Changepoint inference in the presence of missing covariates for principal surrogate evaluation in vaccine trials
BIOMETRIKA(2021)
摘要
We consider the use of threshold-based regression models to evaluate immune response biomarkers as principal surrogate markers of a vaccine's protective effect. Threshold-based regression models, which allow the relationship between a clinical outcome and a covariate to change dramatically across a threshold value in the covariate, have been studied by various authors under fully observed data. Limited research, however, has examined these models in the presence of missing covariates, such as the counterfactual potential immune responses of a participant in the placebo arm of a standard vaccine trial had they been assigned to the vaccine arm instead. Based on a hinge model for a threshold effect of the principal surrogate on vaccine efficacy, we develop a regression method that consists of two components: (i) an estimated likelihood method for handling missing potential outcomes, and (ii) a penalty imposed on the estimated likelihood to ensure satisfactory finite-sample performance. We develop a method that allows joint estimation of all model parameters, as well as a two-step method that separates estimation of the threshold parameter from the rest of the parameters. Stable iterative algorithms are developed to implement the two methods, and the asymptotic properties of the proposed estimators are established. In simulation studies, the proposed estimators are shown to have satisfactory finite-sample performance. The proposed methods are applied to real data collected from dengue vaccine efficacy trials to predict how vaccine efficacy varies with an individual's potential immune response if receiving the vaccine.
更多查看译文
关键词
Hinge threshold regression modelling, Missing potential outcome, Penalized estimated likelihood, Principal surrogate, Vaccine efficacy
AI 理解论文
溯源树
样例
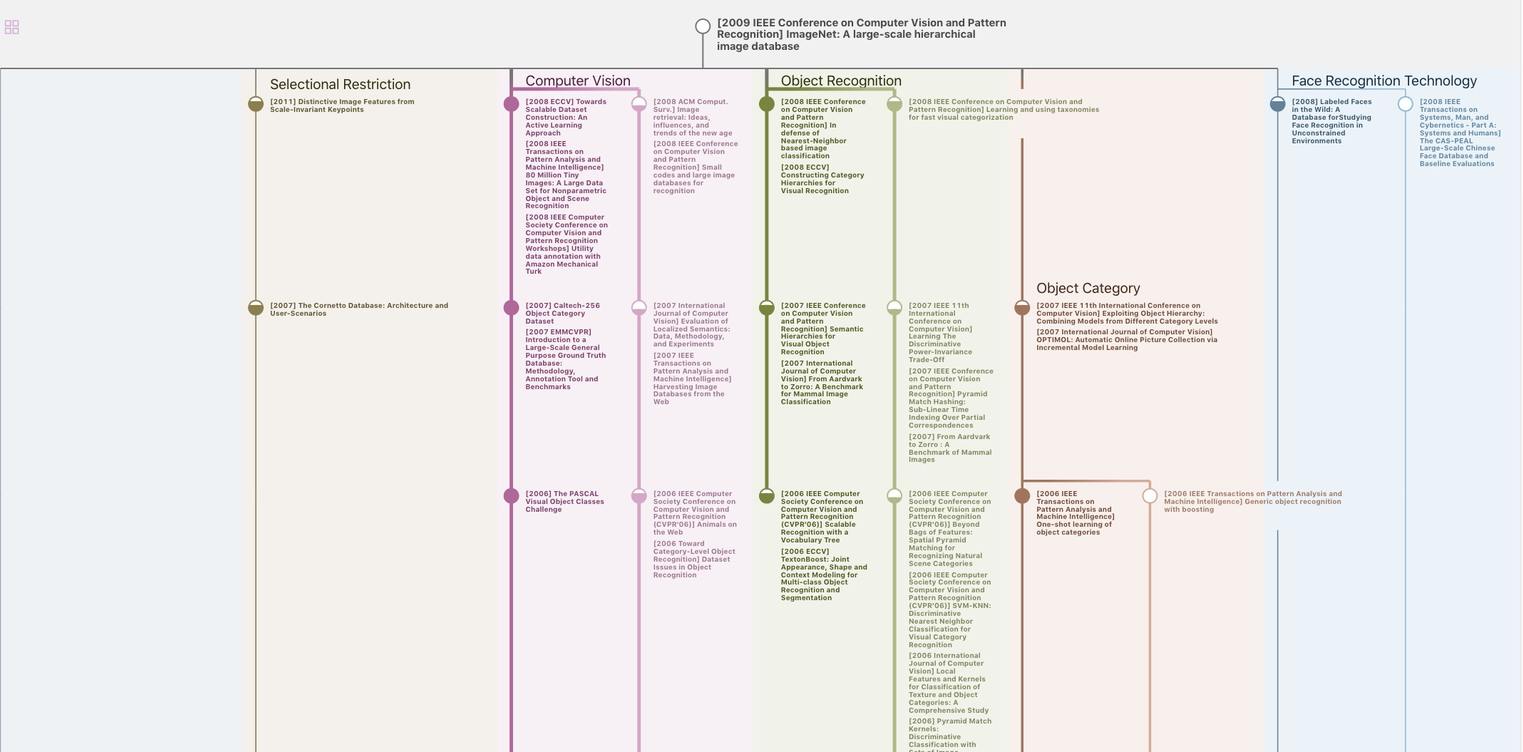
生成溯源树,研究论文发展脉络
Chat Paper
正在生成论文摘要