Experimentally realizing efficient quantum control with reinforcement learning
Science China Physics, Mechanics & Astronomy(2022)
摘要
We experimentally investigate deep reinforcement learning (DRL) as an artificial intelligence approach to control a quantum system. We verify that DRL explores fast and robust digital quantum controls with operation time analytically hinted by shortcuts to adiabaticity. In particular, the protocol’s robustness against both over-rotations and off-resonance errors can still be achieved simultaneously without any priori input. For the thorough comparison, we choose the task as single-qubit flipping, in which various analytical methods are well-developed as the benchmark, ensuring their feasibility in the quantum system as well. Consequently, a gate operation is demonstrated on a trapped 171 Yb + ion, significantly outperforming analytical pulses in the gate time and energy cost with hybrid robustness, as well as the fidelity after repetitive operations under time-varying stochastic errors. Our experiments reveal a framework of computer-inspired quantum control, which can be extended to other complicated tasks without loss of generality.
更多查看译文
关键词
quantum control,reinforcement learning,trapped ion,quantum computing,noise robustness
AI 理解论文
溯源树
样例
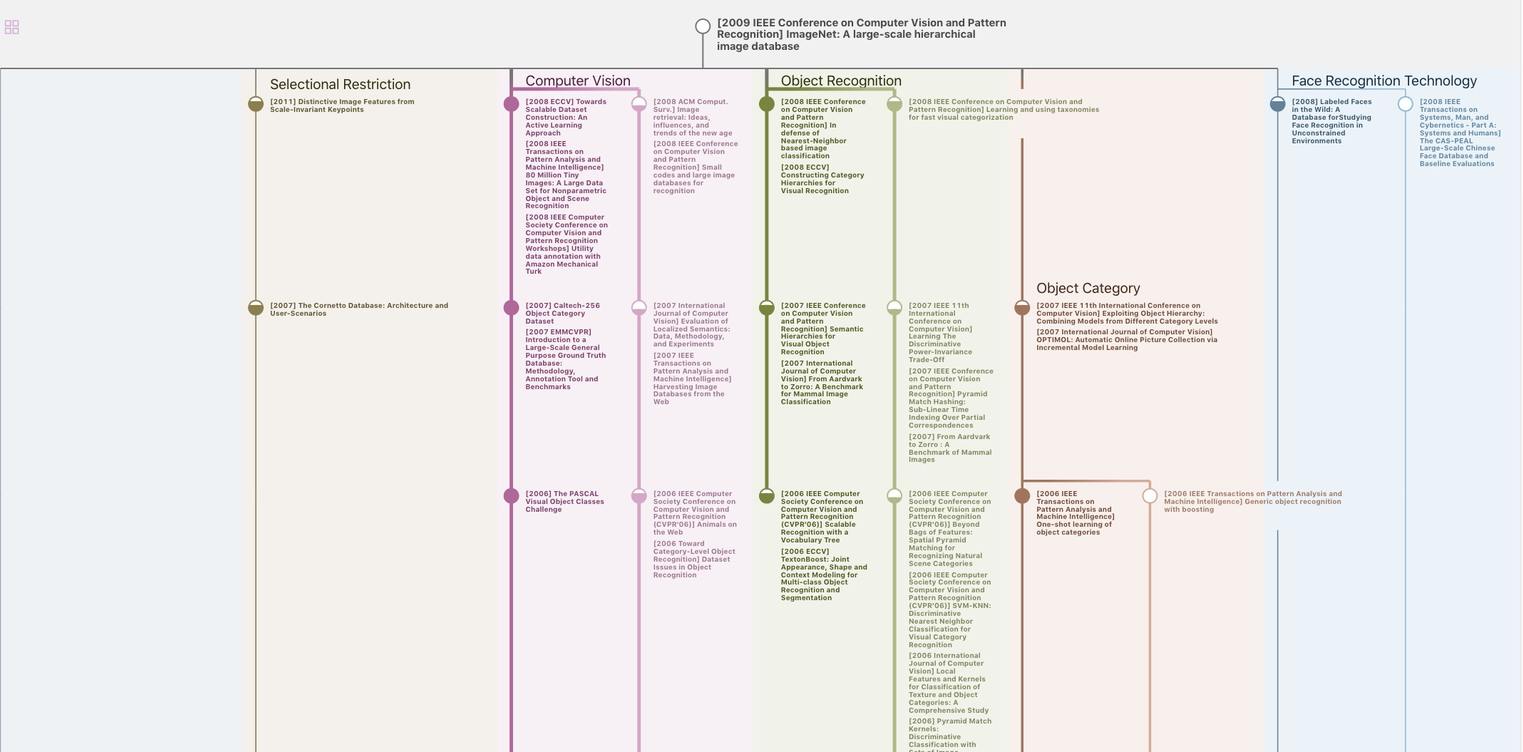
生成溯源树,研究论文发展脉络
Chat Paper
正在生成论文摘要