Accurate and Adversarially Robust Classification of Medical Images and ECG Time-Series with Gradient-Free Trained Sign Activation Neural Networks
IEEE International Conference on Bioinformatics and Biomedicine(2020)
摘要
Adversarial attacks in medical AI imaging systems can lead to misdiagnosis and insurance fraud as recently highlighted by Finlayson et. al. in Science 2019. They can also be carried out on widely used ECG time-series data as shown in Han et. al. in Nature Medicine 2020. At the heart of adversarial attacks are imperceptible distortions that are visually and statistically undetectable but cause the machine learning model to misclassify data. Recent empirical studies have shown that a gradient-free trained sign activation neural network ensemble model requires a larger distortion than state of the art models. We apply them on medical data in this study as a potential solution to detect and deter adversarial attacks. We show on chest X-ray and histopathology images, and on two ECG datasets that this model requires a greater distortion to be fooled than full-precision, binary, and convolutional neural networks, and random forests. We show that adversaries targeting the gradient-free sign networks are visually distinguishable from the original data and thus likely to be detected by human inspection. Since the sign network distortions are higher we expect an automated method could be developed to detect and deter attacks in advance. Our work here is a significant step towards safe and secure medical machine learning.
更多查看译文
关键词
histopathology,X-ray,ECG,adversarial attack,robust classification,gradient-free trained sign activation neural networks
AI 理解论文
溯源树
样例
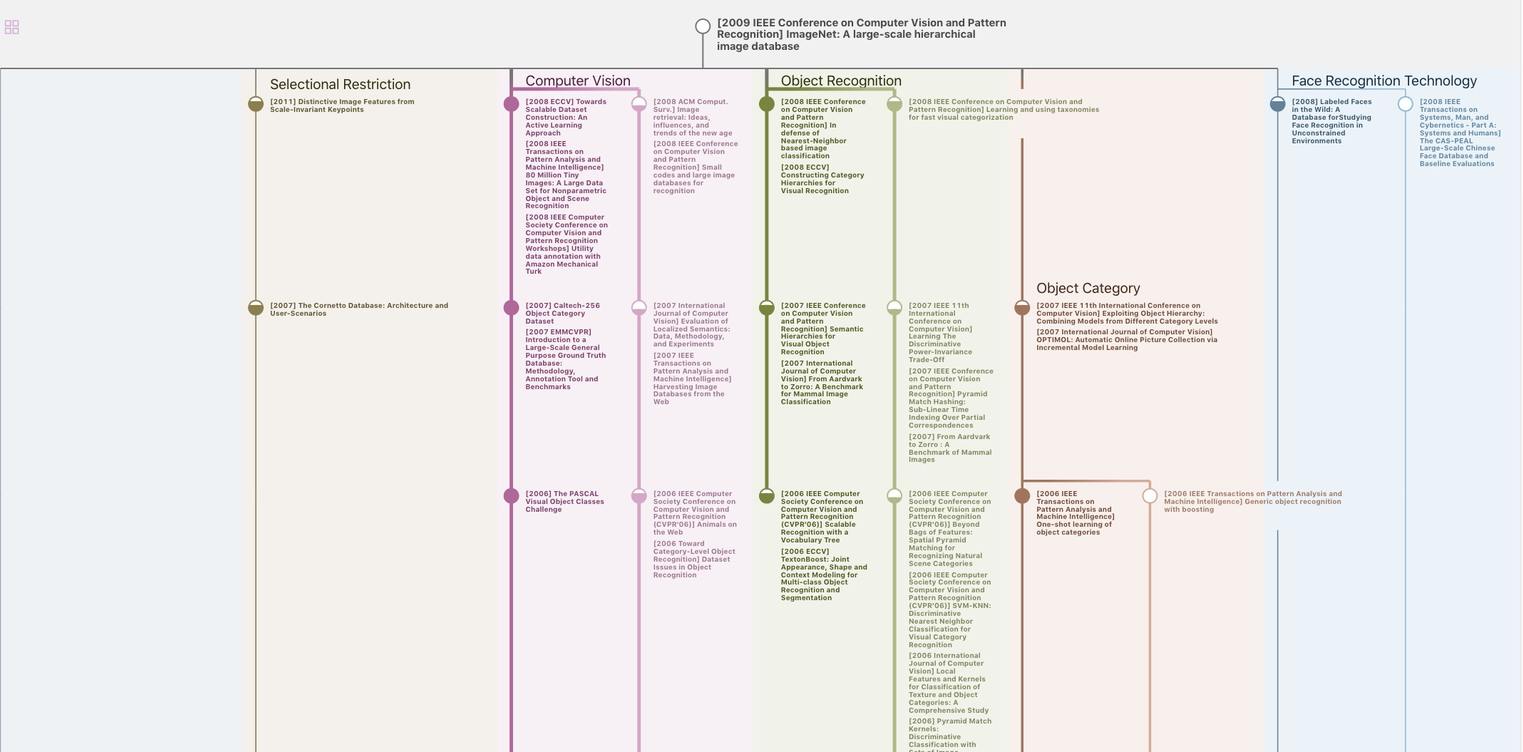
生成溯源树,研究论文发展脉络
Chat Paper
正在生成论文摘要