A Machine Learning based SLA-Aware VNF Anomaly Detection Method in Virtual Networks
2020 International Conference on Information and Communication Technology Convergence (ICTC)(2020)
摘要
Since the concept of Software-Defined Networking (SDN) and Network Function Virtualization (NFV) has been proposed, telcos and service providers have leveraged these concepts to provide their services more efficiently. However, as the virtual network in the data centers becomes more complex, a variety of new network management problems arise. To deal with these management problems, it is necessary to monitor and analyze resource usage and traffic load of Virtual Network Functions (VNFs) operating on the virtual network. Recently, there have been many attempts to develop technologies that enable network management without human intervention. Many of these attempts based on machine learning technologies are limited to detecting typical resource usage-related anomalies with a binary classification such as CPU/memory or traffic overload. In this paper, we specify our anomaly detection problem with scenarios involving SLA violations to satisfy the practical needs of network management. We use the datasets collected from the VNFs' service function chain scenarios implemented on OpenStack environment, and compare the accuracy of the anomaly detection models generated by various machine learning algorithms. Our experimental results show the best model has F1-measure over 95% for anomaly detection.
更多查看译文
关键词
anomaly detection,network monitoring,NFV management,machine learning
AI 理解论文
溯源树
样例
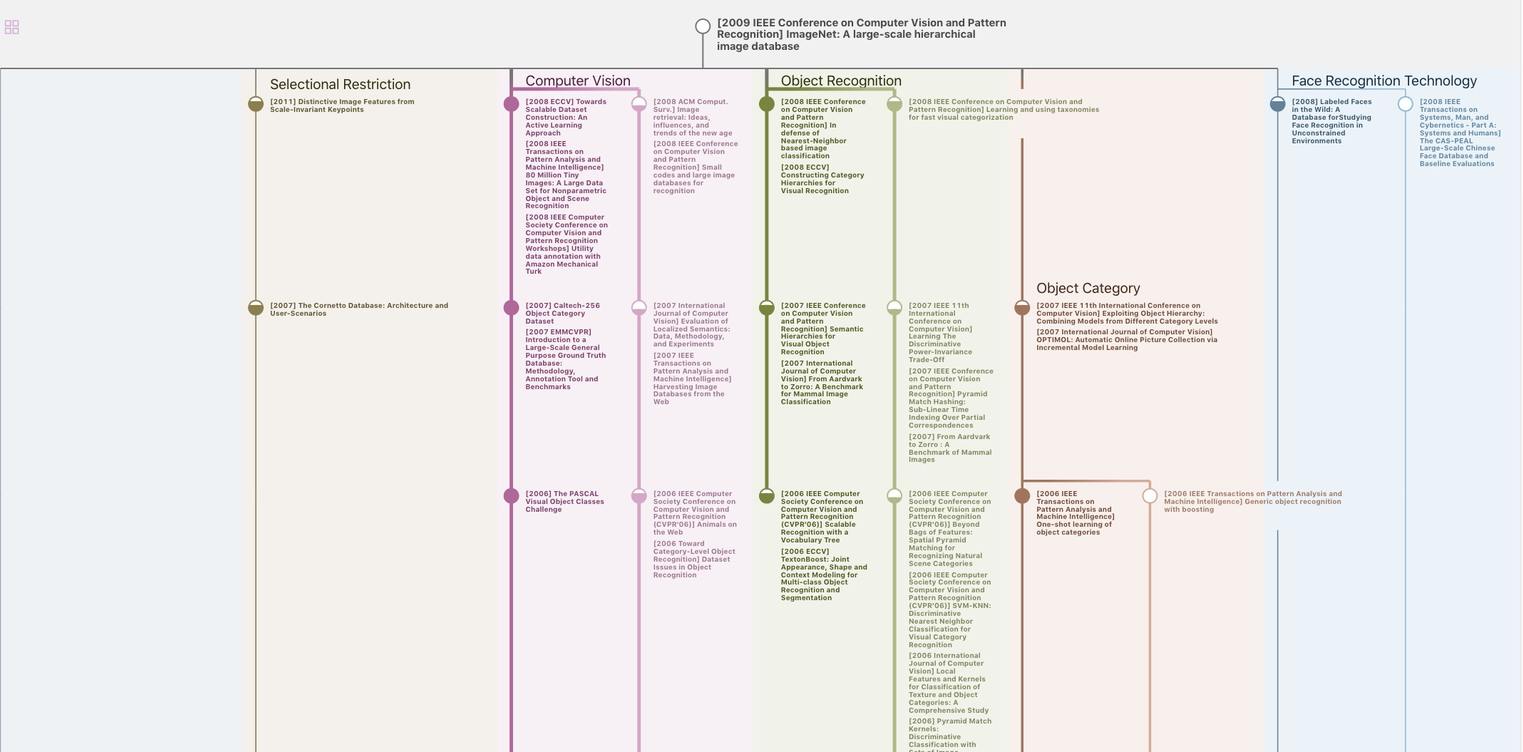
生成溯源树,研究论文发展脉络
Chat Paper
正在生成论文摘要