A Theoretical Revisit to Linear Convergence for Saddle Point Problems
ACM Transactions on Intelligent Systems and Technology(2021)
摘要
AbstractRecently, convex-concave bilinear Saddle Point Problems (SPP) is widely used in lasso problems, Support Vector Machines, game theory, and so on. Previous researches have proposed many methods to solve SPP, and present their convergence rate theoretically. To achieve linear convergence, analysis in those previouse studies requires strong convexity of φ(z). But, we find the linear convergence can also be achieved even for a general convex but not strongly convex φ(z). In the article, by exploiting the strong duality of SPP, we propose a new method to solve SPP, and achieve the linear convergence. We present a new general sufficient condition to achieve linear convergence, but do not require the strong convexity of φ(z). Furthermore, a more efficient method is also proposed, and its convergence rate is analyzed in theoretical. Our analysis shows that the well conditioned φ(z) is necessary to improve the efficiency of our method. Finally, we conduct extensive empirical studies to evaluate the convergence performance of our methods.
更多查看译文
关键词
Saddle point problems, linear convergence, strong convexity, min-max optimization
AI 理解论文
溯源树
样例
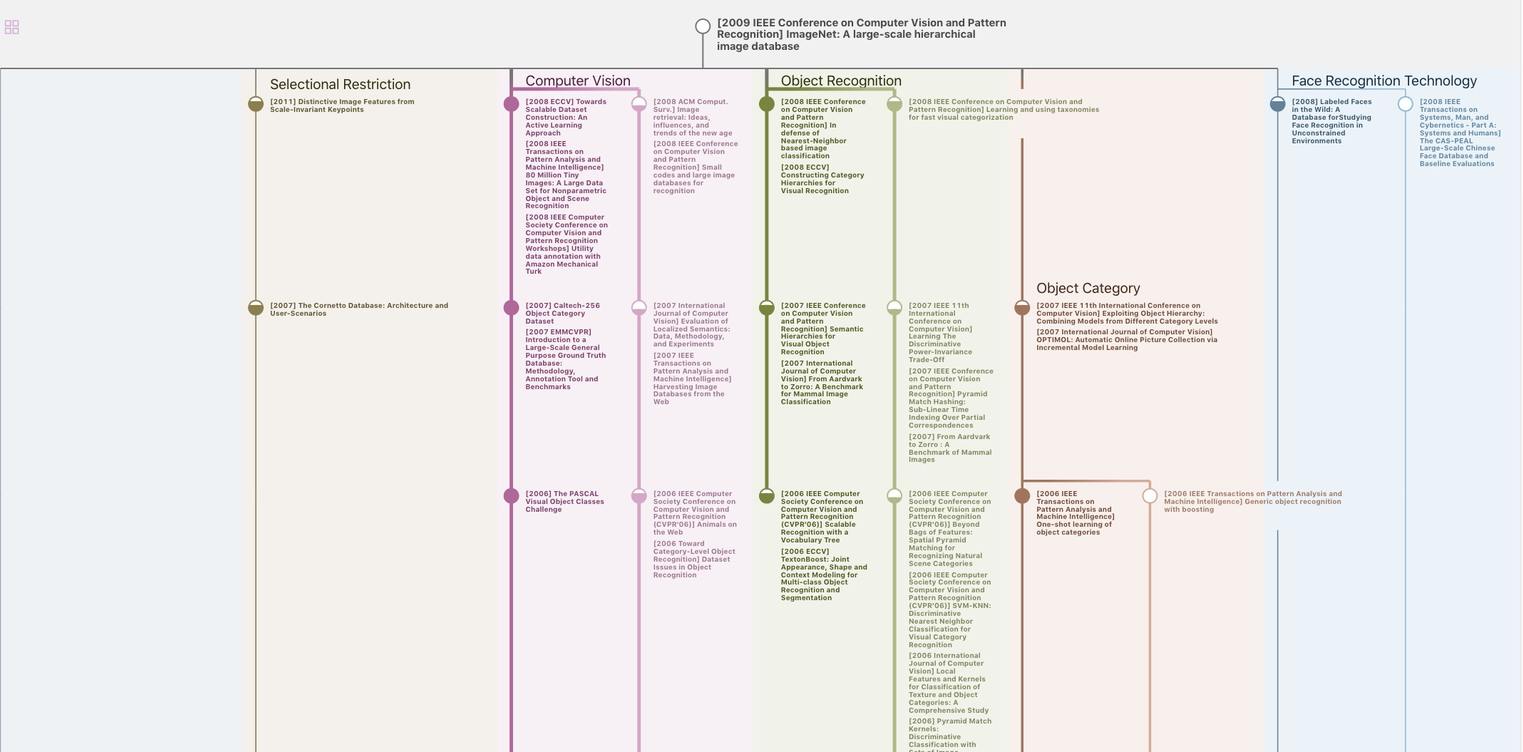
生成溯源树,研究论文发展脉络
Chat Paper
正在生成论文摘要