DeepER: A Deep Learning based Emergency Resolution Time Prediction System
2020 International Conferences on Internet of Things (iThings) and IEEE Green Computing and Communications (GreenCom) and IEEE Cyber, Physical and Social Computing (CPSCom) and IEEE Smart Data (SmartData) and IEEE Congress on Cybermatics (Cybermatics)(2020)
摘要
Accurately predicting resolution time for emergency incidents is crucial for public safety and smooth functioning of cities as it helps in planning resources that will be available for immediate assistance. In this paper, we present DeepER, a deep learning based emergency resolution time prediction system that predicts future resolution times based on past data. DeepER is an encoder-decoder based sequence-to-sequence model that uses Recurrent Neural Networks (RNNs) as the neural network architecture. The basic cell in DeepER is a Long Short-Term Memory (LSTM) cell. We perform experiments on the NYC Emergency Response Incidents data provided by NYC Open Data. We effectively preprocess the data to deal with uneven distribution of resolution times, outliers, and missing values. We compare the performance of the model with ARIMA and Linear Regression using two metrics- Root Mean Squared Error (RMSE) and Mean Absolute Error (MAE). DeepER achieves an average performance improvement of 3% and 16% with respect to RMSE and 10% and 27% with respect to MAE over ARIMA and Linear Regression, respectively.
更多查看译文
关键词
emergency resolution prediction,deep learning,forecasting
AI 理解论文
溯源树
样例
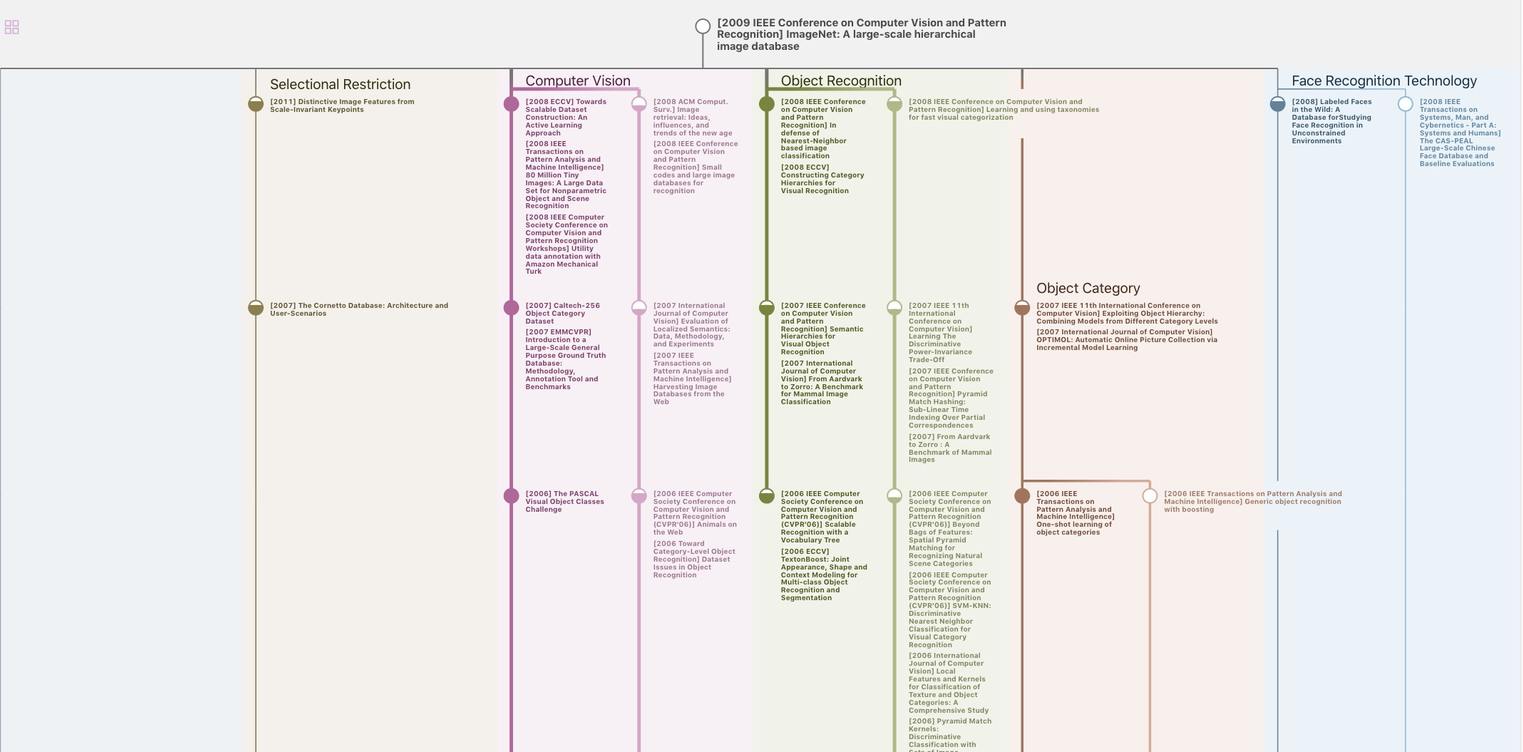
生成溯源树,研究论文发展脉络
Chat Paper
正在生成论文摘要