Warpage prediction of Injection-molded PVC part using ensemble machine learning algorithm
MATERIALS TODAY-PROCEEDINGS(2022)
摘要
In this study, the warpage prediction models have been developed for an injection molded PVC component named a drip chamber. Two popular and widely used ensemble machine learning algorithms, namely random forest and gradient boosted regression tree have been used to develop the predictive models. 40 experiments were carried out for various input process parameters to develop the warpage prediction dataset. ANOVA was performed to identify the significant input process parameters. These process parameters were barrel temperature, holding pressure, holding time, mold temperature, and cooling time. The results shows that the mean absolute percentage errors of random forest and gradient boosted regression tree model are 3.25% and 9.37%, respectively. This indicates that random forest ensemble algorithm outperforms gradient boosted model in predicting the warpage of injection molded part. This model allows production managers to monitor injection molding method parameters and regulate the warpage before actual production and with minimum waste. (c) 2020 Elsevier Ltd. All rights reserved. Selection and peer-review under responsibility of the scientific committee of the 2nd International Conference on Functional Material, Manufacturing and Performances.
更多查看译文
关键词
PVC,Warpage prediction,Injection-molding,Ensemble machine learning algorithms
AI 理解论文
溯源树
样例
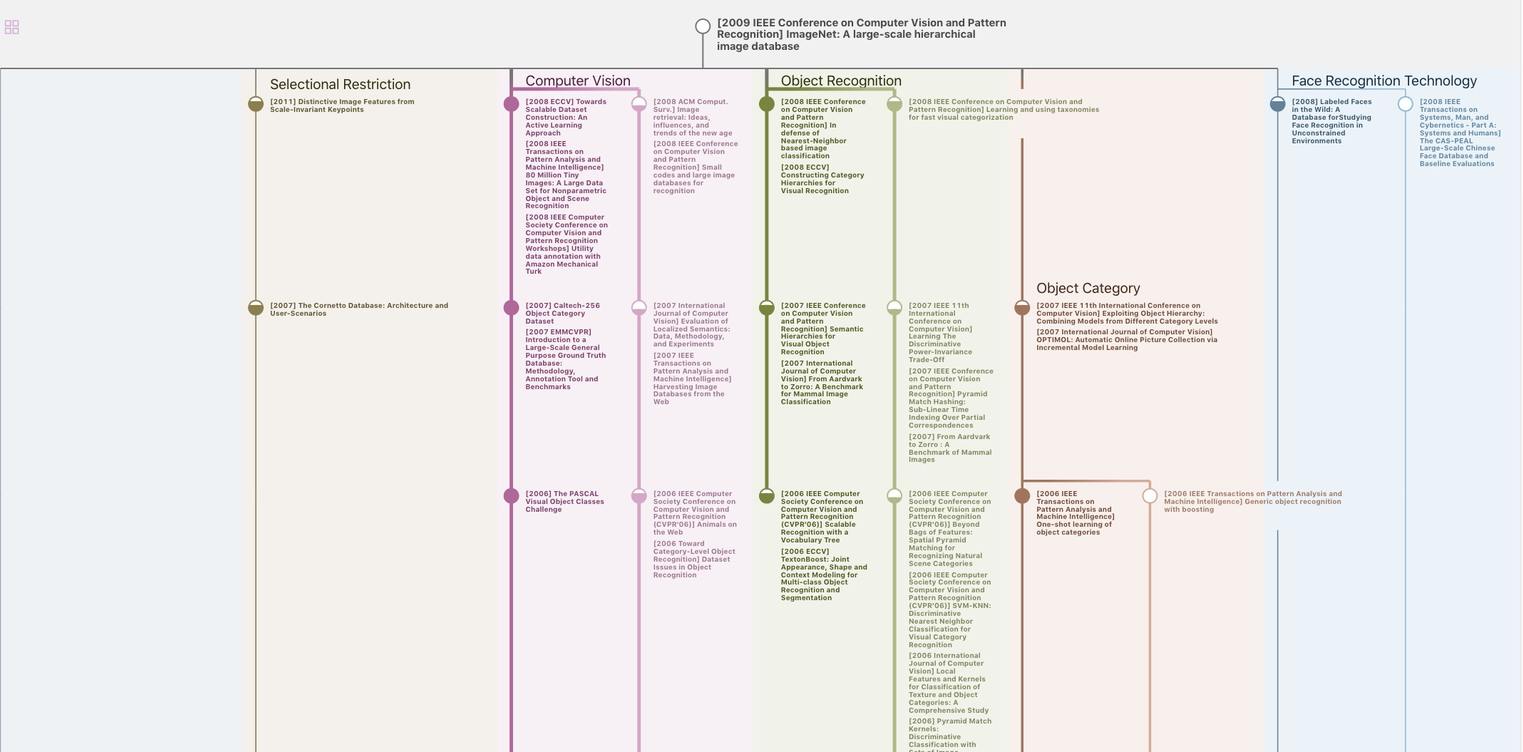
生成溯源树,研究论文发展脉络
Chat Paper
正在生成论文摘要