Deep Reinforcement Learning with Experience Sharing for Power Control
2020 IEEE 20th International Conference on Communication Technology (ICCT)(2020)
摘要
Since wireless communication channels are susceptible to be blocked by interference, it is important to allocate appropriate power to the channels. This paper focuses on power control problem for users in multiple sweep interferences scenario. Without being aware of interference model, deep reinforcement learning algorithm for power control is proposed to maximize the reward performance. Neural networks are applied to approximate Q-table in deep Q-network (DQN) algorithm, action selection and value function in deep deterministic policy gradient (DDPG) algorithm. We propose experience sharing (ES) approach that store experience from all channels with the same probability distribution to accelerate training process and improve converge performance. We have implemented the proposed algorithm based on TensorFlow. The simulation results show experience sharing DDPG (ES-DDPG) algorithm converges to optimal policy, and achieves better reward performance and converge performance than the algorithm without experience sharing.
更多查看译文
关键词
power control,multiple sweep interferences,deep Q-network,deep deterministic policy gradient,experience sharing
AI 理解论文
溯源树
样例
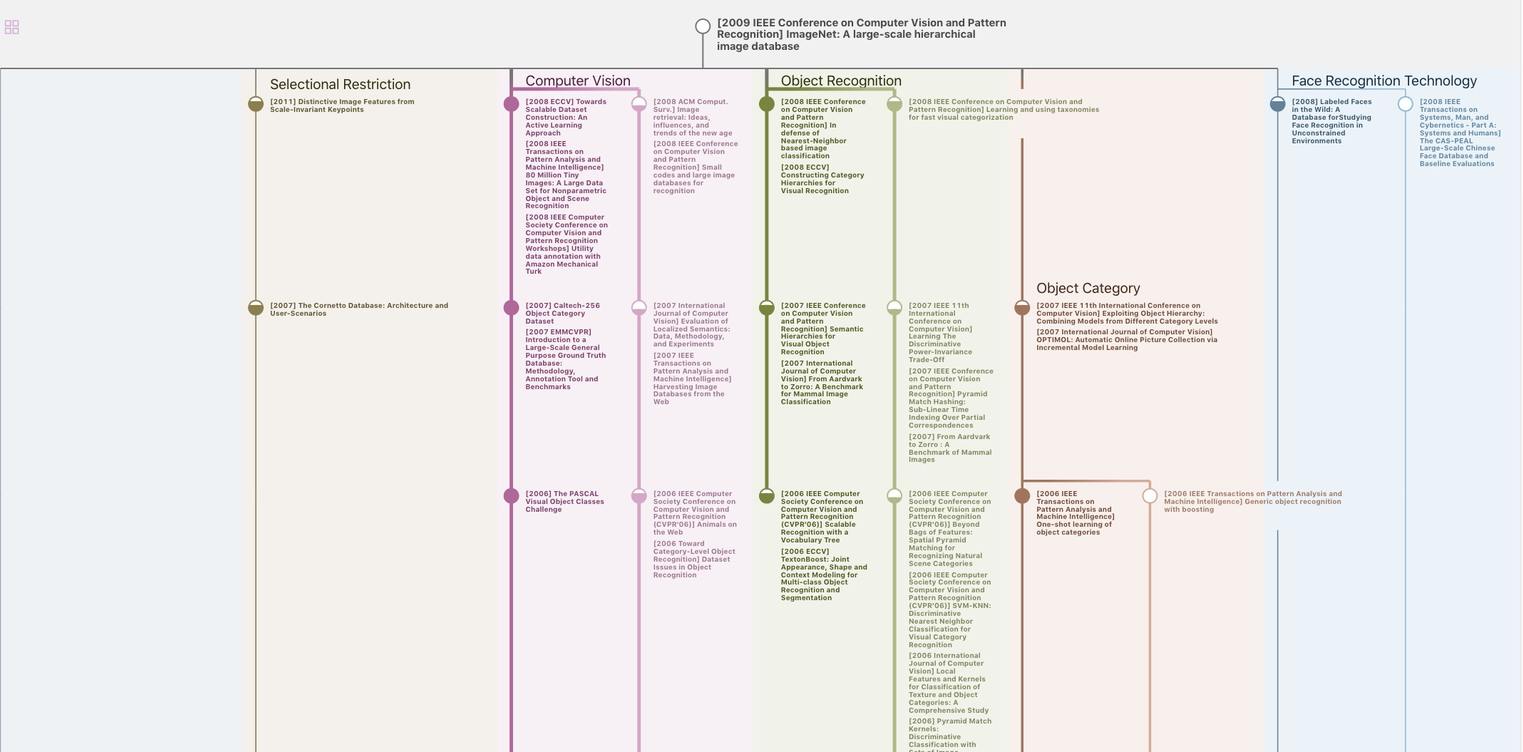
生成溯源树,研究论文发展脉络
Chat Paper
正在生成论文摘要