Reinforcement Learning based Real Time Aerial BS Positioning for Dense Urban 5G Mobile Network
ICTC(2020)
Abstract
Due to the recent surge in mobile users and traffic, next-generation mobile communication aims to increase network capacity through large bandwidth and small cell deployment. To respond to this situation, there have been lots of researches on aerial base stations (BSs) using unmanned aerial vehicles (UAVs) for the mobile user. Aerial BS has the advantage of providing flexible communication range by avoiding obstacles such as buildings in urban areas through 3D positioning. However, finding an optimal point for aerial BS considering the variance of user's requirements, movement, and obstacles in real time is difficult problem to solve. Therefore, it is necessary to find an approximate optimal point for applying to the real-time flight path control of aerial BS. This paper aims to find optimal behavior in real time interacting with a given environment, through reinforcement learning. We propose the algorithm based on Q-learning with a new concept called Coarse Search to reduce convergence speed. We evaluate the performance of the algorithm by comparing it with that of other heuristic algorithms.
MoreTranslated text
Key words
flexible communication range,urban areas,approximate optimal point,real-time flight path control,time interacting,reinforcement learning,time aerial BS positioning,dense urban 5g mobile network,mobile users,traffic,next-generation mobile communication,network capacity,aerial base stations,unmanned aerial vehicles,mobile user
AI Read Science
Must-Reading Tree
Example
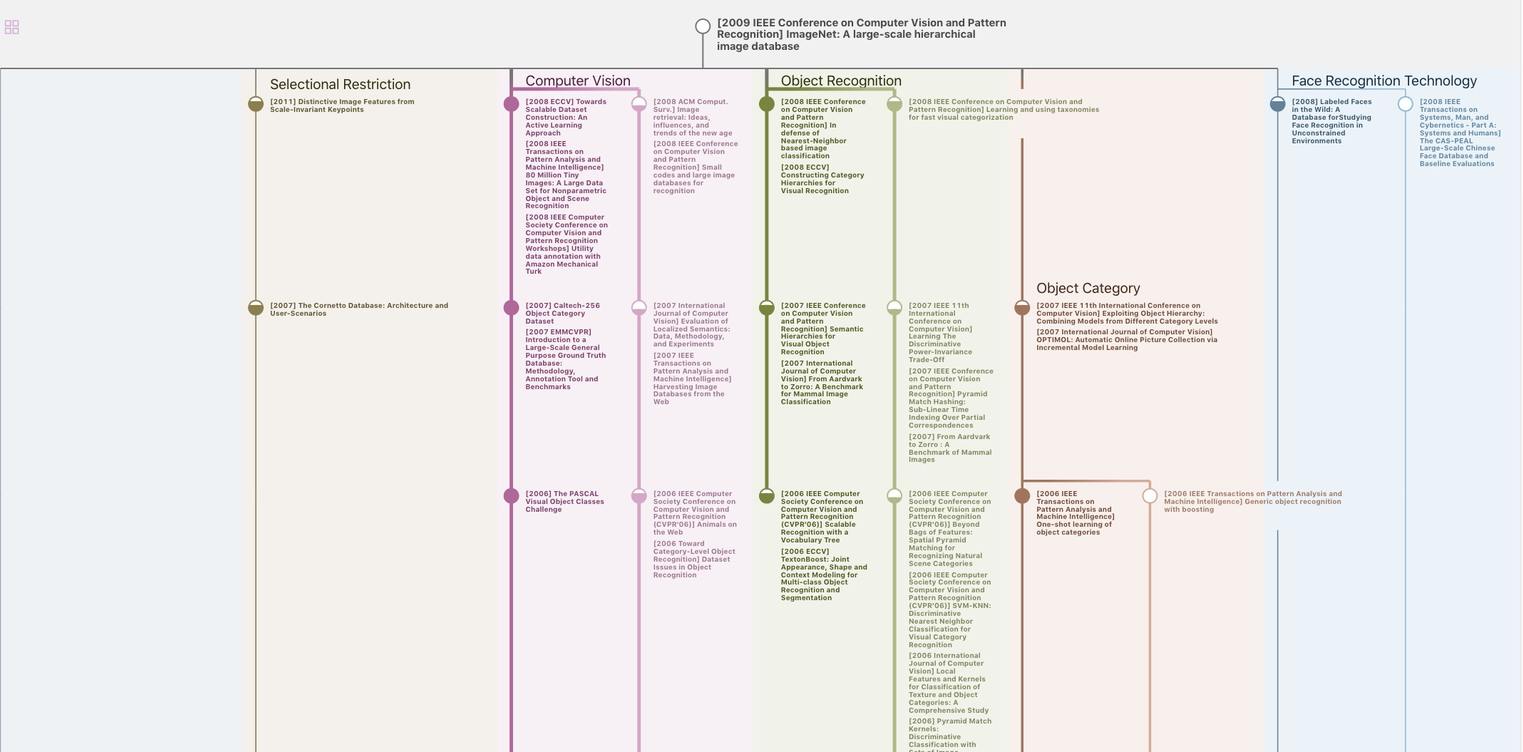
Generate MRT to find the research sequence of this paper
Chat Paper
Summary is being generated by the instructions you defined