Efficient Similarity-Aware Influence Maximization in Geo-Social Network
IEEE Transactions on Knowledge and Data Engineering(2022)
摘要
With the explosion of GPS-enabled smartphones and social media platforms, geo-social networks are increasing as tools for businesses to promote their products or services. Influence maximization, which aims to maximize the expected spread of influence in the networks, has drawn increasing attention. However, most recent work tries to study influence maximization by only considering geographic distance, while ignoring the influence of users’ spatio-temporal behavior on information propagation or location promotion, which can often lead to poor results. To relieve this problem, we propose a Similarity-aware Influence Maximization (SIM) model to efficiently maximize the influence spread by taking the effect of users’ spatio-temporal behavior into account, which is more reasonable to describe the real information propagation. We first calculate the similarity between users according to their historical check-ins, and then we propose a Propagation to Consumption (PTC) model to capture both online and offline behaviors of users. Finally, we propose two greedy algorithms to efficiently maximize the influence spread. The extensive experiments over real datasets demonstrate the efficiency and effectiveness of the proposed algorithms.
更多查看译文
关键词
Geo-social networks,influence maximization,similarity-aware
AI 理解论文
溯源树
样例
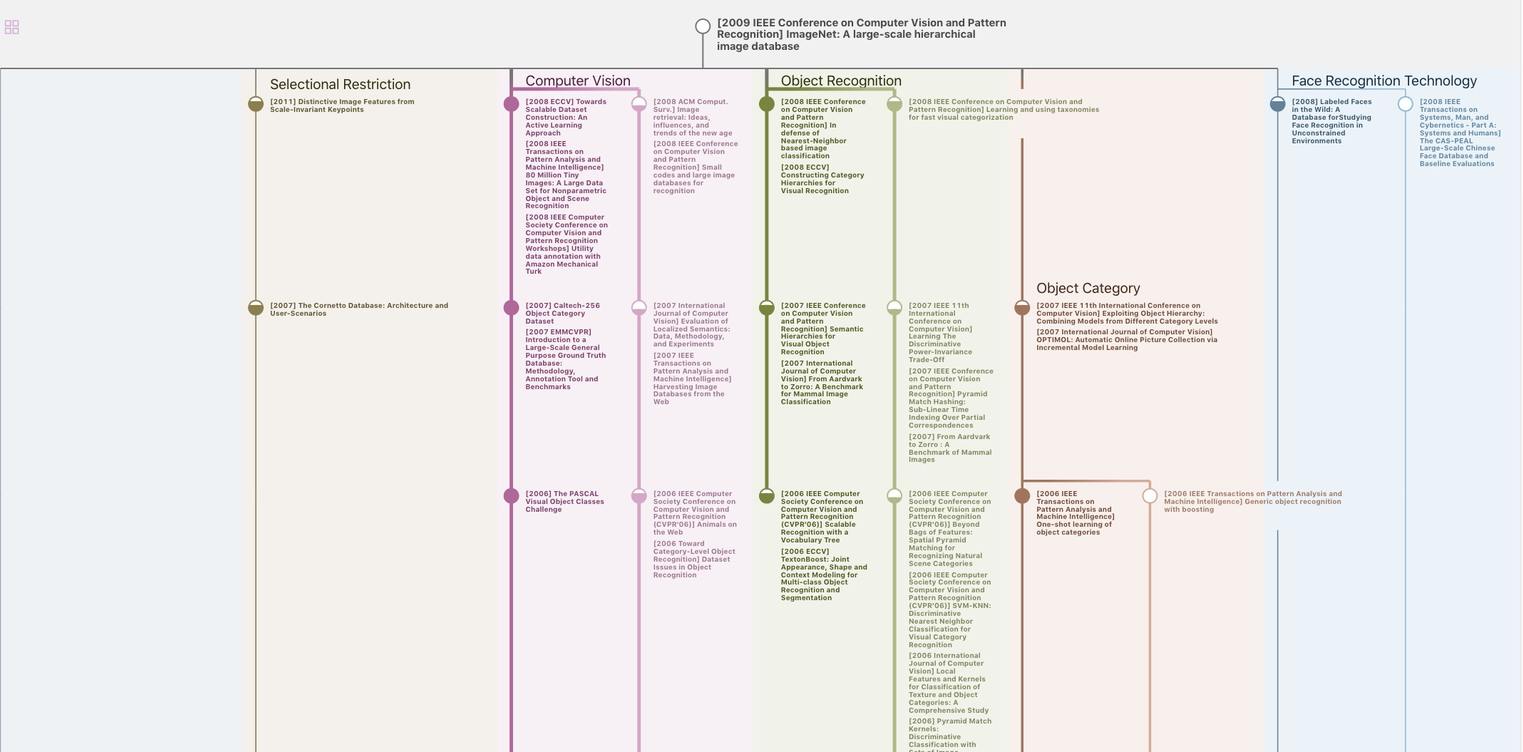
生成溯源树,研究论文发展脉络
Chat Paper
正在生成论文摘要