Show, Attend to Everything, and Tell: Image Captioning with More Thorough Image Understanding
2020 10th International Conference on Computer and Knowledge Engineering (ICCKE)(2020)
摘要
Image captioning is one of the most important cross-modal tasks in machine learning. Attention-based encoder-decoder frameworks have been utilized for this task, abundantly. For visual understanding of an image, via the encoder, most of these networks use the last convolutional layer of a network designed for some computer vision tasks. There are several downsides to that. First, these models are specialized to detect certain objects from the image. Thus, when we get deeper into the network, the network focuses on these objects, becoming almost blind to the rest of the image. These blindspots of the encoder sometimes are where the next word in the caption lies. Moreover, many words in the caption are not included in the target classes of these tasks, such as "snow". having this observation in mind, in order to reduce the blind spots of the last convolutional layer of the encoder, we propose a novel method to reuse other convolutional layers of the encoder. Doing so provides us diverse features of the image while not neglecting almost any part of the image and hence, we "attend to everything" in the image. Using the flickr30k [1] dataset, we evaluate our method and demonstrate comparable results with the state-of-the-art, even with simple attention mechanisms.
更多查看译文
关键词
image captioning,image understanding,cross-modal tasks,machine learning,attention-based encoder-decoder,visual understanding,convolutional layer,computer vision,blind spot reduction,flickr30k dataset,object detection
AI 理解论文
溯源树
样例
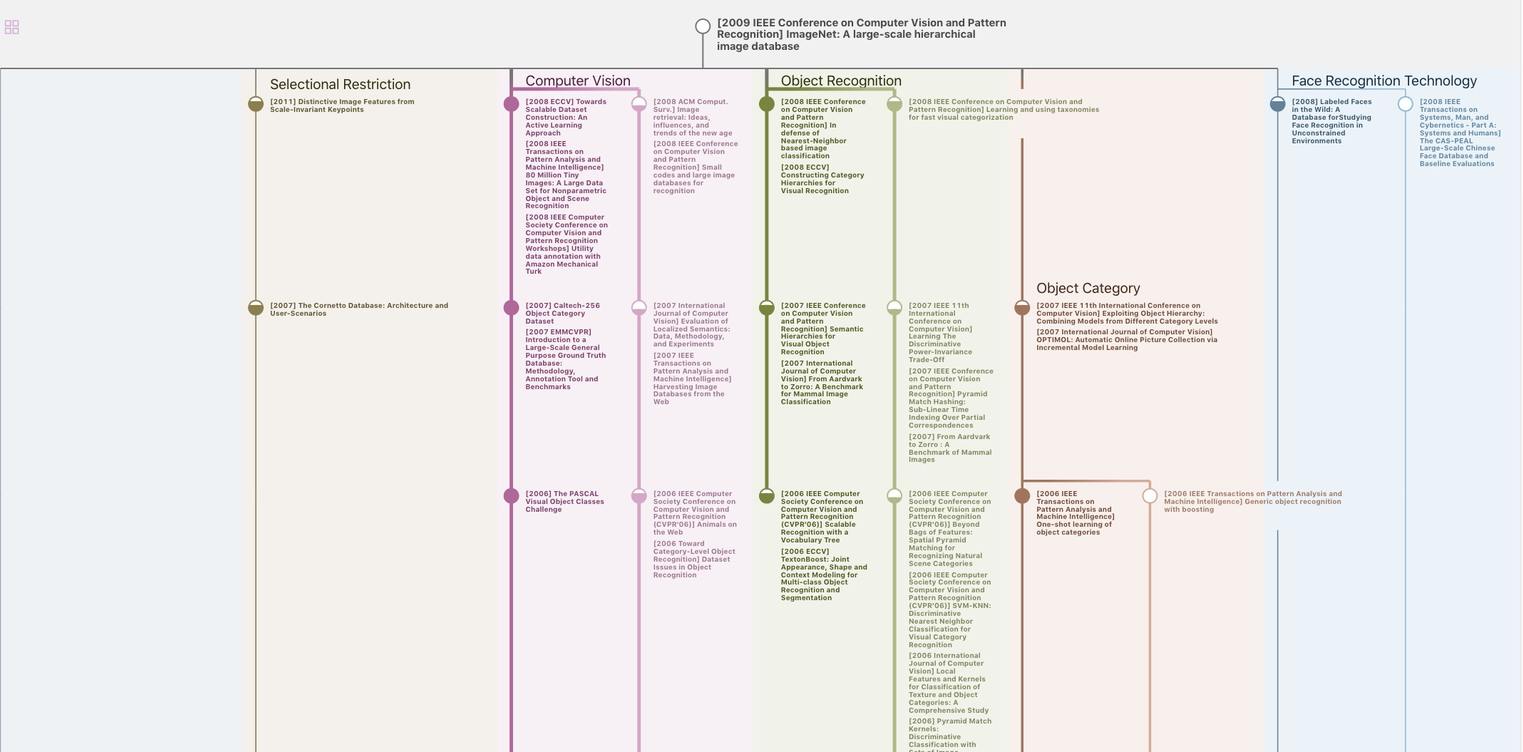
生成溯源树,研究论文发展脉络
Chat Paper
正在生成论文摘要