Use of Machine Learning and Linked Population Health Data to Develop Predictive Risk Algorithms for Population Health Decision-Making
International Journal for Population Data Science(2020)
摘要
IntroductionData from population health surveys, administrative health records and environmental monitoring are increasingly being linked at the individual level. As these data become available to health researchers, there is an increasing need for methods which can make sense of large, noisy and heterogeneous data and can model complex relationships. Using these data, machine learning methods have the potential to produce population health risk algorithms with better performance than those developed with traditional statistical approaches.
Objectives and ApproachThe objective of this work is to explore the use of machine learning methods for the development, validation and implementation of predictive risk algorithms designed specifically for population health planning purposes. Algorithms to predict risk of dementia and avoidable hospitalizations are in development using the Canadian Community Health Survey, geographic sociodemographic information, administrative health care utilization data and vital statistics. Methods being explored include naive Bayes, gradient boosting, support vector machines and neural networks.
ResultsRisk algorithms for population health should generally prioritize calibration over discrimination due to implications for resource allocation decisions. Approaches to minimize the risk of overfitting should be used and reweighting of unbalanced data avoided as it distorts the population-level nature of the data. It is important to be aware of propagating underlying bias in the data or exacerbating existing health inequities, which can be evaluated in part through assessment of calibration across relevant population subgroups. Approaches that consider multi-level data structures are needed to appropriately incorporate neighbourhood-level measures with individual-level information. To maximize population health impact and acceptability, model transparency and interpretability should be prioritized.
ConclusionThere is tremendous potential for machine learning approaches to leverage large volumes of linked population data to produce predictive risk algorithms that will inform population health decision-making. Future work will explore use of complex environmental remote sensing and built environment data.
更多查看译文
关键词
linked population health data,predictive risk algorithms,machine learning,decision-making
AI 理解论文
溯源树
样例
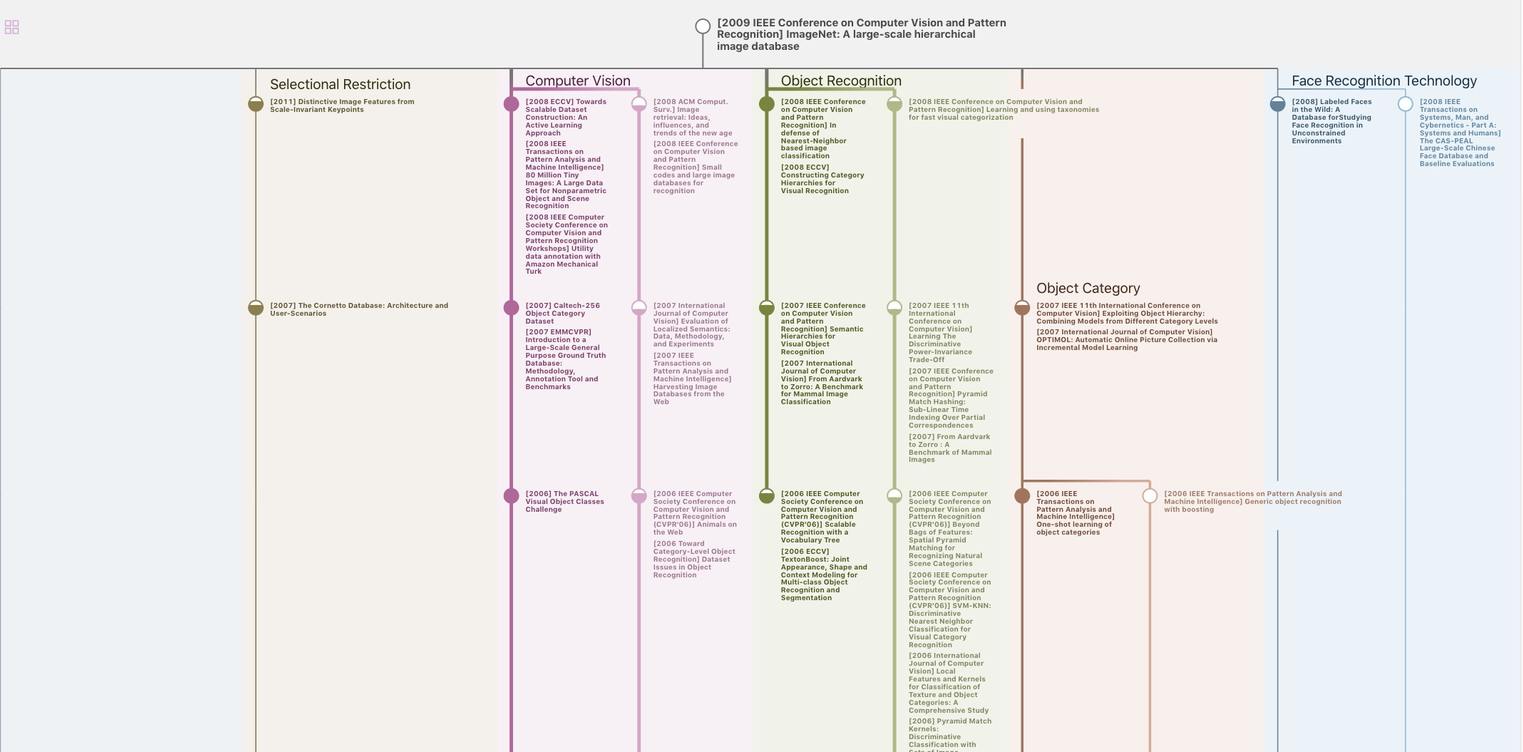
生成溯源树,研究论文发展脉络
Chat Paper
正在生成论文摘要