Machine learning analysis of speech and eye tracking data to distinguish Alzheimer's clinic patients from healthy controls
Alzheimers & Dementia(2020)
摘要
Background Clinical trials of disease‐modifying therapies for Alzheimer’s disease (AD) are increasingly focused on recruiting individuals with preclinical or early‐stage disease. Artificial intelligence may help in enriching clinical trial populations with high‐risk individuals. We analyzed prospectively‐collected speech and eye‐tracking data to distinguish individuals with mild‐moderate AD, mild cognitive impairment (MCI), and subjective memory complaints (SMC) from age and sex‐matched healthy volunteers. Method Individuals with known clinical diagnoses of AD, MCI, and SMC from a specialty clinic, and healthy controls from the community, were prospectively recruited. Participants described the “Cookie Theft Picture” from the Boston Aphasia Battery. Their speech was recorded and manually transcribed and eye movements were assessed using an infrared eye‐tracker. Data underwent feature extraction for language‐related features including lexical and acoustic parameters (from transcripts and speech), and fixation and saccades (from eye‐tracking). Additionally, for language and eye‐tracking, features capturing spatial neglect are explored following the approach in [1]. Separate predictive models were examined using logistic regression (LR), K‐Nearest Neighbours (KNN), and random forests (RF). Result Recruitment and analysis are ongoing. Data from 34 clinic patients (9% SMC, 35% MCI, 56% mild‐moderate AD, mean age 7310, 44% female) and 39 controls (mean age 6811, 79% female) is reported. Best speech‐based models distinguished patients from controls with an Area Under the ROC Curve (AUC) of 79% (95% CI 0.70‐0.87). Eye‐tracking‐based models reached distinguished patients from controls with an AUC of 71% (95% CI 0.59‐0.82). Conclusion Machine‐learning mediated analysis of speech and eye‐tracking data achieved promising classification performance with the current cohort. Both increasing the size of the corpus with ongoing recruitment, and combining demographic and clinical data alongside multimodal feature fusion, may help to improve classification.
更多查看译文
关键词
eye tracking data,machine learning,alzheimer,speech
AI 理解论文
溯源树
样例
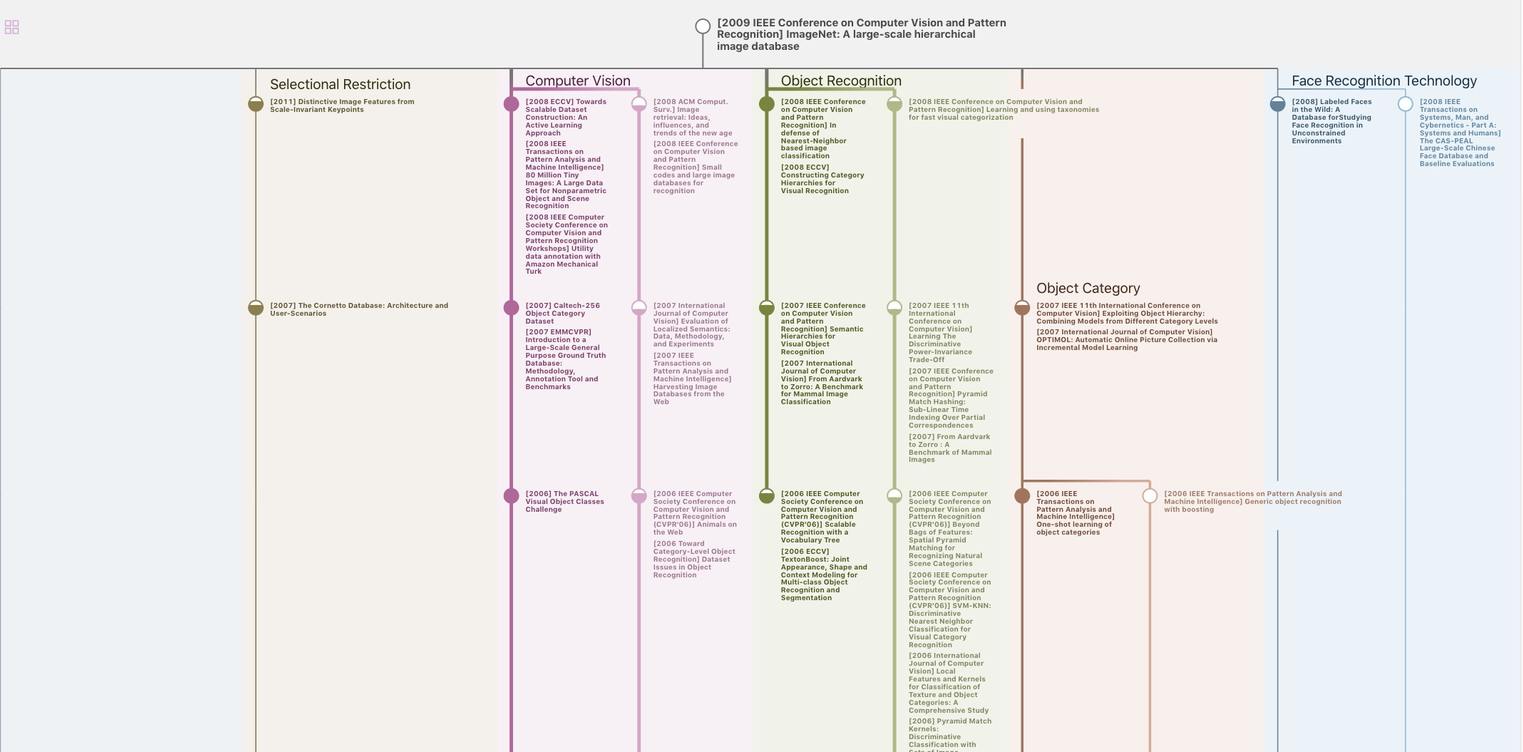
生成溯源树,研究论文发展脉络
Chat Paper
正在生成论文摘要