Designing A Bidirectional, Adaptive Neural Interface Incorporating Machine Learning Capabilities And Memristor-Enhanced Hardware
CHAOS SOLITONS & FRACTALS(2021)
摘要
Building bidirectional biointerfaces is one of the key challenges of modern engineering and medicine, with dramatic potential impact on bioprosthetics. Two of the major challenges of biointerface design concern signal stability and power efficiency. The former entails: a) ensuring that biosignal inputs corresponding to the same ground truth (e.g. patient "intentions") are recorded and interpreted consistently and b) maintaining the mapping from biointerface stimulation outputs to behavioral outputs (e.g. muscle movements). In this work we demonstrate how machine learning techniques, state-of-art nanoelectronics and microfluidics can combine forces to build and test low-power, adaptable biointerfaces that address both key challenges. Specifically, we demonstrate that: 1) we can emulate the input/output transfer characteristics of a structure biological neural network (BNN) with an artificial one (ANN), 2) it is possible to translate the resulting, "ideally trained" ANN into a hardware network using RRAM devices as synapses without significant loss of accuracy, despite concerns in the community about RRAM device reliability and 3) using a very simple mechanism of shifting the active stimulation electrode can fully restore functionality after the initial stimulation site degrades, prolonging the usable lifetime of the biointerface significantly. In this manner we place a key stepping stone towards building self-adjusting, low-power biointerfaces, themselves a foundational stepping stone towards adaptable, low-power bioprostheses. (C) 2020 Elsevier Ltd. All rights reserved.
更多查看译文
关键词
Memristors, Artificial neural network, Design, Simulation, Accuracy, Neural interface, Neuro-hybrid systems, Neuromorphic computing
AI 理解论文
溯源树
样例
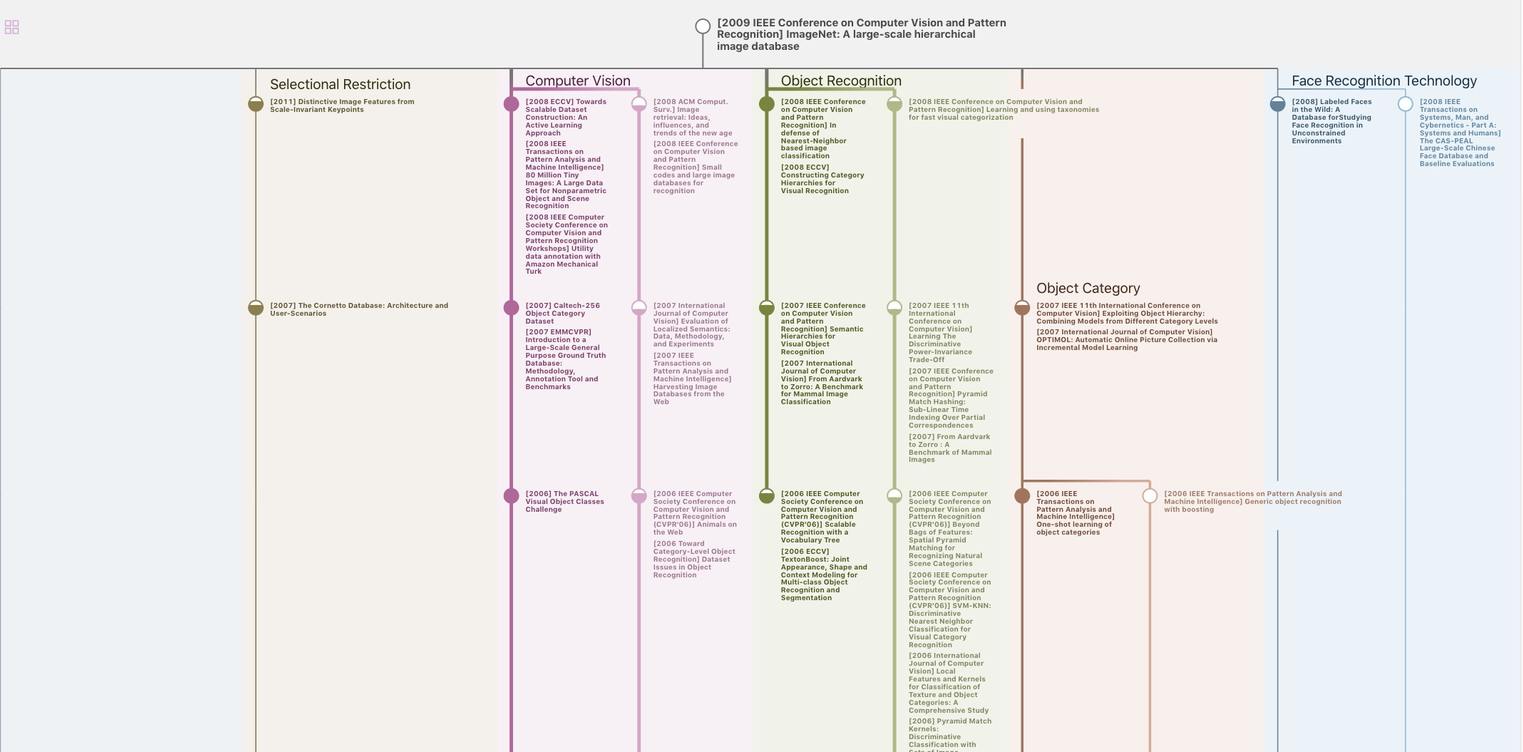
生成溯源树,研究论文发展脉络
Chat Paper
正在生成论文摘要