Isotope Identification Using Deep Learning: an Explanation
NUCLEAR INSTRUMENTS & METHODS IN PHYSICS RESEARCH SECTION A-ACCELERATORS SPECTROMETERS DETECTORS AND ASSOCIATED EQUIPMENT(2021)
摘要
The exceptional performance of machine learning methods has led to their adaptation in many different domains. In the nuclear industry, it has been proposed that machine learning methods have the potential to revolutionize nuclear safety and radiation detection by leveraging that they can be used to augment human and device capabilities. While many applications focus on the accuracy of the learning algorithm’s prediction, it has been shown in practice that these algorithms are prone to learn characteristics that are not descriptive or relevant. Hence, this paper focuses on understanding the reasoning behind the classification using saliency methods. Visual representations of the network’s learned regions of interest are used to demonstrate whether domain-specific characteristics are being identified, which allows for the end-user to evaluate the performance based on domain knowledge. The results obtained show that focusing on a human-centered approach will ultimately enhance the transparency and trust of the deep learning algorithm’s decision.
更多查看译文
关键词
Nuclear science,Robust artificial intelligence,Explainable deep learning,Gamma-ray spectroscopy
AI 理解论文
溯源树
样例
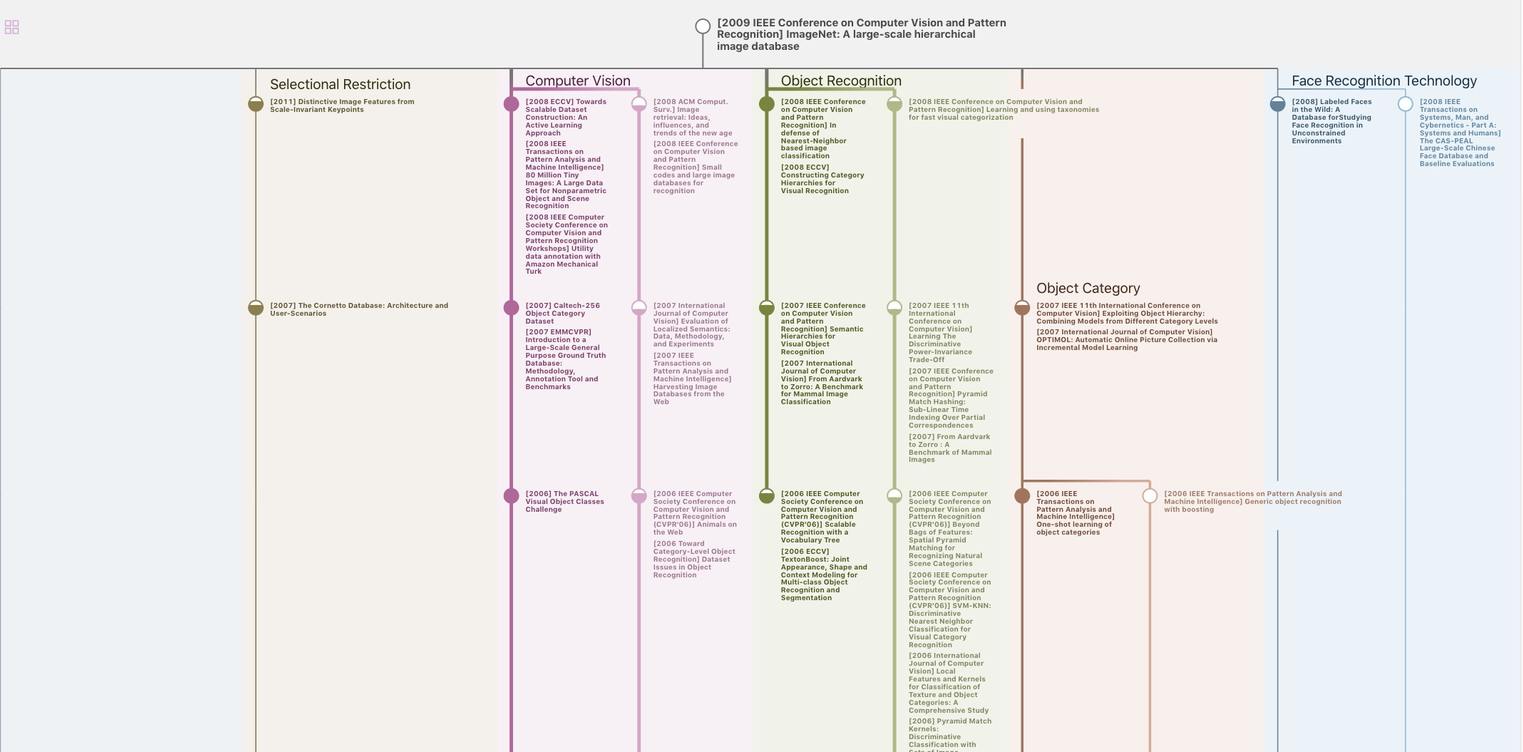
生成溯源树,研究论文发展脉络
Chat Paper
正在生成论文摘要