Random Forest And Autoencoder Data-Driven Models For Prediction Of Dispersed-Phase Holdup And Drop Size In Rotating Disc Contactors
INDUSTRIAL & ENGINEERING CHEMISTRY RESEARCH(2021)
摘要
Linear regression models are traditionally used to capture the relation between the input and output variables. Linear models cannot account for the nonlinear relations in the data. Hence, the prediction models may not be accurate. For this reason, machine learning-based models are being increasingly used. For modeling, design, and scale-up of rotating disc contactors (RDCs), rational estimation of dispersed-phase holdup and drop size is crucial. We have employed random forest (RF) and autoencoder-RF-based models for the prediction of dispersed-phase holdup and drop size in RDCs. Our results show that both these models predict drop size quite well. For holdup, the autoencoder-RF combination predictions are not satisfactory. The standalone RF model predictions generalize very well. RF-based models can be further used for prediction of different variables of interest in RDCs.
更多查看译文
AI 理解论文
溯源树
样例
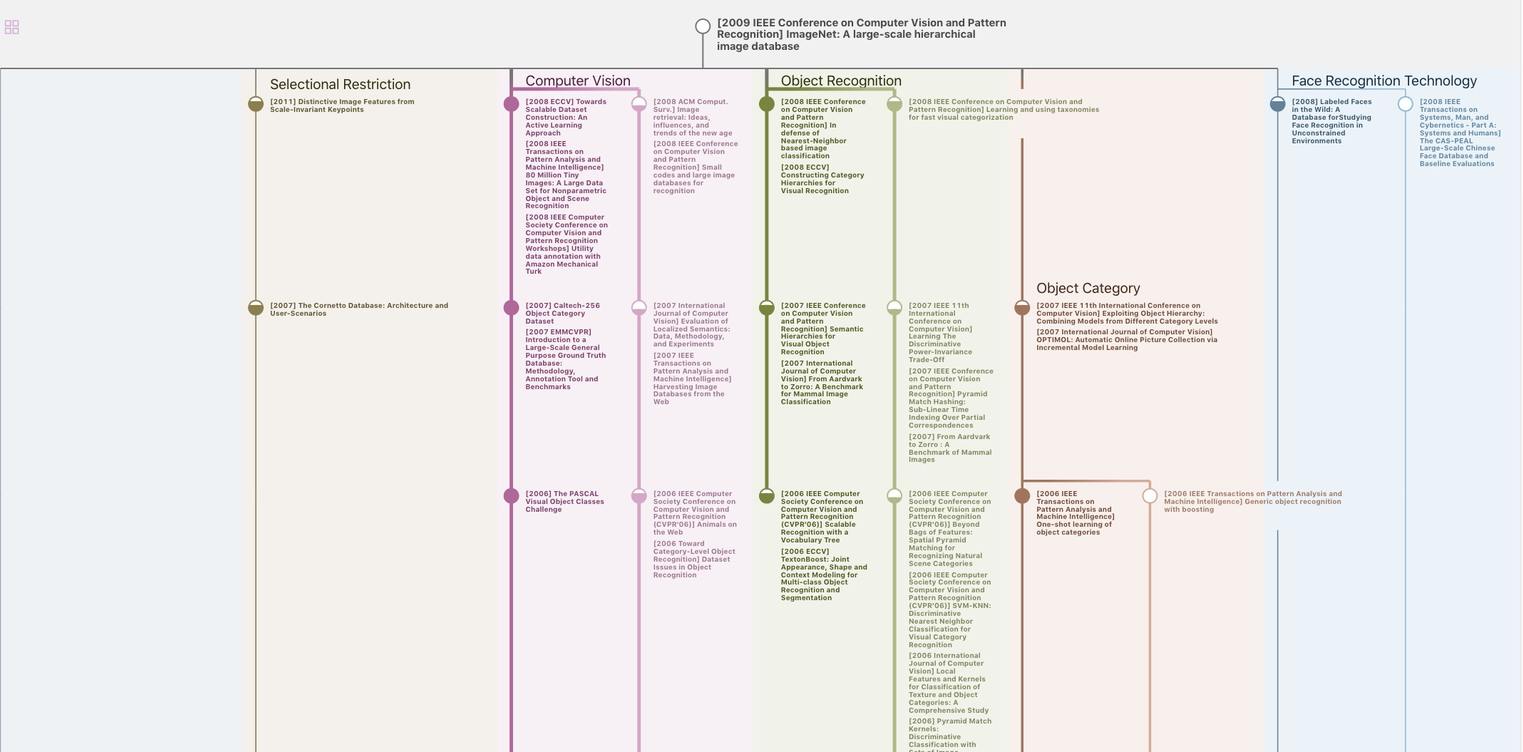
生成溯源树,研究论文发展脉络
Chat Paper
正在生成论文摘要