Variational Autoencoder As a Generative Tool to Produce De-Novo Lead Compounds for Biological Targets
2020 14th International Conference on Innovations in Information Technology (IIT)(2020)
摘要
A variational autoencoder (VAE) is a generational deep learning model based on encoding a particular observation into a latent space and then decoding it while incorporating some random noise with the intuition of being able to generate slightly different forms of the input observation. Here, I propose a VAE that is able to generate denovo drug compounds by feeding in a known set of drug compounds against a particular biological target. To demonstrate the ability of the proposed VAE as a generative model, known drug molecules belonging to the class of Neuraminidase (NA) inhibitors were taken from the ZINC database and fed into the VAE model as one-hot encoded SMILES strings. Similarly, active NA inhibitors and decoy molecules together were also fed to compare efficiency. The generated molecules were then screened to remove impractical structures. Next, a drug-likeness (QED) score was computed for each candidate molecule and a cutoff of 0.5 was used to extract viable candidates. To ensure that the generated drug compounds were active NA inhibitors, a series of Artificial Neural Networks (ANNs) classifiers based on three different characterization techniques, namely chemical fingerprinting, molecular descriptions and graph convolutions, were developed to identify active NA inhibitors from decoy molecules. The feature candidate data were then fed into the three designed ANNs to obtain the final set of novel, viable and active NA inhibitors. Seventy-one new NA inhibitors were obtained after three runs of the VAE model under different parameterizations. The proposed VAE can hence be used to generate de-novo drug compounds for a wide variety of biological targets.
更多查看译文
关键词
Variational Autoencoder,ANNs,ZINC Database,SMILES strings,QED score,Neuraminidase
AI 理解论文
溯源树
样例
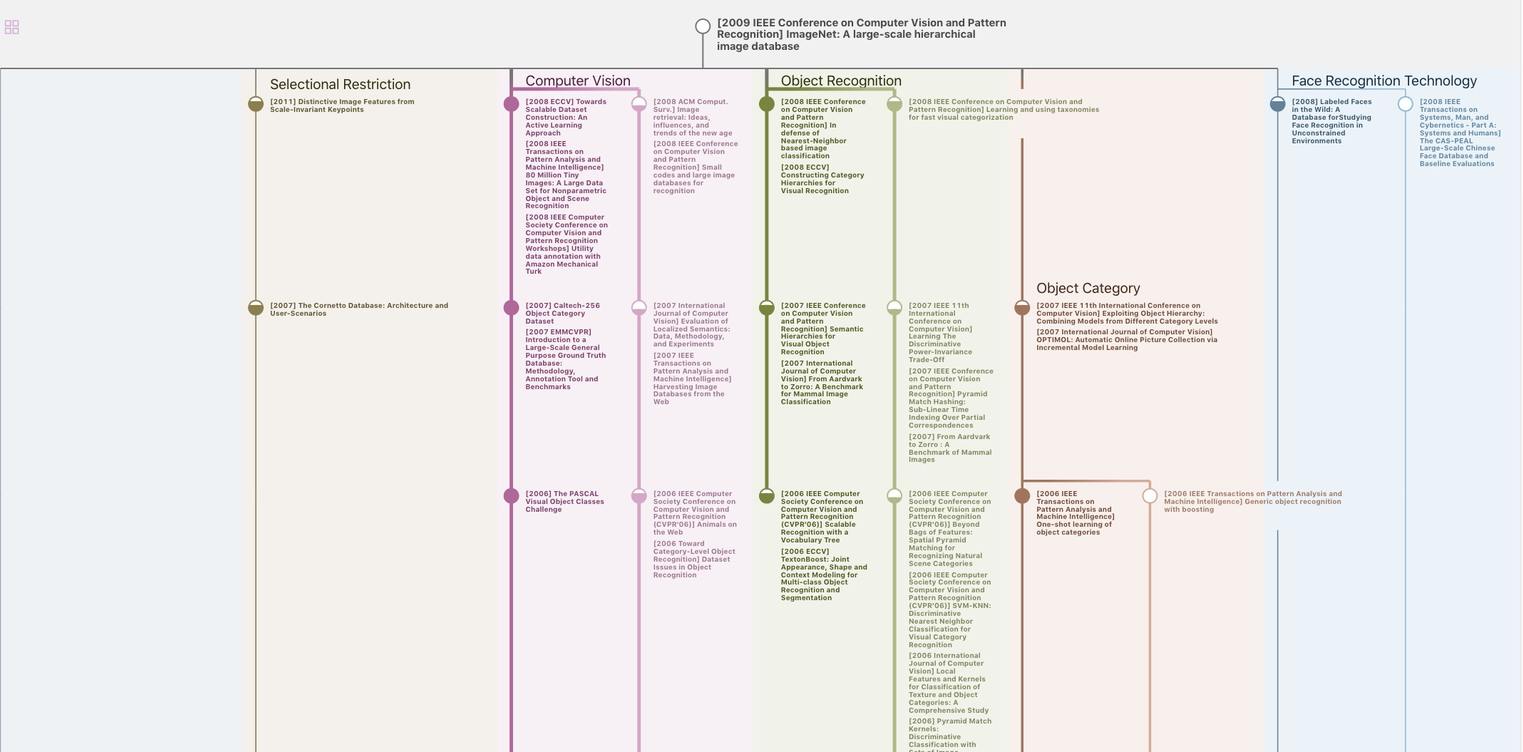
生成溯源树,研究论文发展脉络
Chat Paper
正在生成论文摘要