Fully Supervised and Guided Distillation for One-Stage Detectors.
ACCV (3)(2020)
摘要
Model distillation has been extended from image classification to object detection. However, existing approaches are difficult to focus on both object regions and false detection regions of student networks to effectively distill the feature representation from teacher networks. To address it, we propose a fully supervised and guided distillation algorithm for one-stage detectors, where an excitation and suppression loss is designed to make a student network mimic the feature representation of a teacher network in the object regions and its own high-response regions in the background, so as to excite the feature expression of object regions and adaptively suppress the feature expression of high-response regions that may cause false detections. Besides, a process-guided learning strategy is proposed to train the teacher along with the student and transfer knowledge throughout the training process. Extensive experiments on Pascal VOC and COCO benchmarks demonstrate the following advantages of our algorithm, including the effectiveness for improving recall and reducing false detections, the robustness on common one-stage detector heads and the superiority compared with state-of-the-art methods.
更多查看译文
关键词
guided distillation,detectors,one-stage
AI 理解论文
溯源树
样例
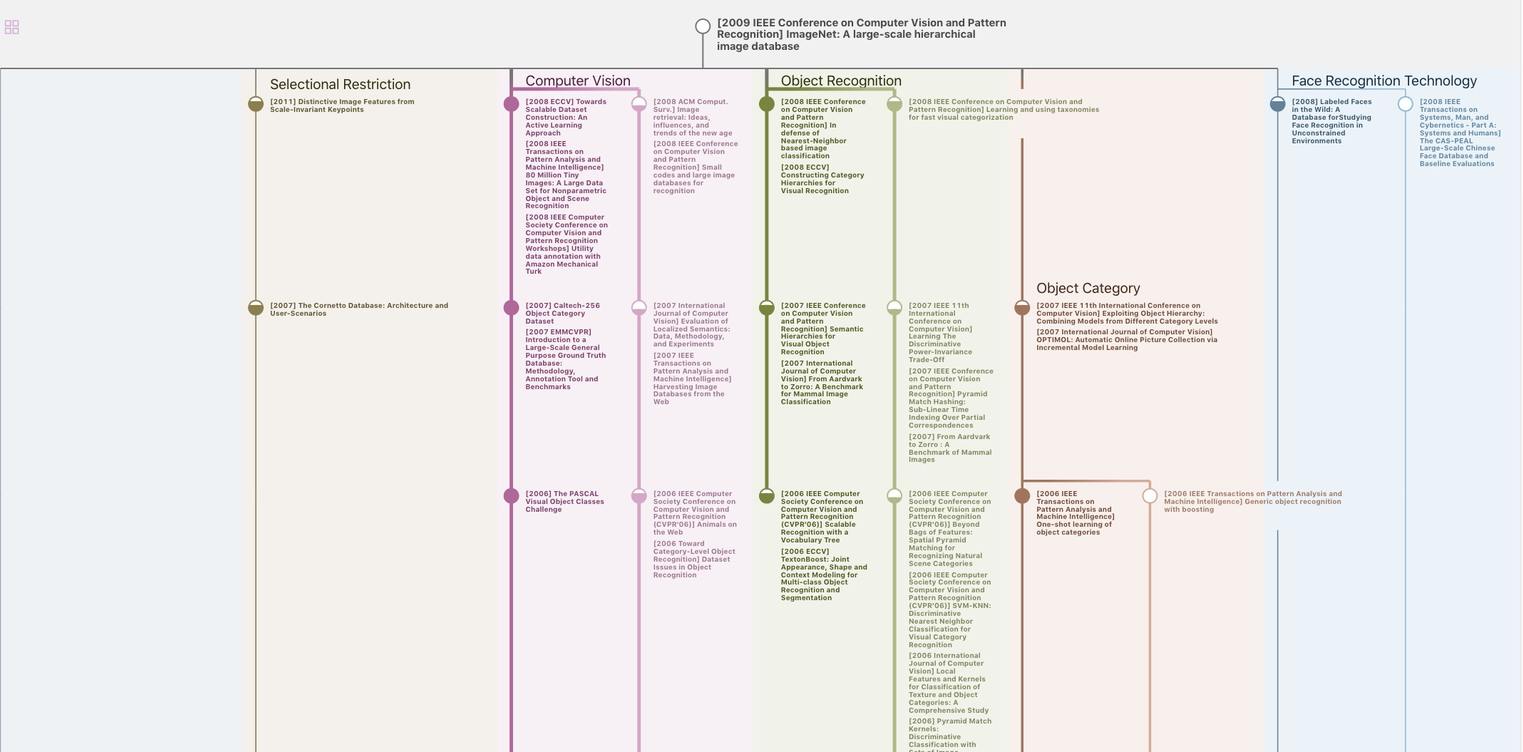
生成溯源树,研究论文发展脉络
Chat Paper
正在生成论文摘要