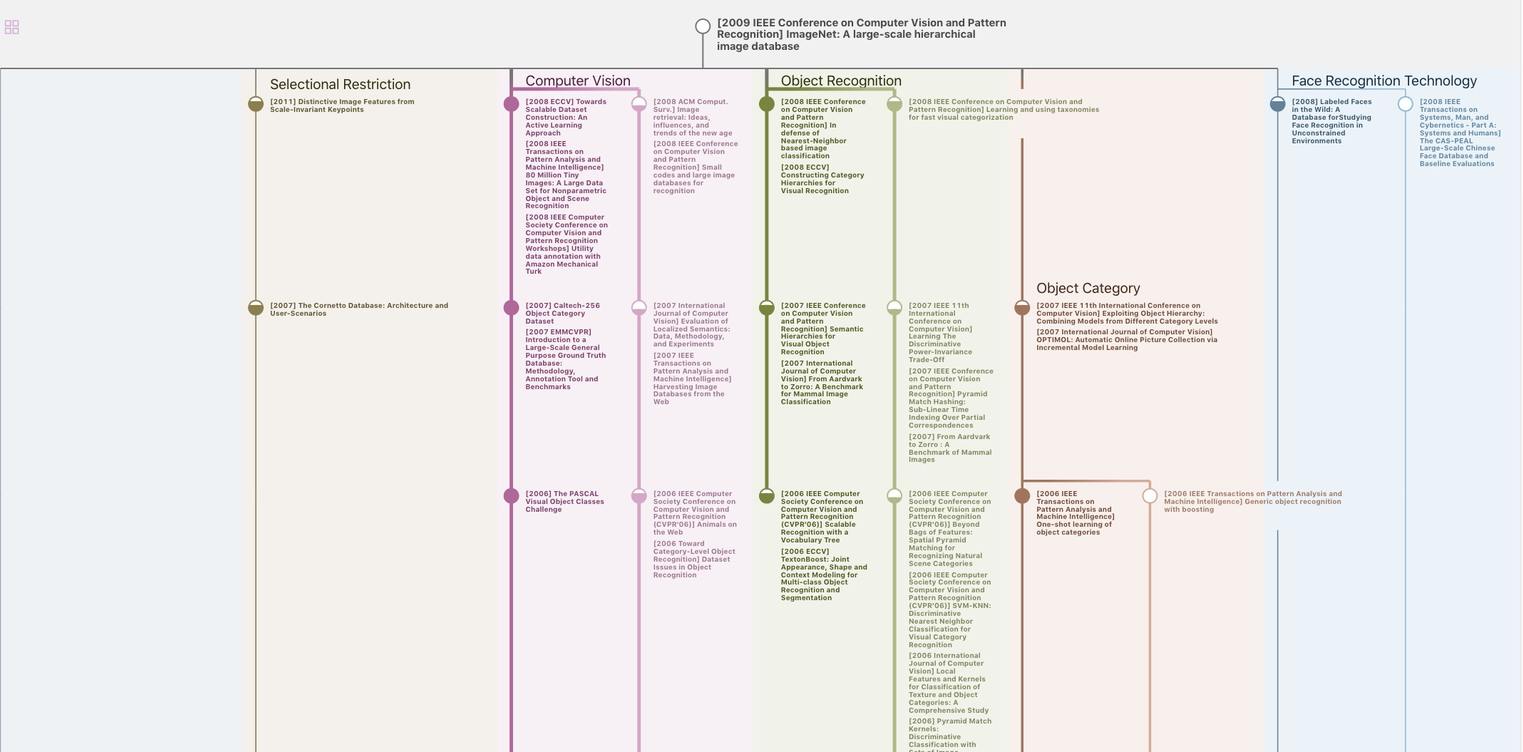
生成溯源树,研究论文发展脉络
Chat Paper
正在生成论文摘要