Pre-selected class-level testing of longitudinal biomarkers reduces required multiple testing corrections to yield novel insights in longitudinal small sample human studies.
Statistical Communications in Infectious Diseases(2020)
摘要
Objectives:Exploratory studies that aim to evaluate novel therapeutic strategies in human cohorts often involve the collection of hundreds of variables measured over time on a small sample of individuals. Stringent error control for testing hypotheses in this setting renders it difficult to identify statistically signification associations. The objective of this study is to demonstrate how leveraging prior information about the biological relationships among variables can increase power for novel discovery.
Methods:We apply the class level association score statistic for longitudinal data (CLASS-LD) as an analysis strategy that complements single variable tests. An example is presented that aims to evaluate the relationships among 14 T-cell and monocyte activation variables measured with CD4 T-cell count over three time points after antiretroviral therapy (n=62).
Results:CLASS-LD using three classes with emphasis on T-cell activation with either classical vs. intermediate/inflammatory monocyte subsets detected associations in two of three classes, while single variable testing detected only one out of the 14 variables considered.
Conclusions:Application of a class-level testing strategy provides an alternative to single immune variables by defining hypotheses based on a collection of variables that share a known underlying biological relationship. Broader use of class-level analysis is expected to increase the available information that can be derived from limited sample clinical studies.
更多查看译文
AI 理解论文
溯源树
样例
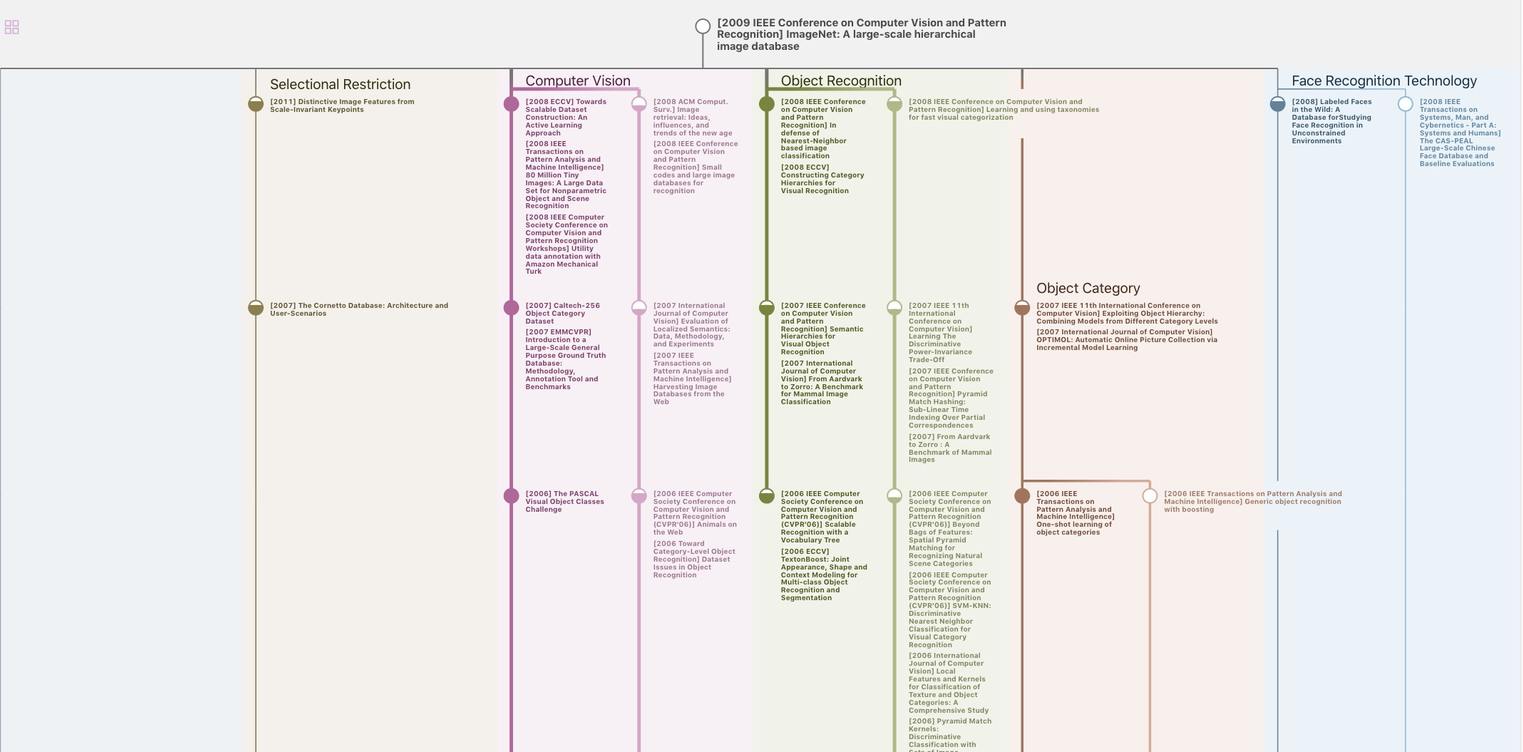
生成溯源树,研究论文发展脉络
Chat Paper
正在生成论文摘要