Cyberattack Detection for Electric Vehicles Using Physics-Guided Machine Learning
IEEE Transactions on Transportation Electrification(2021)
摘要
With the continuous development of intellectualization and network interconnection, the cyber-physical security of power electronics systems has become increasingly prominent. Particularly, powertrain systems composed of one or more electric drives in modern electric vehicles (EVs) are becoming more vulnerable to cyberthreats due to the connection to external networks in the intelligent traffic environment. In this article, we design a physics-guided machine learning to detect cyberattacks on EVs considering varying driving scenarios, which, to the best if our knowledge, has not been attempted before. To reflect the transient physical characteristics of EVs, we collect both device-level (e.g., current and voltage in the motor drive) and vehicle-level signals. Then, innovative data features concerning critical system performance and physical dynamics of the vehicle are proposed, with which we leverage data-driven methodology with high-fidelity physical power electronics and vehicular models. Based on the physics-guided data features, a machine-learning-based classifier is developed and validated in an OPAL-RT hardware-in-the-loop (HIL) simulation testbed and demonstrates high accuracy under various driving scenarios.
更多查看译文
关键词
Cyberattack detection,cyber–physical security,electric vehicles (EVs),physics-guided machine learning,power-train system in EVs
AI 理解论文
溯源树
样例
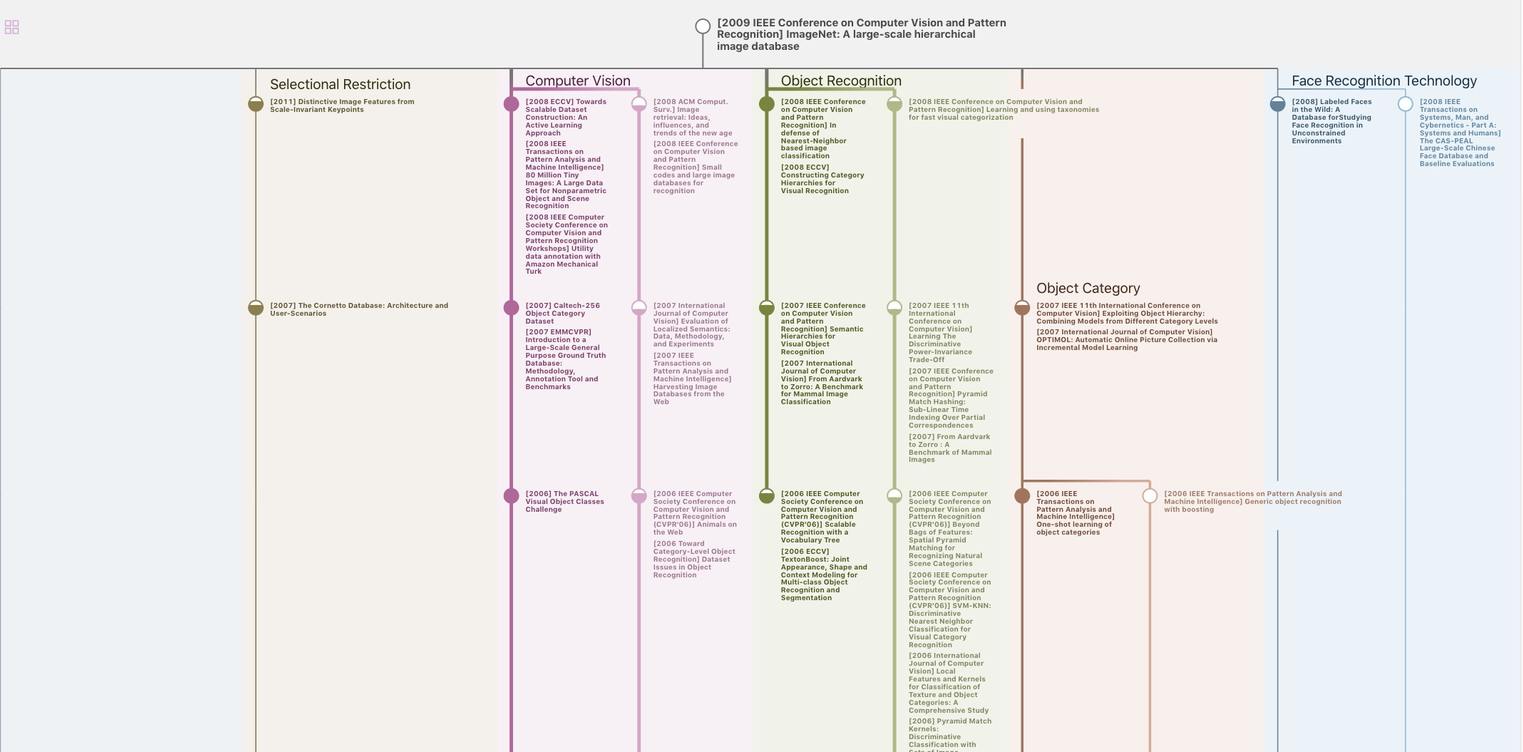
生成溯源树,研究论文发展脉络
Chat Paper
正在生成论文摘要