Deep Multi-Instance Learning with Induced Self-Attention for Medical Image Classification
2020 IEEE International Conference on Bioinformatics and Biomedicine (BIBM)(2020)
摘要
Existing Multi-Instance learning (MIL) methods for medical image classification typically segment an image (bag) into small patches (instances) and learn a classifier to predict the label of an unknown bag. Most of such methods assume that instances within a bag are independently and identically distributed. However, instances in the same bag often interact with each other. In this paper, we propose an Induced SelfAttention based deep MIL method that uses the self-attention mechanism for learning the global structure information within a bag. To alleviate the computational complexity of the naive implementation of self-attention, we introduce an inducing point based scheme into the self-attention block. We show empirically that the proposed method is superior to other deep MIL methods in terms of performance and interpretability on three medical image data sets. We also employ a synthetic MIL data set to provide an intensive analysis of the effectiveness of our method. The experimental results reveal that the induced self-attention mechanism can learn very discriminative and different features for target and non-target instances within a bag, and thus fits more generalized MIL problems.
更多查看译文
关键词
multi-instance learning,deep learning,selfattention,medical image classification
AI 理解论文
溯源树
样例
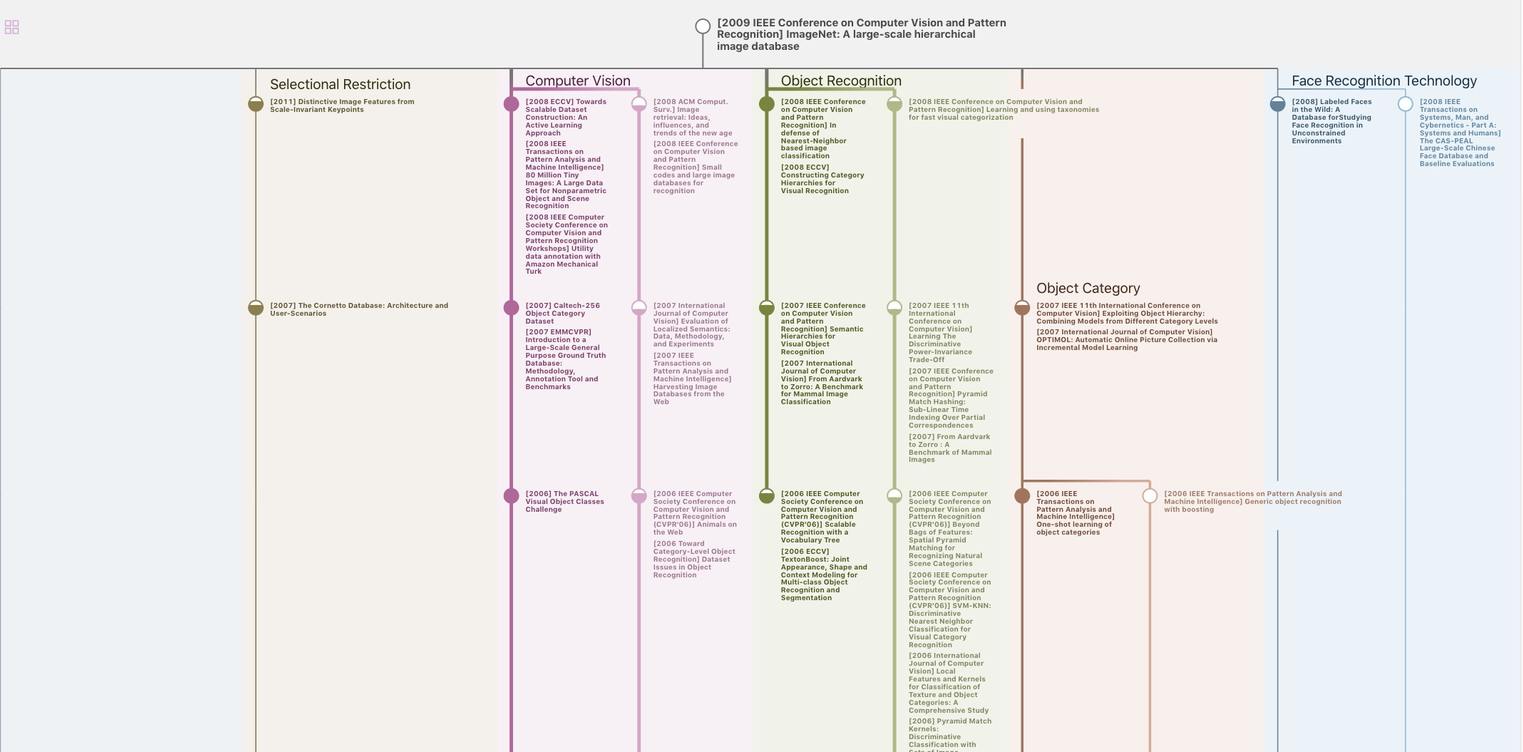
生成溯源树,研究论文发展脉络
Chat Paper
正在生成论文摘要