Unsupervised Attributed Network Embedding via Cross Fusion
WSDM(2021)
摘要
ABSTRACTAttributed network embedding aims to learn low dimensional node representations by combining both the network's topological structure and node attributes. Most of the existing methods either propagate the attributes over the network structure or learn the node representations by an encoder-decoder framework. However, propagation based methods tend to prefer network structure to node attributes, whereas encoder-decoder methods tend to ignore the longer connections beyond the immediate neighbors. In order to address these limitations while enjoying the best of the two worlds, we design cross fusion layers for unsupervised attributed network embedding. Specifically, we first construct two separate views to handle network structure and node attributes, and then design cross fusion layers to allow flexible information exchange and integration between the two views. The key design goals of the cross fusion layers are three-fold: 1) allowing critical information to be propagated along the network structure, 2) encoding the heterogeneity in the local neighborhood of each node during propagation, and 3) incorporating an additional node attribute channel so that the attribute information will not be overshadowed by the structure view. Extensive experiments on three datasets and three downstream tasks demonstrate the effectiveness of the proposed method.
更多查看译文
关键词
Network embedding,network structure,local community,node attributes
AI 理解论文
溯源树
样例
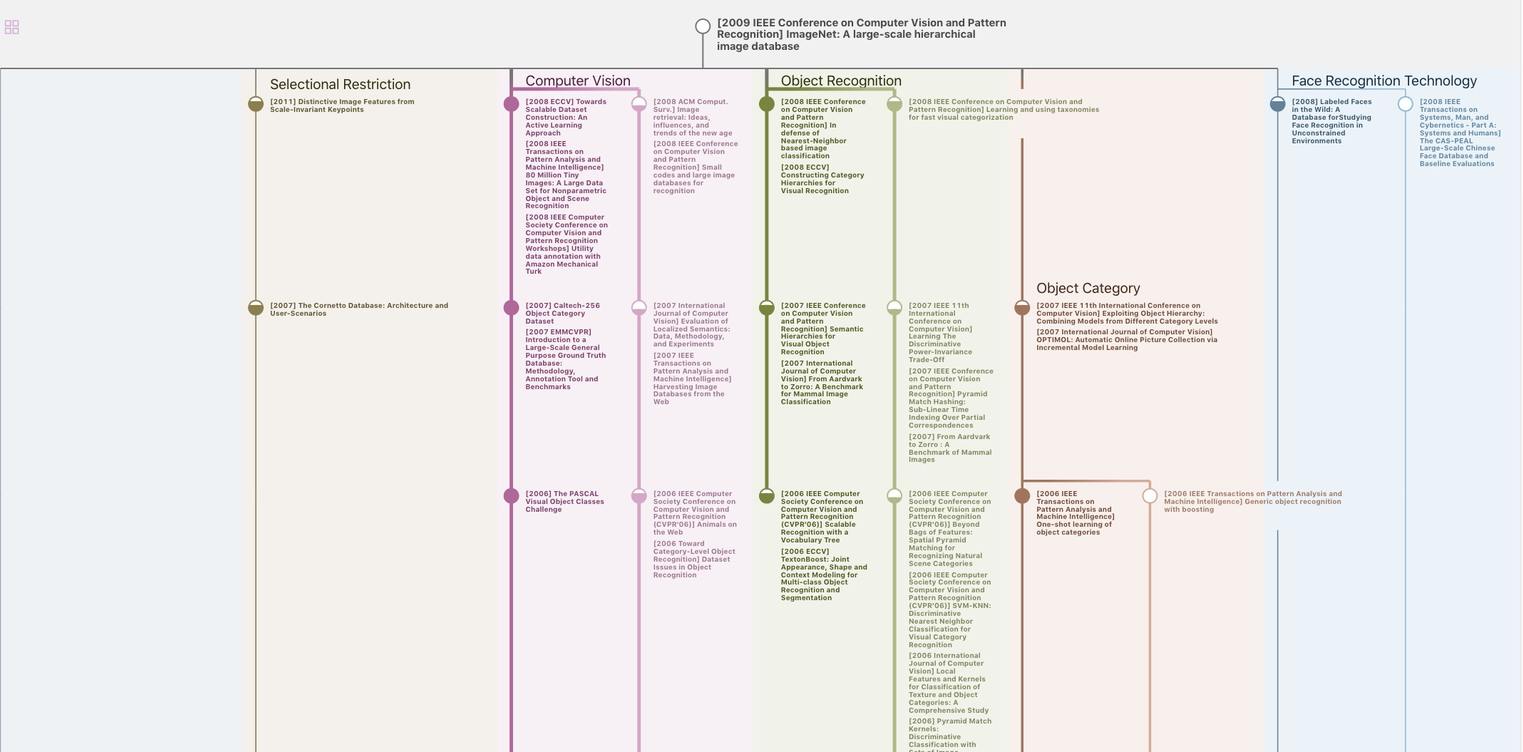
生成溯源树,研究论文发展脉络
Chat Paper
正在生成论文摘要