L1-norm Unsupervised Fukunaga-Koontz Transform
Signal Processing(2021)
摘要
The Fukunaga-Koontz transform (FKT) is a powerful supervised feature extraction method used in two class recognition problems, particularly when the classes have equal mean vectors but different covariance matrices. The present work proves that it is also possible to perform the FKT in an unsupervised manner, sparing the need for labeled data, by using a variant of L1-norm Principal Component Analysis (L1-PCA) that minimizes the L1-norm in the feature space. Rigorous proof is given in the case of data drawn from a mixture of Gaussians. A working iterative algorithm based on gradient-descent in the Stiefel manifold is put forward to perform L1-norm minimization with orthogonal constraints. A number of numerical experiments on synthetic and real data confirm the theoretical findings and the good convergence characteristics of the proposed algorithm. (c) 2021 The Authors. Published by Elsevier B.V. This is an open access article under the CC BY-NC-ND license ( http://creativecommons.org/licenses/by-nc-nd/4.0/ )
更多查看译文
关键词
Fukunaga-Koontz,Common spatial patterns,Tuned-based functions,L1-PCA
AI 理解论文
溯源树
样例
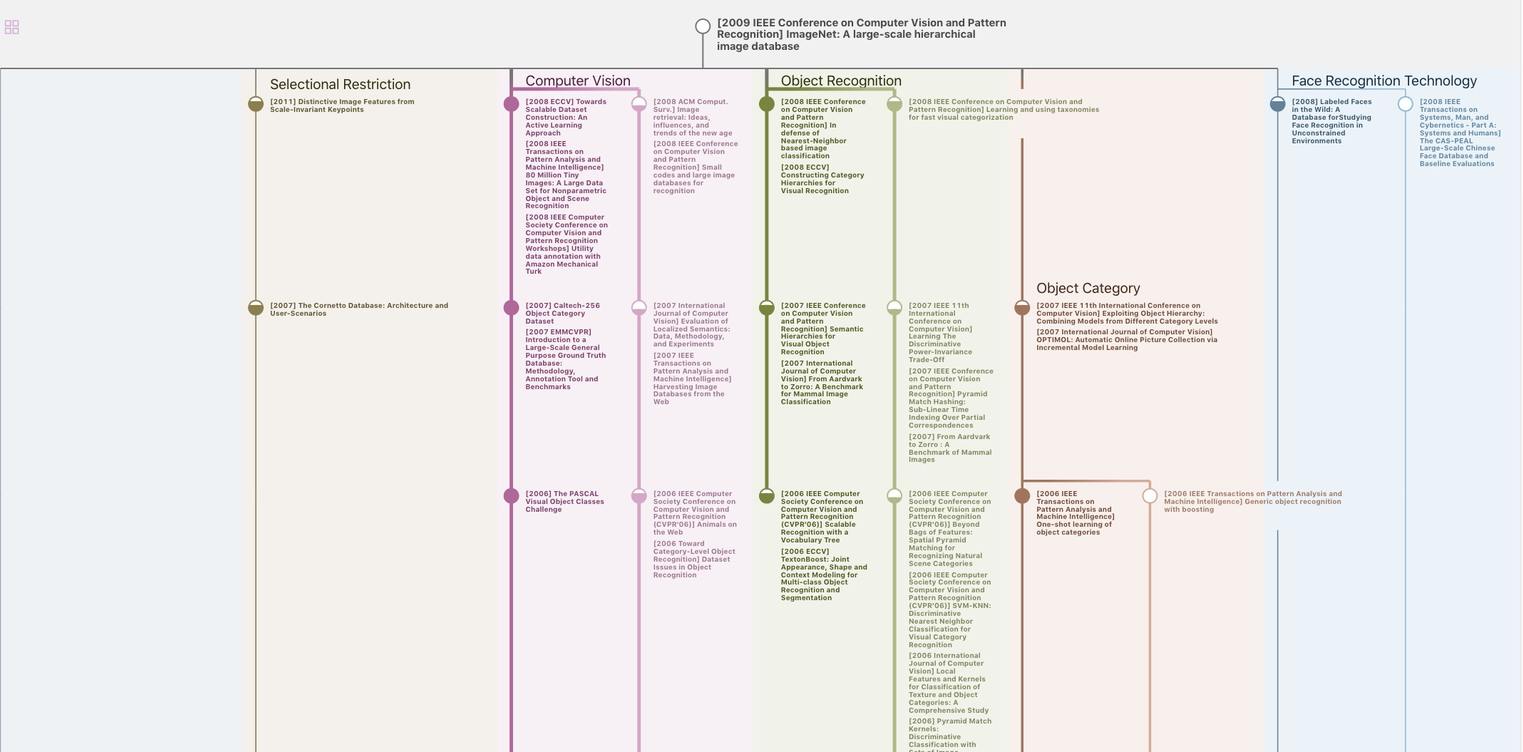
生成溯源树,研究论文发展脉络
Chat Paper
正在生成论文摘要