Network Update Compression for Federated Learning
VCIP(2020)
摘要
In federated learning setting, models are trained in a variety of edge-devices with locally generated data and each round only updates in the current model rather than the model itself are sent to the server where they are aggregated to compose an improved model. These edge devices, however, reside in highly uneven nature of network with higher latency and lower-throughput connections and are intermittently available for training. In addition, a network connection has an asymmetric nature of downlink and uplink. All these contribute to a major challenge while synchronizing these updates to the server.In this work, we proposed an efficient coding solution to significantly reduce uplink communication cost by reducing the total number of parameters required for updates. This was achieved by applying Gaussian Mixture Model (GMM) to localize Karhunen-Loève Transform (KLT) on inter-model subspace and representing it with two low-rank matrices. Experiments on convolutional neural network (CNN) models showed the proposed model can significantly reduce the uplink communication cost in federated learning while preserving reasonable accuracy.
更多查看译文
关键词
federated learning,network-update compression,Karhunen–Loève Transform (KLT)
AI 理解论文
溯源树
样例
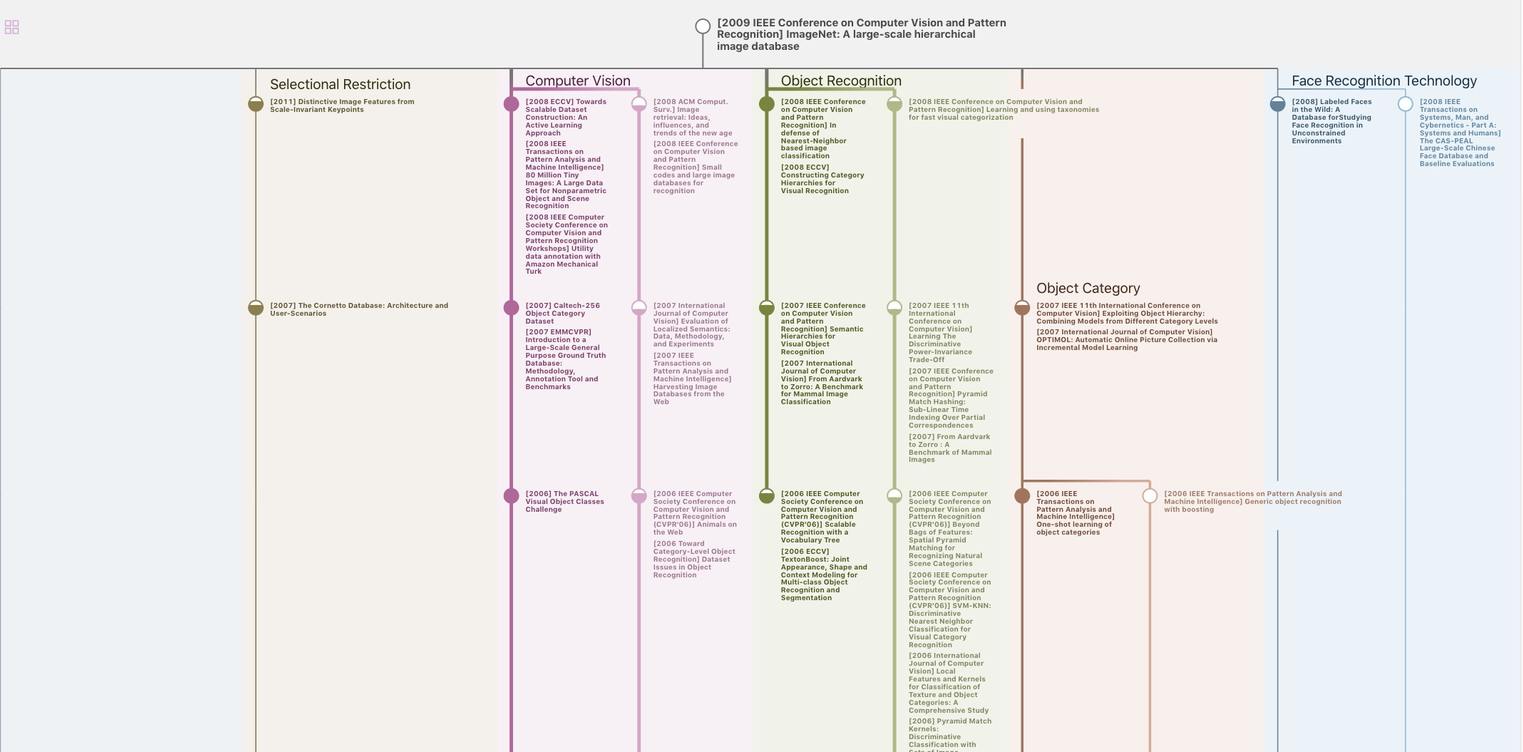
生成溯源树,研究论文发展脉络
Chat Paper
正在生成论文摘要