Joint Learning of Primary and Secondary Labels based on Multi-scale Representation for Alzheimer's Disease Diagnosis.
BIBM(2020)
摘要
The cause of Alzheimer's disease (AD) is insufficient to understand so far, and its diagnosis is challenging in clinical practice. Recently, the convolutional neural network (CNN) model has shown impressive performance in medical image analysis. Combining CNN with magnetic resonance imaging (MRI) image has excellent potential for AD diagnosis. However, it is still a challenging task. To address the challenge, we propose a joint learning method based on multi-scale representation (JL-MSR). The multi-scale representation is proposed to obtain more feature maps by the multi-scale atrous convolutions. Furthermore, in order to use the intrinsic relationship between diagnostic results and clinical scores, we propose a joint learning strategy using the diagnosis result as the primary label and the Mini-Mental State Examination (MMSE) score as the secondary label to joint training. The proposed method is evaluated on a dataset of 417 subjects (including 188 AD and 229 health controls (HC)) from the Alzheimer's Disease Neuroimaging Initiative (ADNI). The experimental results show that our proposed method achieves an accuracy of 88.1% and an area under the receiver operating characteristic (ROC) curve (AUC) value of 0.942 for AD diagnosis, respectively. Compared with a state-of-the-art method in AD diagnosis, our proposed method performs better, and has potential in clinical diagnosis.
更多查看译文
关键词
Alzheimer’s Disease Diagnosis,Atrous Convolution,Multi-scale Representation,Joint Learning
AI 理解论文
溯源树
样例
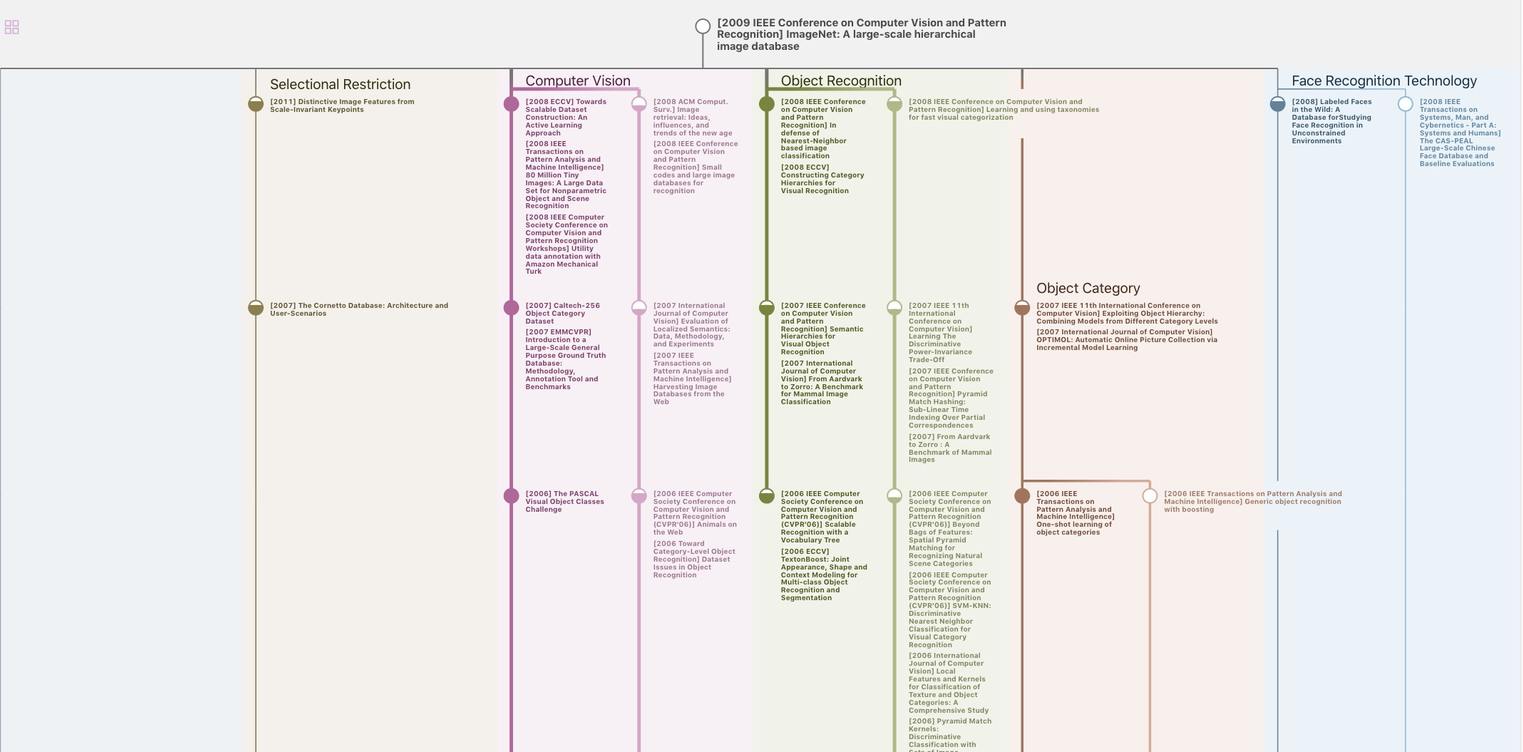
生成溯源树,研究论文发展脉络
Chat Paper
正在生成论文摘要