Mapping Air Quality In Iot Cities: Cloud Calibration And Air Quality Inference Of Sensor Data
2020 IEEE SENSORS(2020)
摘要
Monitoring air quality in cities is challenging as a high resolution in both space and time is required to accurately assess population exposure. This paper presents an innovative IoT approach for highly granular air quality mapping in cities relying on (1) a combination of cloud-calibrated fixed and mobile air quality sensors and (2) machine learning approaches to infer the collected spatiotemporal point measurements in both space and time. Within this work, we focus on validation of this IoT approach by presenting data quality improvements of the cloud calibration algorithm and performance metrics of two spatiotemporal inference models (AVGAE and GRF). The observed cloud calibration improvements and model inference results approaching current physical state-of-the-art models demonstrate the potential of our approach in achieving accurate highly granular air quality maps and ultimately better air quality assessments.
更多查看译文
关键词
IoT, urban, air quality, machine learning, calibration
AI 理解论文
溯源树
样例
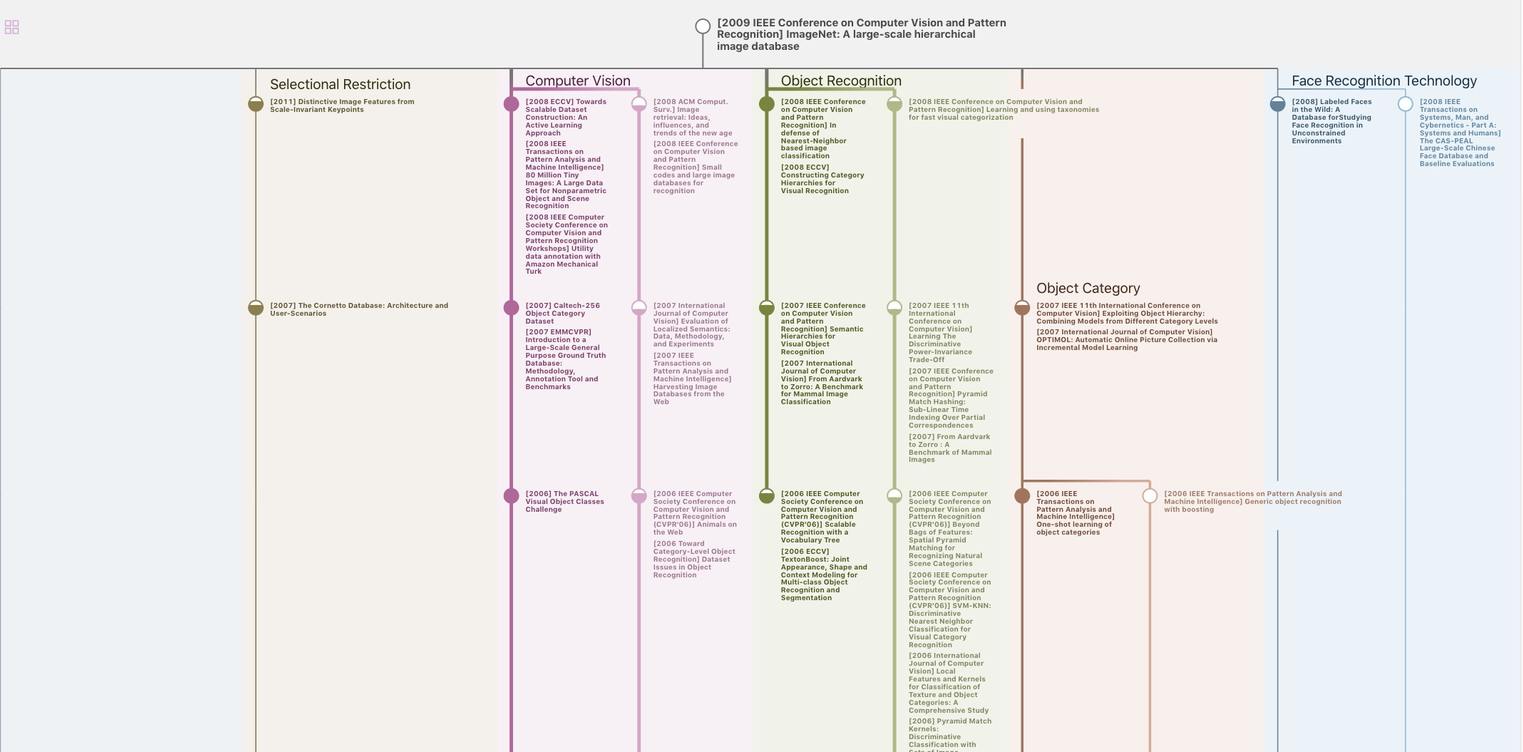
生成溯源树,研究论文发展脉络
Chat Paper
正在生成论文摘要