Wind field reconstruction from lidar measurements at high-frequency using machine learning
Journal of Physics Conference Series(2018)
摘要
The increase in wind turbine size during recent years has led to a situation where the wind speed measured at hub height is no longer a sufficient representation of the power available to be extracted by a turbine. Light Detection and Ranging ("Lidar") wind sensing devices can measure wind speeds in a volume in front of the sensor, offering affordable and improved estimation of wind turbine power performance and loading. However, a Lidar can only measure the component of the wind velocity in the direction of the beam. This makes reconstruction of the wind velocity field from raw Lidar measurements a challenge, and current methods contain assumptions which cause inaccuracies in complex terrain and in wind turbine wakes. In addition, data availability is reduced in specific weather conditions, such as very cold climates and dense fog. A novel Machine Learning method is developed here, based on Gaussian Process regression, to remove these assumptions when producing full 3D wind fields from Lidar measurements. This approach is naturally robust to overfitting and predicts uncertainty derived from data density and machine error, without needing physical information about the local terrain. Initial validation by comparison of a Windcube Lidar with a meteorological mast, on a site with simple conditions, shows excellent performance which meets the threshold for commercial use. The method also infers data during measurement gaps, offering the potential for 100% data availability. Instantaneous 2D wake measurements in a plane can be made, which when validated and further developed will offer a breakthrough in understanding of wind farm interactions and turbine loading.
更多查看译文
AI 理解论文
溯源树
样例
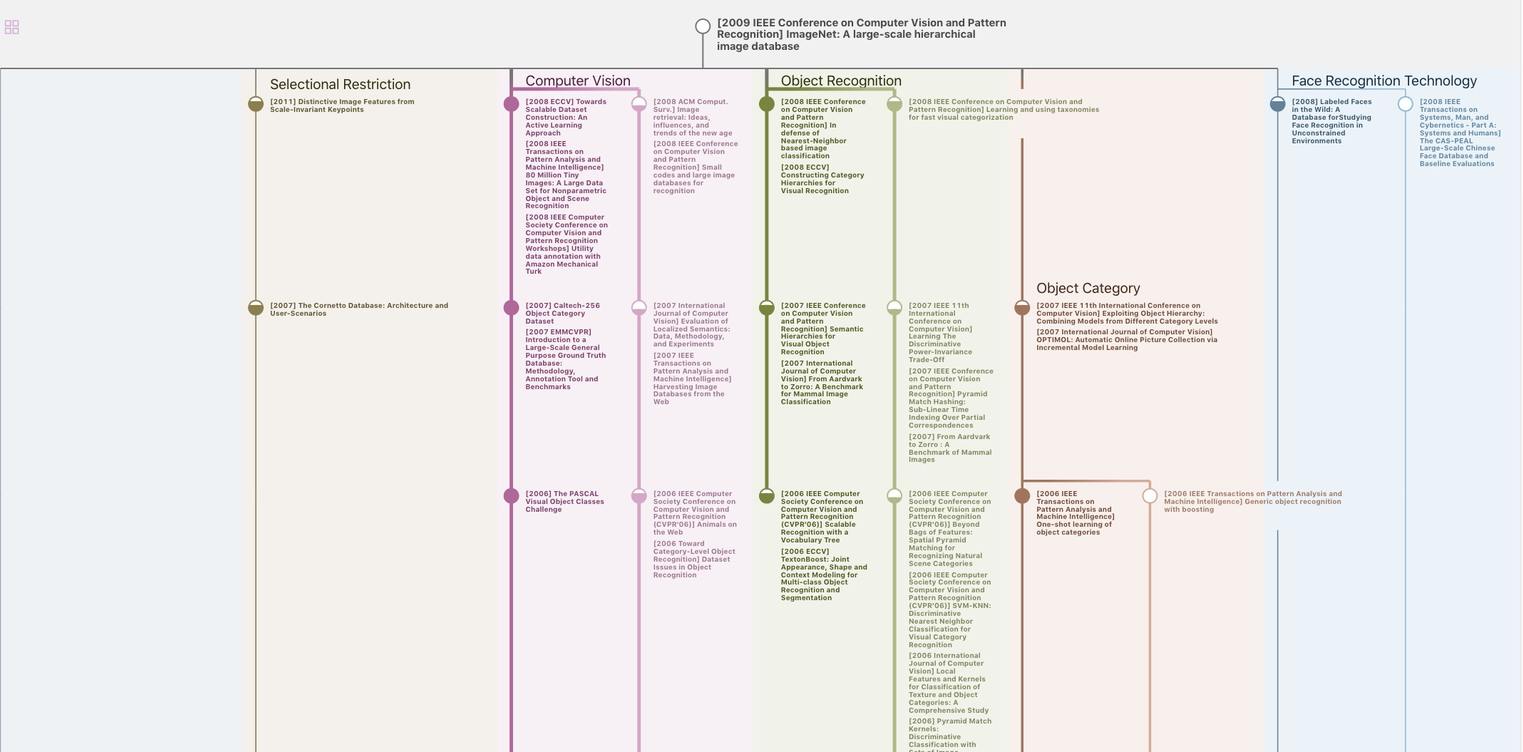
生成溯源树,研究论文发展脉络
Chat Paper
正在生成论文摘要