On the combination of data augmentation method and gated convolution model for building effective and robust intrusion detection
CYBERSECURITY(2020)
摘要
Deep learning (DL) has exhibited its exceptional performance in fields like intrusion detection. Various augmentation methods have been proposed to improve data quality and eventually to enhance the performance of DL models. However, the classic augmentation methods cannot be applied to those DL models which exploit the system-call sequences to detect intrusion. Previously, the seq2seq model has been explored to augment system-call sequences. Following this work, we propose a gated convolutional neural network (GCNN) model to thoroughly extract the potential information of augmented sequences. Also, in order to enhance the model’s robustness, we adopt adversarial training to reduce the impact of adversarial examples on the model. Adversarial examples used in adversarial training are generated by the proposed adversarial sequence generation algorithm. The experimental results on different verified models show that GCNN model can better obtain the potential information of the augmented data and achieve the best performance. Furthermore, GCNN with adversarial training can enhance robustness significantly.
更多查看译文
关键词
Data augmentation, Intrusion detection system, Machine learning algorithms, System call
AI 理解论文
溯源树
样例
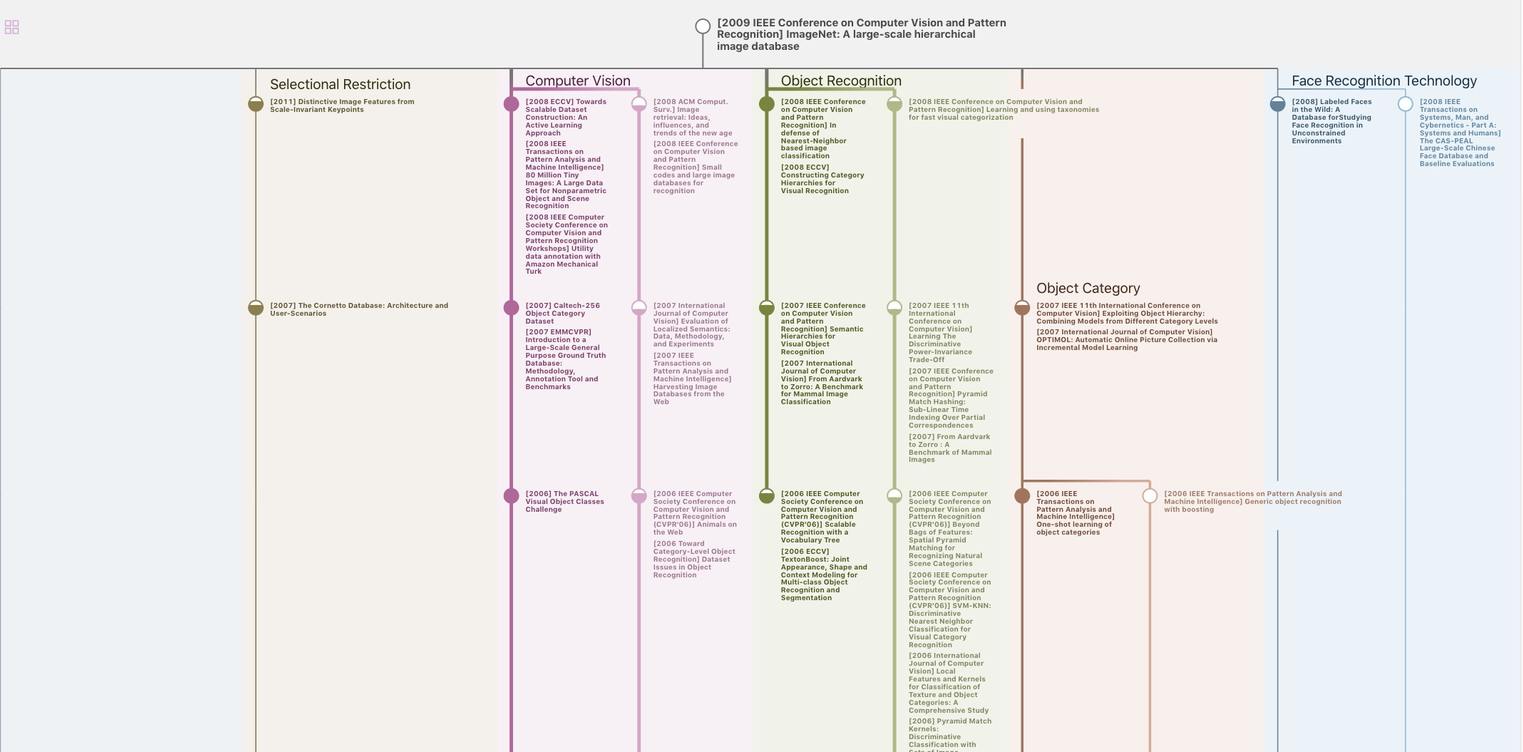
生成溯源树,研究论文发展脉络
Chat Paper
正在生成论文摘要