Predicting Crowd Flows via Pyramid Dilated Deeper Spatial-temporal Network
WSDM(2021)
摘要
ABSTRACTPredicting crowd flows is crucial for urban planning, traffic management and public safety. However, predicting crowd flows is not trivial because of three challenges: 1) highly heterogeneous mobility data collected by various services; 2) complex spatio-temporal correlations of crowd flows, including multi-scale spatial correlations along with non-linear temporal correlations. 3) diversity in long-term temporal patterns. To tackle these challenges, we proposed an end-to-end architecture, called pyramid dilated spatial-temporal network (PDSTN), to effectively learn spatial-temporal representations of crowd flows with a novel attention mechanism. Specifically, PDSTN employs the ConvLSTM structure to identify complex features that capture spatial-temporal correlations simultaneously, and then stacks multiple ConvLSTM units for deeper feature extraction. For further improving the spatial learning ability, a pyramid dilated residual network is introduced by adopting several dilated residual ConvLSTM networks to extract multi-scale spatial information. In addition, a novel attention mechanism, which considers both long-term periodicity and the shift in periodicity, is designed to study diverse temporal patterns. Extensive experiments were conducted on three highly heterogeneous real-world mobility datasets to illustrate the effectiveness of PDSTN beyond the state-of-the-art methods. Moreover, PDSTN provides intuitive interpretation into the prediction.
更多查看译文
关键词
Mobility Data,Crowd Flow Prediction,Spatial-Temporal Modeling,Attention Mechanism
AI 理解论文
溯源树
样例
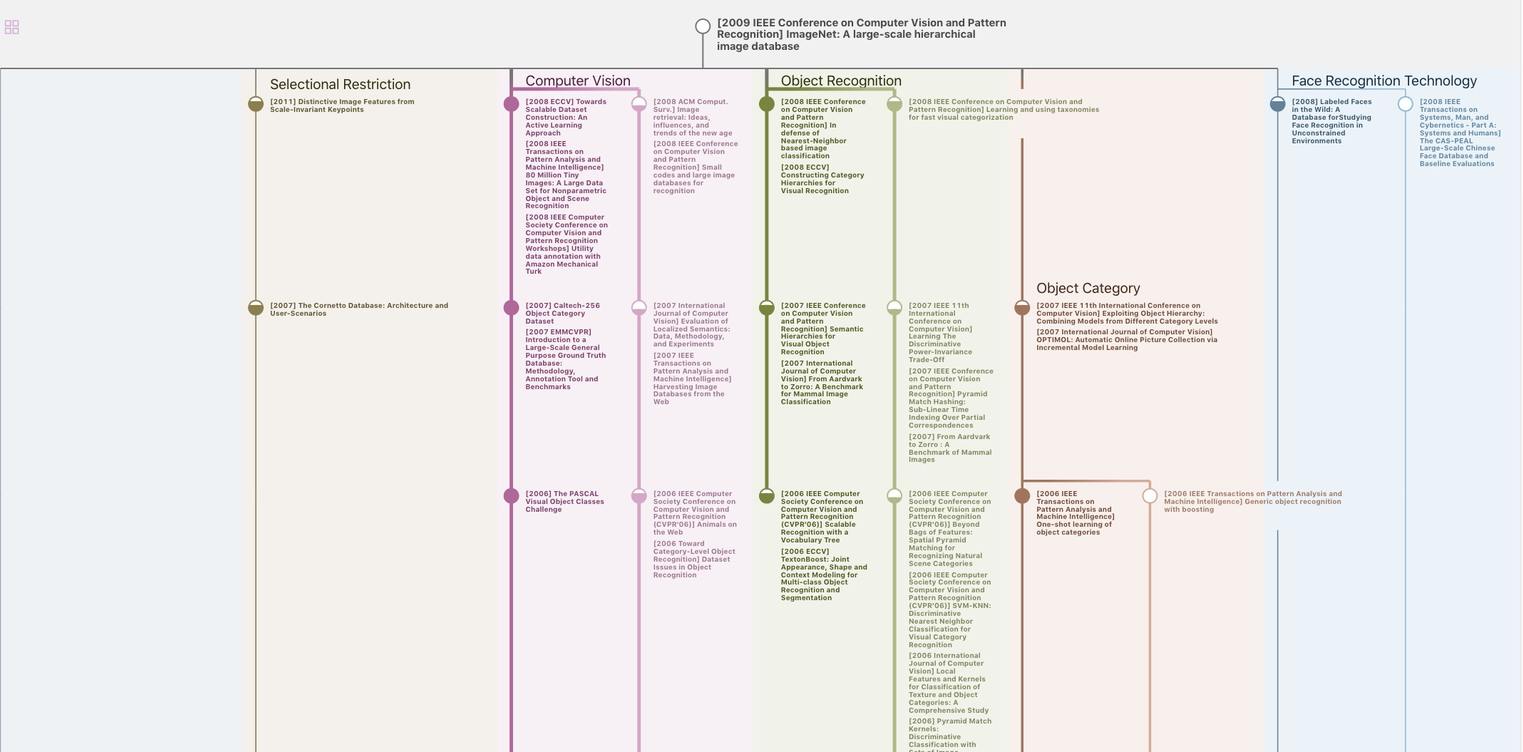
生成溯源树,研究论文发展脉络
Chat Paper
正在生成论文摘要