Failure Prediction By Regularized Fuzzy Learning With Intelligent Parameters Selection
APPLIED SOFT COMPUTING(2021)
摘要
Software failure time prediction, which is critical in software dependable evaluation, is among one of the most critical issues in software quality assurance research. Conventional software reliability predictions often use single models to illustrate the overall characteristic of the process of software failure. However, those models often fail to produce good prediction performance owing to the constant changes in software failure patterns during different time periods. Fuzzy systems like Takagi- Sugeno-Kang are multimodel approaches that combine simple submodels to represent the overall characteristic of dynamic nonlinear complex systems. This paper presents a novel Fuzzy system based software reliability prediction model, called regularized extreme learning adaptive neurofuzzy inference system, and employs the quantum-inspired binary gravitational search algorithm to determine its suitable regularization parameters. The new model can effectively reduce randomness, computational complexity as well as avoid local optimization when searching for optimal parameters. An experimentation using four real-world software failure data sets was carried out to compare the performance of the proposed model with that of certain representative software reliability prediction models. The results showed that the proposed model with the optimized parameters exhibits superior performance.(c) 2020 Elsevier B.V. All rights reserved.
更多查看译文
关键词
Dependable machine learning algorithms, Intelligent dependable modeling, Machine learning, Adaptive neuro-fuzzy inference system, Sequential behavior modeling and analysis
AI 理解论文
溯源树
样例
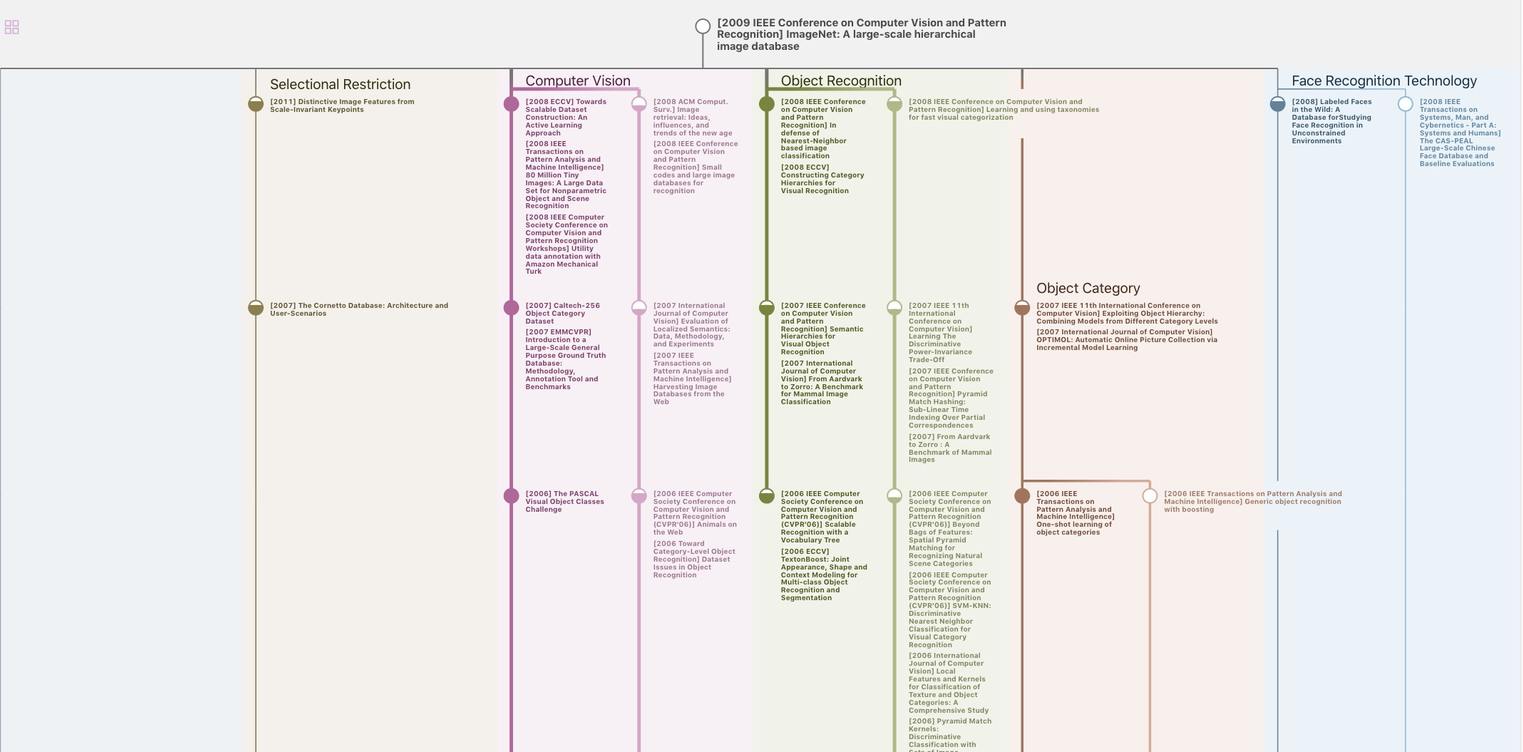
生成溯源树,研究论文发展脉络
Chat Paper
正在生成论文摘要