Unbiased Learning to Rank in Feeds Recommendation
WSDM(2021)
摘要
ABSTRACTIn feeds recommendation, users are able to constantly browse items generated by never-ending feeds using mobile phones. The implicit feedback from users is an important resource for learning to rank, however, building ranking functions from such observed data is recognized to be biased. The presentation of the items will influence the user's judgements and therefore introduces biases. Most previous works in the unbiased learning to rank literature focus on position bias (i.e., an item ranked higher has more chances of being examined and interacted with). By analyzing user behaviors in product feeds recommendation, in this paper, we identify and introduce context bias, which refers to the probability that a user interacting with an item is biased by its surroundings, to unbiased learning to rank. We propose an Unbiased Learning to Rank with Combinational Propensity (ULTR-CP) framework to remove the inherent biases jointly caused by multiple factors. Under this framework, a context-aware position bias model is instantiated to estimate the unified bias considering both position and context biases. In addition to evaluating propensity score estimation approaches by the ranking metrics, we also discuss the evaluation of the propensities directly by checking their balancing properties. Extensive experiments performed on a real e-commerce data set collected from JD.com verify the effectiveness of context bias and illustrate the superiority of ULTR-CP against the state-of-the-art methods.
更多查看译文
关键词
Feeds Recommendation,Learning to Rank,Unbiased Learning
AI 理解论文
溯源树
样例
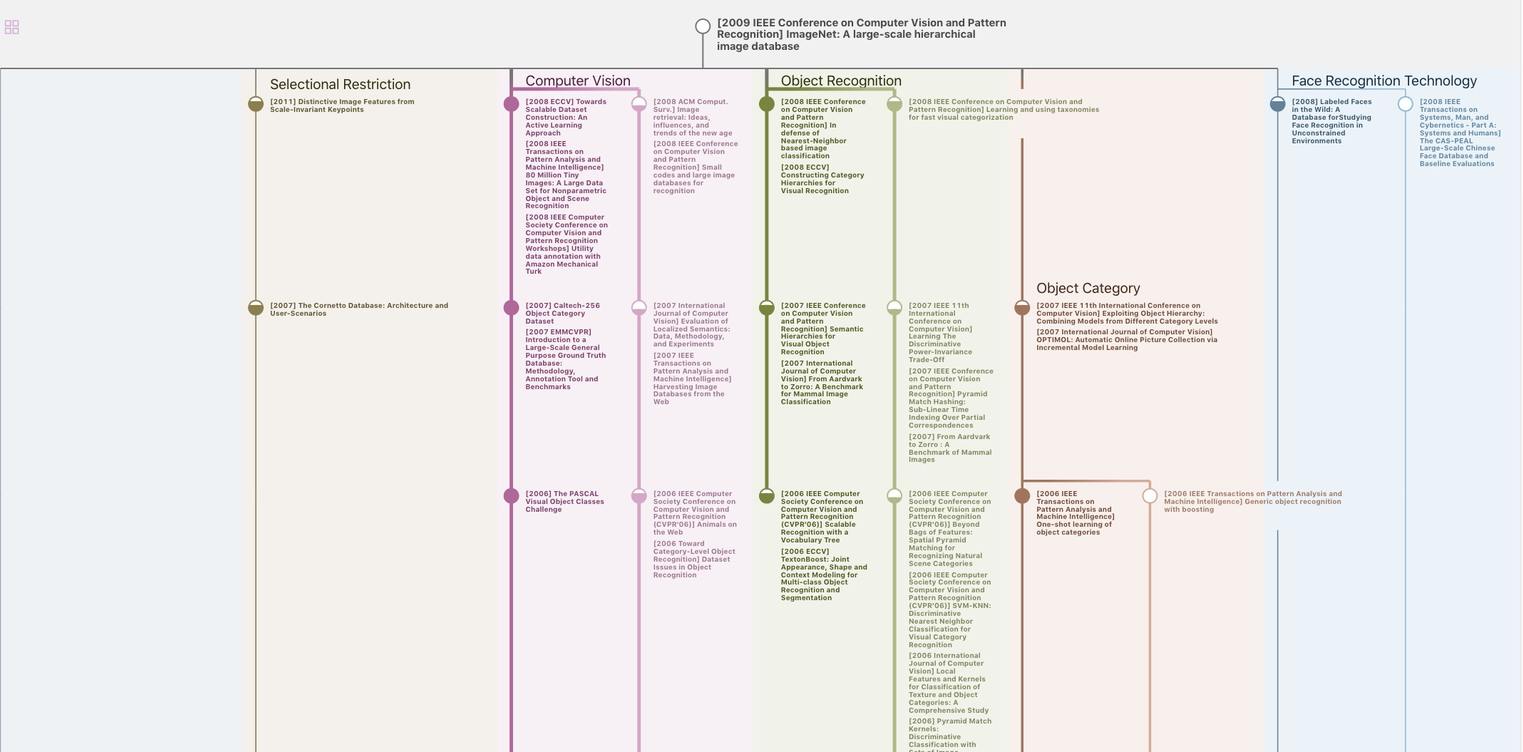
生成溯源树,研究论文发展脉络
Chat Paper
正在生成论文摘要