Implications of Public Cloud Resource Heterogeneity for Inference Serving
MIDDLEWARE(2020)
摘要
ABSTRACTWe are witnessing an increasing trend towards using Machine Learning (ML) based prediction systems, spanning across different application domains, including product recommendation systems, personal assistant devices, facial recognition, etc. These applications typically have diverse requirements in terms of accuracy and response latency, that can be satisfied by a myriad of ML models. However, the deployment cost of prediction serving primarily depends on the type of resources being procured, which by themselves are heterogeneous in terms of provisioning latencies and billing complexity. Thus, it is strenuous for an inference serving system to choose from this confounding array of resource types and model types to provide low-latency and cost-effective inferences. In this work we quantitatively characterize the cost, accuracy and latency implications of hosting ML inferences on different public cloud resource offerings. Our evaluation shows that, prior work does not solve the problem from both dimensions of model and resource heterogeneity. Hence, to holistically address this problem, we need to solve the issues that arise from combining both model and resource heterogeneity towards optimizing for application constraints. Towards this, we discuss the design implications of a self-managed inference serving system, which can optimize for application requirements based on public cloud resource characteristics.
更多查看译文
关键词
serverless, resource-management, inference
AI 理解论文
溯源树
样例
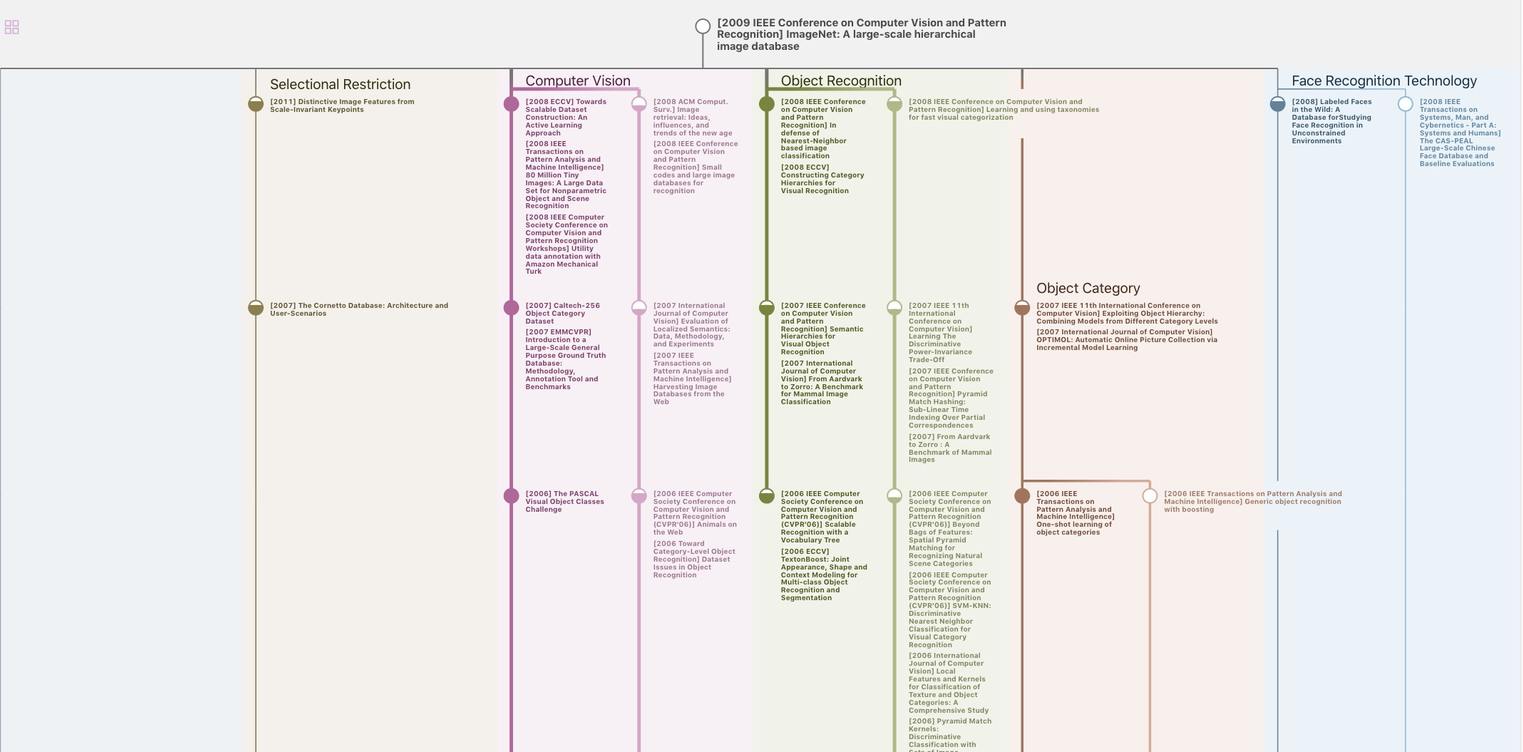
生成溯源树,研究论文发展脉络
Chat Paper
正在生成论文摘要