Modeling Autosomal Dominant Alzheimer'S Disease With Machine Learning
ALZHEIMERS & DEMENTIA(2021)
摘要
Introduction Machine learning models were used to discover novel disease trajectories for autosomal dominant Alzheimer's disease.Methods Longitudinal structural magnetic resonance imaging, amyloid positron emission tomography (PET), and fluorodeoxyglucose PET were acquired in 131 mutation carriers and 74 non-carriers from the Dominantly Inherited Alzheimer Network; the groups were matched for age, education, sex, and apolipoprotein epsilon 4 (APOE epsilon 4). A deep neural network was trained to predict disease progression for each modality. Relief algorithms identified the strongest predictors of mutation status.Results The Relief algorithm identified the caudate, cingulate, and precuneus as the strongest predictors among all modalities. The model yielded accurate results for predicting future Pittsburgh compound B (R-2 = 0.95), fluorodeoxyglucose (R-2 = 0.93), and atrophy (R-2 = 0.95) in mutation carriers compared to non-carriers.Discussion Results suggest a sigmoidal trajectory for amyloid, a biphasic response for metabolism, and a gradual decrease in volume, with disease progression primarily in subcortical, middle frontal, and posterior parietal regions.
更多查看译文
关键词
autosomal dominant Alzheimer's disease (ADAD), fluorodeoxyglucose (FDG), machine learning, magnetic resonance imaging (MRI), Pittsburgh compound B (PiB)
AI 理解论文
溯源树
样例
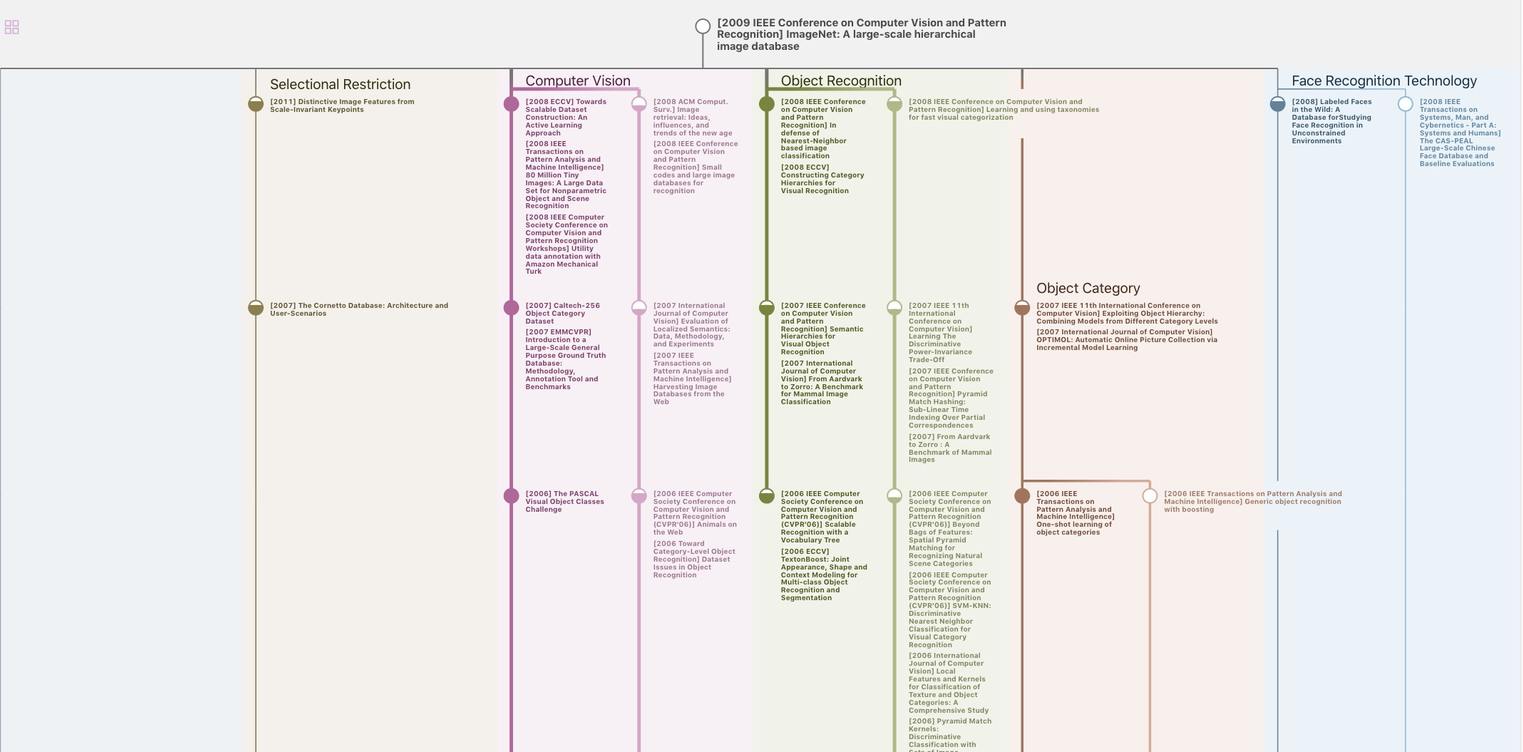
生成溯源树,研究论文发展脉络
Chat Paper
正在生成论文摘要