Feature-ranking-based ensemble classifiers for survivability prediction of intensive care unit patients using lab test data
Informatics in Medicine Unlocked(2021)
摘要
Clinical decision support systems (CDSSs) have received increasing research attention in recent years because they can improve the quality, safety, efficiency, and effectiveness of healthcare. A CDSS combined with advanced data analytics is more accurate and efficient than traditional systems. In this domain, survival or deterioration prediction of critical care patients, e.g., intensive care unit (ICU) patients, is an active research area. Early deterioration prediction can help healthcare providers in providing efficient and effective patient care. Research in this field is primarily based on vital signs. However, very few studies have investigated survival prediction using lab test data. Although some studies have made advancements in this field, accuracy remains insufficient. Thus, this study aims to improve the accuracy and efficiency of survival prediction for ICU patients. We propose a feature-ranking-based ensemble of classifiers for survival prediction of ICU patients using only lab test data. In the proposed method, features are evaluated first, and subsets of useful features are selected. Subsequently, training data with the selected features are clustered using a feature vector compaction (FVC) technique. Finally, ensemble classifier models are trained. Extensive experiments with over 3000 different settings on six ICU patient datasets were performed to evaluate the efficacy of the proposed method. The proposed technique achieves weighted average F1 score (Fwa) as high as 82.6% with support vector machine classifier when feature ranking is used with a combination of vertical and horizontal grouping-based FVC. All experimental results demonstrate that this technique outperforms existing methods, with the Fwa score difference being as high as 4.5%.
更多查看译文
关键词
ICU Patients,Feature vector compaction,Feature grouping,Lab test data,Clinical prediction,Clustering
AI 理解论文
溯源树
样例
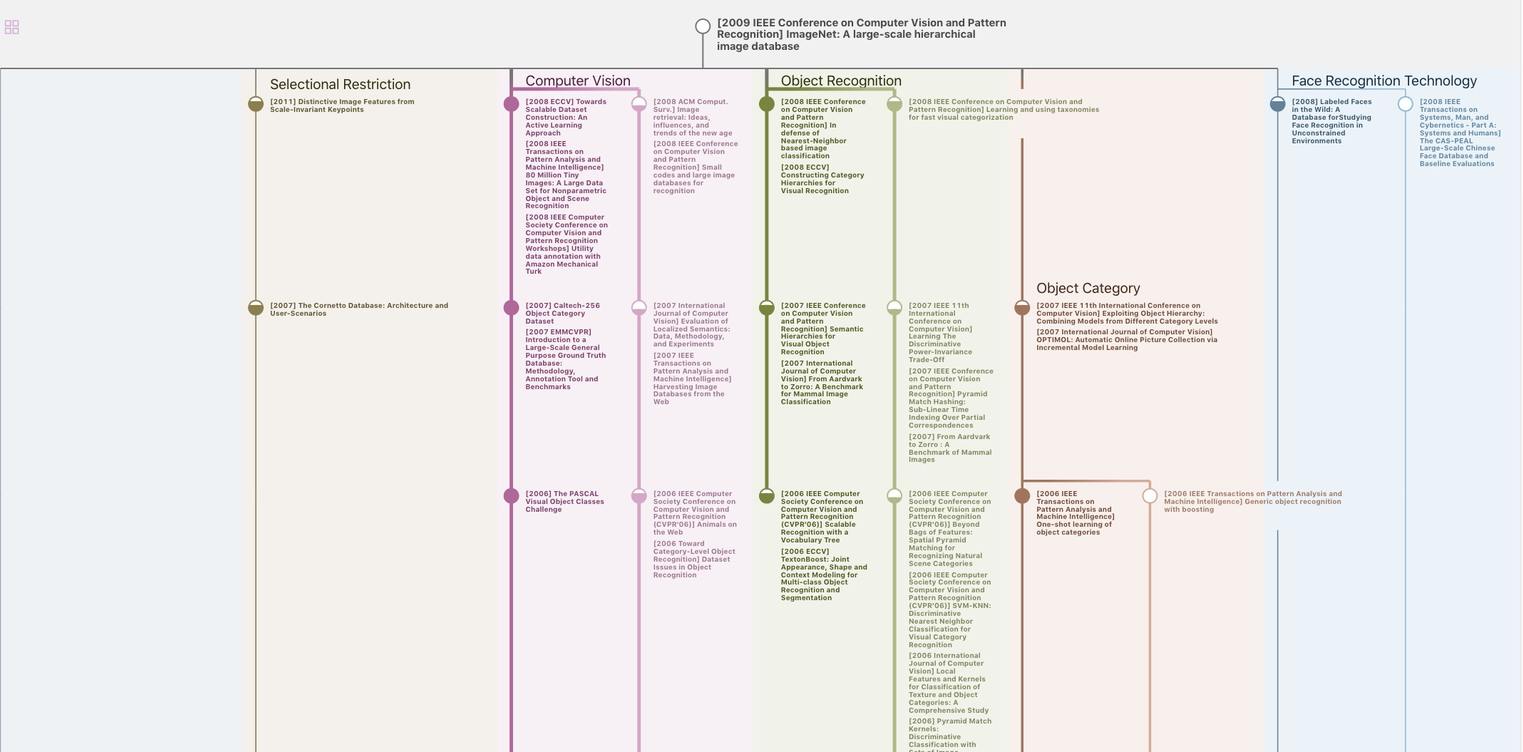
生成溯源树,研究论文发展脉络
Chat Paper
正在生成论文摘要